- 1Speech and Hearing Research Group, Department of Computer Science, University of Sheffield, Sheffield, UK
- 2Interaction Lab, School of Informatics, University of Skövde, Skövde, Sweden
Almost all animals exploit vocal signals for a range of ecologically motivated purposes: detecting predators/prey and marking territory, expressing emotions, establishing social relations, and sharing information. Whether it is a bird raising an alarm, a whale calling to potential partners, a dog responding to human commands, a parent reading a story with a child, or a business-person accessing stock prices using Siri, vocalization provides a valuable communication channel through which behavior may be coordinated and controlled, and information may be distributed and acquired. Indeed, the ubiquity of vocal interaction has led to research across an extremely diverse array of fields, from assessing animal welfare, to understanding the precursors of human language, to developing voice-based human–machine interaction. Opportunities for cross-fertilization between these fields abound; for example, using artificial cognitive agents to investigate contemporary theories of language grounding, using machine learning to analyze different habitats or adding vocal expressivity to the next generation of language-enabled autonomous social agents. However, much of the research is conducted within well-defined disciplinary boundaries, and many fundamental issues remain. This paper attempts to redress the balance by presenting a comparative review of vocal interaction within-and-between humans, animals, and artificial agents (such as robots), and it identifies a rich set of open research questions that may benefit from an interdisciplinary analysis.
1. Introduction
Almost all living organisms make (and make use of) sounds – even plants (Appel and Cocroft, 2014) – and many animals have specialized biological apparatus that is adapted to the perception and production of sound (Hopp and Evans, 1998). For example, some fish vibrate their swim bladders, many arthropods stridulate,1 and the majority of birds and mammals “vocalize” (using a vocal organ known as a syrinx or a larynx, respectively). Predators may use vocal cues to detect their prey (and vice versa), and a variety of animals (such as birds, frogs, dogs, wolves, foxes, jackals, coyotes, etc.) use vocalization to mark or defend their territory. Social animals (including human beings) also use vocalization to express emotions, to establish social relations, and to share information. Human beings, in particular, have extended this behavior to a very high level of sophistication through the evolution of speech and language – a phenomenon that appears to be unique in the animal kingdom, but which shares many characteristics with the communication systems of other animals.
Likewise, auditory perception in many animals is adapted to their acoustic environment and the vocal behavior of other animals, especially conspecifics2 (Talkington et al., 2012). Vocalization thus sits alongside other modes (such as vision and olfaction) as a primary means by which living beings are able to sense their environment, influence the world around them, coordinate cooperative or competitive behavior with other organisms, and communicate information.
Alongside the study of vocal behavior, recent years have seen important developments in a range of technologies relating to vocalization. For example, systems have been created to analyze and playback animals calls, to investigate how vocal signaling might evolve in communicative agents, and to interact with users of spoken language technology.3 Indeed, the latter has witnessed huge commercial success in the past 10–20 years, particularly since the release of Naturally Speaking (Dragon’s continuous speech dictation software for a PC) in 1997 and Siri (Apple’s voice-operated personal assistant and knowledge navigator for the iPhone) in 2011. Research interest in this area is now beginning to focus on voice-enabling autonomous social agents (such as robots).
Therefore, whether it is a bird raising an alarm, a whale calling to potential partners, a dog responding to human commands, a parent reading a story with a child, or a business-person accessing stock prices using an automated voice service on their mobile phone, vocalization provides a valuable communication channel through which behavior may be coordinated and controlled, and information may be distributed and acquired. Indeed, the ubiquity of vocal interaction has given rise to a wealth of research across an extremely diverse array of fields from the behavioral and language sciences to engineering, technology, and robotics.
Some of these fields, such as human spoken language or vocal interactivity between animals, have a long history of scientific research. Others, such as vocal interaction between artificial agents or between artificial agents and animals, are less well studied – mainly due to the relatively recent appearance of the relevant technology. This means that there is huge potential for cross-fertilization between the different disciplines involved in the study and exploitation of vocal interactivity. For example, it might be possible to use contemporary advances in machine learning to analyze animal activity in different habitats or to use artificial agents to investigate contemporary theories of language grounding. Likewise, an understanding of animal vocal behavior might inform how vocal expressivity might be integrated into the next generation of autonomous social agents.
This paper appraises our current level of understanding about vocal interactivity within-and-between humans, animals, and artificial agents (such as robots). In particular, we present a snapshot of our understanding in six key areas of vocal interaction: animal⇔animal, human⇔human, robot⇔robot, human⇔animal, human⇔robot, and animal⇔robot (see Figure 1) through the consideration of three aspects of vocal interactivity:
1. Vocal signals in interaction. This concerns properties of the signals themselves, including their structure, grammar, and semantic content where applicable. This topic contains a large body of research on both animal and human vocalizations.
2. Vocal interaction between agents. Here, we primarily discuss the functions and different types of vocally interactive behavior between animals, between human beings and animals, and between human beings and technology.
3. Technology-based research methodologies. Lastly, this paper reviews the use of technology in studying vocal interactivity. These are of interest since they provide relatively recent and novel means to further our understanding in the field while also contributing to the development of new technology capable of vocal interaction.

Figure 1. Illustration of the key types of vocal interactivity linking humans, animals, and artificial agents (such as robots).
Given the vastness of the topics covered, we aim for snapshots that provide a good sense of the current state-of-the-art and allow us to identify some of the most pertinent open research questions that might benefit from a cross-disciplinary approach. In particular, when reviewing research on specific aspects of human and/or animal vocal interactivity, we also highlight questions pertaining to the design of future vocally interactive technologies that these raise.
2. Vocal Signals in Interaction
2.1. Physiology and Morphology
A range of different neural and physical mechanisms are involved in the production, perception, and interpretation of vocal behavior in humans, animals, and artificial agents (Doupe and Kuhl, 1999; Jarvis, 2004; Ackermann et al., 2014; Andics et al., 2014). The physical apparatus for articulation and audition differs from species to species, as does the neural substrate for processing incoming signals and generating outgoing signals. In some species, it has also been hypothesized that exploiting the vocal production system in a form of analysis-by-synthesis may facilitate the understanding of vocal input (Arbib, 2005).
Human beings are mammals and, as such, the physical mechanisms for producing and perceiving vocalizations are constructed along the same lines as those possessed by all other land mammals. Air flow from the lungs excites resonances in the oral cavity (the “vocal tract”) by vibrating the vocal cords to produce a rich harmonic sound structure, by creating partial closures and using the resulting turbulence to generate noisy fricative sounds, or by closing the vocal tract completely and producing explosive sounds on releasing the air pressure. The spectral characteristics of the generated sounds are modified by the shape of the vocal tract and thus continually influenced by the movement and position of the main articulators – the tongue, the lips, and the jaw. As in other animals, body size influences the characteristics of the vocalizations that human beings are capable of producing. Hence, the pitch of the voice and the “formants” (the vocal tract resonances) are considerably higher in a small child than they are in an adult. In a recent review, Pisanski et al. (2016) suggest that the control of vocal aspects, such as height of formants and pitch, to convey body size information, could be an evolutionary step toward our ability of producing speech.
One difference between the human vocal tract and those of all other mammals is that it is bent into an “L” shape, primarily as a result of our upright vertical posture. This configuration gives rise to the so-called “descended larynx” in adult humans, and it has been hypothesized that this allows human beings to produce a much richer variety of sounds than other mammals (for example, a dog or a monkey) (Lieberman, 1984). This traditional view has been challenged (Fitch and Reby, 2001).
In general terms, however, much regarding the similarities/differences between the vocal systems (including brain organization) in different animals remain unknown and open to further research. Similarly, while morphology has an obvious influence on vocalization as just discussed, the precise nature of this influence and how vocal mechanisms are constrained (or indeed facilitated) by the morphology of the individual agents involved is a topic deserving further study.
2.2. Properties and Function of Animal Signals
Several works have been dedicated to studying how non-human animals adapt their vocalizations to the acoustic context and to the listeners’ perception. Potash (1972) showed how ambient noise modifies the intensity, rate, and type of calls of the Japanese quail. Experiments conducted by Nonaka et al. (1997) demonstrate that the brain stems of cats hold neuronal mechanisms for evoking the Lombard reflex (Lombard, 1911) of increasing speaker effort under the presence of noise. This effect has also been observed in many avian species (Cynx et al., 1998; Manabe et al., 1998; Kobayasi and Okanoya, 2003; Leonard and Horn, 2005) and in frogs (Halfwerk et al., 2016). Recent work has focused on how other aspects of the vocalizations, such as duration or frequency, are adapted and on the role of auditory feedback in such adaptations (Osmanski and Dooling, 2009; Hage et al., 2013).
Non-human animals have been shown to adapt their vocalizations depending on the audience. For instance, female Vervet monkeys produce a higher rate of alarm calls in the presence of their offspring. Likewise, male Vervet monkeys make more calls in the presence of adult females than when other dominant males are near (Cheney and Seyfarth, 1985). In some cases, animals may employ vocalizations targeted at individuals of a different species. The kleptoparasitic fork-tailed drongo, when following terrestrially foraging pied babblers, will even perform false alarm calls to make the babblers fly to cover, thereby giving the drongos an opportunity to steal food items (Ridley et al., 2007). Also, vocal communication between species is not confined to animals of the same class, e.g., hornbills (a tropical bird) are known to be capable of distinguishing between different primate alarm calls (Rainey et al., 2004).
Some alarm and mobbing calls serve as an example of the capacity of non-human animals to transmit semantic information referring to specific stimuli categories, to an associated risk, or to a particular amount of danger. Seyfarth et al. (1980) showed how vervet monkeys use and recognize different alarm calls for at least three predators: leopards, eagles, and snakes. Predator or danger-specific calls have been observed in many other species and situations (Blumstein and Armitage, 1997; Greene and Meagher, 1998; Zuberbühler, 2000, 2001; Manser, 2001; Templeton et al., 2005; Griesser, 2009; Yorzinski and Vehrencamp, 2009).
Perhaps, the most interesting recent development in the field of non-human animal vocal interaction is the evidence of syntactic and combinatory rules, grammar, and learning in certain species. For example, McCowan et al. (1999) showed (using bottlenose dolphin whistle repertoires) how an information–theoretic analysis could be used to compare the structural and organizational complexity of various animal communications systems. Ouattara et al. (2009) investigated the ability of non-human primates to generate meaningful acoustic variation during call production – a behavior that is functionally equivalent to suffixation in human language when referring to specific external events. A study by Schel et al. (2010) on the alarm call sequences of colobus monkeys concluded that the monkeys attended to the compositional aspects of utterances. Clay and Zuberbühler (2011) showed the ability of bonobos to extract information about external events by attending to vocal sequences of other individuals, thus highlighting the importance of call combinations in their natural communication system. Candiotti et al. (2012) describe how some non-human primates vary the acoustic structure of their basic call type and, through combination, create complex structures that increase the effective size of their vocal repertoire.
Zuberbühler (2002) shows that the semantic changes introduced by a combinatory rule in the natural communication of a particular species of primate may be comprehended by members of another species. Arnold and Zuberbühler (2008) conclude that in the free-ranging putty-nosed monkeys, meaning is encoded by call sequences, not individual calls. Clarke et al. (2006) provide evidence of referential signaling in a free-ranging ape species, based on a communication system that utilizes combinatorial rules. Even though most work is focused on primates, this vocal behavior is also seen in others, e.g., Kershenbaum et al. (2012) provide evidence of complex syntactic vocalizations in a small social mammal: the rock hyrax. More recently, several quantitative approaches have been proposed to understanding the complex structure of bird songs (Sasahara et al., 2012; Weiss et al., 2014) and other non-human vocalization sequences (Kershenbaum et al., 2016).
ten Cate and Okanoya (2012) review a series of studies and perceptual experiments using artificial grammars that confirm the capacity of non-human animals to generalize and categorize vocal sequences based on phonetic features. Another reviewed set of experiments show ability in non-humans to learn simple rules, such as co-occurrence or duplication of vocal units. However, the capacity of non-human animals to detect abstract rules or rules beyond finite-state grammars remains an open question.
Overall, establishing reliable communications in a challenging environment may therefore require additional effort on the part of the interlocutors, and there is good evidence that animals and human beings alter the characteristics (such as loudness, clarity, or timing) of their vocalizations as a function of the context and perceived communicative success (Brumm and Slater, 2006; Hooper et al., 2006; Candiotti et al., 2012; Hotchkin et al., 2013). Such adaptive behavior may be conditioned on the distance between the interlocutors, the ambient noise level, or the reverberant characteristics of the environment. In general, such behavior is an evidence for “negative feedback control” (Powers, 1974). What objective functions are being optimized? How are vocalizations manipulated to achieve the desired results, and is such behavior reactive or proactive? How should vocally interactive artificial agents be designed in this context?
Further, advanced vocal communication systems, such as language, seem to depend on an intimate connection between low-level sensorimotor processing and high-level cognitive processing. This appears to be necessary in order for contextual knowledge, priors, and predictions to constrain the interpretation of ambiguous and uncertain sensory inputs. “Theory of Mind” (ToM), in particular, is the mechanism by which agents can infer intentions and cognitive states underlying overt behavior. However, the degree to which non-human animals have ToM (Premack and Woodruff, 1978; Bugnyar et al., 2016) or how such insights are supported in different brains (Kirsch et al., 2008) remain unclear. As discussed further below, vocal interactivity is likely often teleological and is thus conditioned on underlying intentions. Does this imply that ToM is crucial for language-based interaction? What level of ToM do animals possess, and could this be used to predict the complexity of their vocal interactivity? Similarly, do artificial agents need ToM in order to interact effectively with human beings vocally?
Finally, although we focus vocal interactivity here, it is nonetheless worth mentioning that there are important issues arising from the relationship between vocal and non-vocal signals in various types of animal (and indeed human) modes of interaction. Indeed, vocal interaction almost always takes place in a multimodal context (Wermter et al., 2009; Liebal et al., 2013; Mavridis, 2014), and this means that vocalization may well be critically coordinated with other physical activities, such as gestures (Esposito and Esposito, 2011; Gillespie-Lynch et al., 2013; Wagner et al., 2014), gaze direction (Holler et al., 2014), and body posture (Morse et al., 2015). How are such multimodal behaviors orchestrated, especially in multi-agent situations? How is information distributed across the different modes, and what is the relationship between vocal and non-vocal (sign) language?
2.3. Properties of Human Language
2.3.1. Structure
The main difference between human and animal vocalization lies not in the physical mechanisms per se, but in how they are used. As Miyagawa et al. (2014) have pointed out, human beings still employ their vocal apparatus as an animal-like call system (primarily to communicate affect). However, humans have also evolved a remarkable system for very high-rate information transfer that appears to be vastly superior to that enjoyed by any other animals – language. Indeed, based on the work of Dawkins (1991) and Gopnik et al. (2001), it can be reasonably claimed that “Spoken language is the most sophisticated behaviour of the most complex organism in the known universe” (Moore, 2007b).
The “special” nature of human spoken language has been much discussed, and it has been hypothesized that it is distinguished from all other forms of animal communication systems through its use of “recursion,” especially in syntactic structure (Hauser et al., 2002). Although compelling, such a distinction was immediately questioned by Pinker and Jackendoff (2005). What is clear is that human language appears to be based on a “particulate” (as opposed to “blending”) mechanism for combining elements in a hierarchical structure that exploits the combinatorial properties of compound systems (Abler, 1989). This means that the expressive power of human language is effectively unlimited – as von Humboldt (1836) famously said “Language makes infinite use of finite media.” Likewise, human spoken language appears to be organized as a “contrastive” communication system, which aims to minimize communicative effort (i.e., employs minimal sound distinctions) while at the same time preserving communicative effectiveness, thereby giving rise to language-dependent “phonemic” structure.
The traditional representational hierarchy for spoken language spans acoustics, phonetics, phonology, syntax, semantics, and pragmatics. There is insufficient space here to discuss all of these levels in detail. Suffice to say that each area has been the subject of intensive study for several hundred years, and these investigations have given rise to many schools of thought regarding the structure and function of the underlying mechanisms. Of particular interest are the perspectives provided by research into the phylogenetic roots and ontogenetic constraints that condition spoken language in both the species and the individual (Stark, 1980; MacNeilage, 1998; Aitchison, 2000; Fitch, 2000, 2010).
2.3.2. Human Language Evolution and Development
The contemporary view is that language is based on the coevolution of two key traits – ostensive-inferential communication and recursive mind-reading (Scott-Phillips, 2015) – and that meaning is grounded in sensorimotor experience. For relatively concrete concepts, this is substantiated by a number of studies that show activations in sensorimotor areas of the brain during language processing [see, e.g., Chersi et al. (2010), for a discussion]. For more abstract concepts, if (and if so, how) they are grounded in sensorimotor experience is still a matter of debate [e.g., Thill et al. (2014)]. Metaphors have been put forward as one mechanism to achieve such grounding (Lakoff and Johnson, 1980; Feldman, 2008), but others argue that abstract concepts may (possibly in addition) build on linguistic or statistical information that is not directly grounded (Barsalou et al., 2008; Dove, 2011; Thill and Twomey, 2016).
There is also considerable interest in the developmental trajectory exhibited by young children while acquiring language (Gopnik et al., 2001), including long-term studies of word learning (Roy et al., 2015). It is well established that early babbling and vocal imitation serves to link perception and production, and that an adult addressing an infant will adapt their own speech to match that of the child (so-called “infant-directed speech”) (Kuhl, 2000). There is also evidence that children are sensitive to statistical and prosodic regularities allowing them to infer the structure and composition of continuous contextualized input (Saffran et al., 1996; Saffran, 2003; Kuhl, 2004; Smith and Yu, 2008).
Rather more controversial is the claim that children exhibit an acceleration in word learning around the age of 18 months – the so-called “vocabulary spurt” phenomenon (McCarthy, 1954; Goldfield and Reznick, 1990; Nazzi and Bertoncini, 2003; Ganger and Brent, 2004). However, using data from almost 1800 children, Moore and ten Bosch (2009) found that the acquisition of a receptive/productive lexicon can be quite adequately modeled as a single mathematical growth function (with an ecologically well founded and cognitively plausible interpretation) with little evidence for a vocabulary spurt.
2.3.3. Interlocutor Abilities
These perspectives on language not only place strong emphasis on the importance of top-down pragmatic constraints (Levinson, 1983) but they are also founded on an implicit assumption that interlocutors share significant priors. Indeed, evidence suggests that some animals draw on representations of their own abilities [expressed as predictive models (Friston and Kiebel, 2009)] in order to interpret the behaviors of others (Rizzolatti and Craighero, 2004; Wilson and Knoblich, 2005). For human beings, this is thought to be a key enabler for efficient recursive mind-reading (Scott-Phillips, 2015) and hence for language (Pickering and Garrod, 2007; Garrod et al., 2013).
A significant factor in the study of (spoken) language is that its complexity and sophistication tends to be masked by the apparent ease with which it is used. As a result, theories are often dominated by a somewhat naïve perspective involving the coding and decoding of messages passing from one brain (the sender) to another (the receiver). They also place a strong emphasis on “turn-taking,” and hence interaction, in spoken language dialog (Levinson, 2006, 2015). However, some researchers claim that “languaging” is better viewed as an emergent property of the dynamic coupling between cognitive unities that serves to facilitate distributed sense-making through cooperative (social) behaviors (Maturana and Varela, 1987; Bickhard, 2007; Cowley, 2011; Cummins, 2014; Fusaroli et al., 2014).
It is also important to consider the dependencies that exist between interlocutors and the effect such dependencies have on interactive behaviors. The degree to which a talker takes into account the perceived needs of the listener strongly conditions the resulting vocalizations. For example, it is well established that talkers adjust the volume and clarity of their speech in the presence of noise and interference (Lombard, 1911). This is the reason why there is a lack of so-called “invariance” in the vocal signals. Such adaptive behavior is ubiquitous, and speaker–listener coupling may be readily observed in interactions between adults and children (Fernald, 1985), between native and non-native speakers (Nguyen and Delvaux, 2015), and even between humans and machines (Moore and Morris, 1992). As observed by Lindblom (1990), such dependencies may be explained by the operation of control–feedback processes that maximize communicative effectiveness, while minimizing the energy expended in doing so.
2.3.4. Conveyance of Emotion
The formal study of emotion started with the observational work of Charles Darwin (Darwin, 1872) and has since grown into the field we know today as “Affective Science” [and its technical equivalent – “Affective Computing” (Picard, 1997)]. Emotion is a complex physiological, cognitive, and social phenomenon that is exhibited by both humans and animals. Plutchik (1980) hypothesized that emotions serve an adaptive role in helping organisms deal with key survival issues posed by the environment, and that, despite different forms of expression in different species, there are certain common elements, or prototype patterns, that can be identified. In particular, Plutchik (1980) claimed that there a small number of basic, primary, or prototype emotions – conceptualized in terms of pairs of polar opposites – and that all other emotions are mixed or derivative states. Ekman (1999) subsequently proposed six “basic emotions”: happiness, sadness, fear, anger, surprise, and disgust. More recent research favors a “dimensional” approach based on valence (positive vs. negative), arousal, and dominance [Mehrabian (1996), see also the circumplex model, Russell (1980)].
The expression of emotions can be of communicative value, and a number of theories exist regarding this value (Thill and Lowe, 2012). For example, it has been put forward that expressing emotions facilitates social harmony (Griffiths and Scarantino, 2005), while Camras (2011) suggests that emotion expression may even serve the need of the expressor in the sense that it can manipulate the perceiver to the benefit of the expressor’s needs.
In general, emotion is thought to be just one aspect of the various “affective states” that an animal or human being can exhibit, the others being personality, mood, interpersonal stances, and attitudes – all of which have the potential to influence vocalization (Scherer, 2003; Seyfarth and Cheney, 2003; Pongrácz et al., 2006; Soltis et al., 2009; Perez et al., 2012). The research challenge, especially in respect of emotionally aware artificial agents, is to identify the degree to which affective states can be interpreted and expressed, and whether they should be treated as superficial or more deeply rooted aspects of behavior. What is the role of vocal affect in coordinating cooperative or competitive behavior? How do affective states influence communicative behavior? Interesting work in this direction includes, for example, the design of sound systems that are capable of conveying internal states of a robot through appropriate modulation of the vocal signals (Schwenk and Arras, 2014).
2.4. Comparative Analysis of Human and Animal Vocalization
One of the most important overarching set of research questions relates to the special (or possibly unique) position of human language in relation to the signaling systems used by other living systems, and how we acquired it as a species (Fitch, 2000; Knight et al., 2000; MacNeilage, 2008; Tomasello, 2008; Berwick et al., 2013; Ravignani et al., 2016; Vernes, 2016). Likewise, it is often asked whether the patterning of birdsong is similar to speech or, perhaps, more related to music (Shannon, 2016). As discussed earlier, human spoken language appears to have evolved to be a contrastive particulate compositional communication system founded on ostensive–inferential recursive mind-reading. Some of these features are exhibited by non-human animals (Berwick et al., 2011; Arnold and Zuberbühler, 2012; ten Cate, 2014). In particular, Engesser et al. (2015) recently claimed evidence for “phonemic” structure in the song of a particular species of bird [although the value of this result was immediately questioned by Bowling and Fitch (2015)]. However, only humans appear to have evolved a system employing all these aspects, so there is considerable interest in comparative analyses of how communication systems can emerge in both living and artificial systems (Oller, 2004; Lyon et al., 2007; Nolfi and Mirolli, 2010). What, for example, is the relationship (if any) between language and the different signaling systems employed by non-human animals? To what degree is there a phonemic structure to animal communications, and how would one experimentally measure the complexity of vocal interactions (beyond information–theoretic analyses)? Bringing it all together, to what extent can different animals said to possess language and to what degree can human vocal interactivity be said to be signaling?
Similarly, vocal learning (especially imitation and mimicry) is thought to be a key precursor of high-order vocal communication systems, such as language (Jarvis, 2006a,b; Lipkind et al., 2013), and only a subset of species exhibits vocal learning: parrots, songbirds, humming birds, humans, bats, dolphins, whales, sea lions, and elephants (Reiss and McCowan, 1993; Tchernichovski et al., 2001; Poole et al., 2005; Pepperberg, 2010; King and Janik, 2013; Chen et al., 2016). More recently, Watson et al. (2015) have added chimpanzees to the list. However, the degree to which animals are capable of learning complex rules in vocal interaction remains an open question (ten Cate and Okanoya, 2012). What are the common features of vocal learning that these species share, and why is it restricted to only a few species? How does a young animal (such as a human child) solve the correspondence problem between the vocalizations that they hear and the sounds that they can produce? Who should adapt to whom in order to establish an effective channel [see, for example, Bohannon and Marquis (1977), for a study showing that adults adapt their vocal interactivity with children based on comprehension feedback by the children]? How are vocal referents acquired? What, precisely, are the mechanisms underlying vocal learning?
3. Vocal Interactivity
3.1. Use of Vocalization
Cooperative, competitive, and communicative behaviors are ubiquitous in the animal kingdom, and vocalization provides a means through which such activities may be coordinated and managed in communities of multiple individuals [see, e.g., work by Fang et al. (2014); King et al. (2014); Volodin et al. (2014); Ma (2015)]. Recent years have also seen an emergence of interest in “social signal processing” (Pentland, 2008; Vinciarelli et al., 2009) and even in the characteristics of speech used during speed-dating (Ranganath et al., 2013). This in itself already raises a number of interesting questions. Does the existence (or absence) of prior relationships between agents impact on subsequent vocal activity? Do the characteristics of vocalizations carry information about the social relationship connecting the interactants (for example, how is group membership or social status signaled vocally)? This goes beyond conspecifics – humans and dogs are able to manage a productive and mutually supportive relationship despite the vocal communication being somewhat one-sided. What is it about the human–dog relationship that makes this one-sidedness sufficient, and conversely, what can biases in communication balancing say about social relationships? Finally, how is vocalization used to sustain long-term social relations?
Non-human animals make multiple uses of vocalizations – from signals warning of the presence of predators to social calls strengthening social bonding between individuals. Alarm vocalizations are characterized by being high pitched to avoid the predator localizing the caller (Greene and Meagher, 1998). Alarm calls have been extensively studied in a wide variety of species (Seyfarth et al., 1980; Cheney and Seyfarth, 1985; Blumstein, 1999; Manser, 2001; Fichtel and van Schaik, 2006; Arnold and Zuberbühler, 2008; Stephan and Zuberbühler, 2008; Schel et al., 2010).
The function of alarm calls is not limited to warning conspecifics. For example, Zuberbühler et al. (1999) observed that high rates of monkey alarm calls had an effect on the predator who gave up his hiding location faster once it was detected. Many other animals employ vocalizations to cooperatively attack or harass a predator; these are known as mobbing calls (Ficken and Popp, 1996; Hurd, 1996; Templeton and Greene, 2007; Clara et al., 2008; Griesser, 2009; Yorzinski and Vehrencamp, 2009).
Another role of vocal communication between animals is to inform individuals during the selection of mating partners. Mating or advertising calls have received much research attention in birds due to their complex vocalizations (McGregor, 1992; Searcy and Yasukawa, 1996; Vallet et al., 1998; Gil and Gahr, 2002; Mennill et al., 2003; Pfaff et al., 2007; Alonso Lopes et al., 2010; Bolund et al., 2012; Hall et al., 2013). However, many other species employ such vocal interaction during sexual selection (Brzoska, 1982; Gridi-Papp et al., 2006; Charlton et al., 2012).
Some species use vocalizations to advertise their territory or to maintain territorial exclusion, and the sounds emitted will usually travel long distances. For example, wolves use howls as means to control wolf pack spacing (Harrington and Mech, 1983). These types of vocalization are also used by frogs to advertise their willingness to defend their territory (Brzoska, 1982). Territorial calls also play an important role in sea lions during the breeding season (Peterson and Bartholomew, 1969; Schusterman, 1977). Sea lions and other pinnipeds are also commonly cited as animals that use vocalization between mothers and their offspring. Mothers employ a “pup-attraction call” that will often elicitate a “mother-response call” in the pup (Trillmich, 1981; Hanggi and Schusterman, 1990; Gisiner and Schusterman, 1991; Insley, 2001). Mother–offspring calls are one of many examples of the transmission of identity information through animal vocalizations. This aspect has also been studied in the context of songbirds (Weary and Krebs, 1992; Lind et al., 1996), domestic horses (Proops et al., 2009), dolphins (Kershenbaum et al., 2013), and primates (Candiotti et al., 2013).
Overall, vocal signals are therefore arguably generated on purpose (Tomasello et al., 2005; Townsend et al., 2016) and serve to attract attention (Crockford et al., 2014) as well as to provide information (Schel et al., 2013) and support cooperation (Eskelinen et al., 2016). However, other agents can exploit unintentional vocalizations for their own purposes. Also, in living systems, the ultimate driver of behavior is thought to be a hierarchy of “needs” (with survival as the most basic) (Maslow, 1943). As a result, there is interest in the role of “intrinsic motivations,” especially learning (Moulin-Frier et al., 2013). To what extent are vocal signals teleological, and is it possible to distinguish between intentional and unintentional vocalizations? Can intentional vocal activity be simulated by technological means to explore animal behavior? Does a vocalization carry information about the underlying intention, and how can the latter be inferred from the former? How do motivational factors such as “urgency” impact on vocalization? What motivational framework would be appropriate for a voice-enabled autonomous social agent?
An interesting type of vocal interaction, which often occurs between mating pairs is duetting. This comprises a highly synchronized and temporally precise vocal display involving two individuals. Duets have been observed in several bird species (Grafe et al., 2004; Hall, 2004; Elie et al., 2010; Templeton et al., 2013; Dowling and Webster, 2016). There are a number of different hypotheses concerning the function of such behavior, e.g., territory defense, mate-guarding, and paternity-guarding (Mennill, 2006; Dowling and Webster, 2016). Duets also occur in other species and contexts, such as in the alarm calls of lemurs (Fichtel and van Schaik, 2006) and gibbons (Clarke et al., 2006). More generally, vocalizations are often carefully timed in relation to other events taking place in an environment (including other vocalizations) (Benichov et al., 2016). This may take the form of synchronized ritualistic behavior (such as rhythmic chanting, chorusing, or singing) or asynchronous turn-taking (which can be seen as a form of dialog) (Cummins, 2014; Fusaroli et al., 2014; Ravignani et al., 2014).
Of particular interest is the dynamics of such interactions in both humans and animals (Fitch, 2013; Takahashi et al., 2013; De Looze et al., 2014), especially between conspecifics (Friston and Frith, 2015). Is there a common physiological basis for such rhythmic vocal behavior, and how is vocal synchrony achieved between agents? What are the segmental and suprasegmental prosodic features that facilitate such timing relations? What are the dependencies between vocalizations and other events, and how would one characterize them? Given the crucial nature of synchrony and timing in interactivity between natural agents, to what extent does this importance carry over to human–machine dialog? How would one model the relevant dynamics (whether to study natural interactivity or to facilitate human–machine interaction)?
3.2. Vocal Interactivity between Non-Conspecifics
Vocal interaction normally takes place between conspecifics (that is, agents with similar capabilities), but what happens between mismatched entities – between humans and/or animals and/or artificial agents? For example, Joslin (1967) employed both human-simulated howls and playback recordings to study wolf behavior and, surprisingly, discovered that the wolves responded more to the human-simulated howls than to the playbacks. Also Kuhl (1981) conducted listening tests on chinchillas in order to determine their capacity for discriminating speech and to provide support for the existence of a relation between the mammalian auditory system and the evolution of different languages.
More recently, the study of domestic or domesticated animals has become a topic of interest in the field of human⇔animal vocal interaction. For example, Waiblinger et al. (2006) proposes considering vocal interaction in the assessment of human–animal relationships, especially in the context of farm animals’ welfare. Also, Kaminski et al. (2004) present a case study in which they demonstrate a dog’s capacity to “fast map,” i.e., forming quick and rough semantic hypotheses of a new word after a single presentation. Horowitz and Hecht (2016) investigated owner’s vocalizations in dog–human “play” sessions and found some identifiable characteristics associated with affect.
Research in this area also extends to wild animals. For example, McComb et al. (2014) show how elephants respond differently to playbacks of human speech depending on their gender and age – aspects that can greatly affect the predator risks that humans present to elephants.
Research on vocal interactivity between non-conspecifics is particularly pertinent to the design of vocally interactive artificial agents. For example, Jones et al. (2008) found differences in individual preferences when people interacted with dog-like robots. According to Moore (2015, 2016b), understanding this situation could be critical to the success of future speech-based interaction with “intelligent” artificial agents. For example, different bodies may lead to different sensorimotor experiences in which an agent’s concepts are grounded, which may impact the degree to which two agents can communicate about the same things (Thill et al., 2014).
What, therefore, are the limitations (if any) of vocal interaction between non-conspecifics? What can be learned from attempts to teach animals, the human language (and vice versa)? How do conspecifics accommodate mismatches in temporal histories (for example, interaction between different aged agents) or cultural experience? How can insights from such questions inform the design of vocally interactive artificial agents beyond Siri? Is it possible to detect differences in how different agents ground concepts from their language use, and can artificial agents use such information in vocal interactivity with humans [as suggested by Thill et al. (2014)]?
3.3. Spoken Language Systems
On the technology front, recent years have seen significant advances in technologies that are capable of engaging in voice-based interaction with a human user. The performance of automatic speech recognition, text-to-speech synthesis, and dialog management has improved year-on-year, and this has led to a growth in the sophistication of the applications that are able to be supported, from the earliest military Command and Control Systems to contemporary commercial Interactive Voice Response (IVR) Systems and the latest Voice-Enabled Personal Assistants (such as Siri) – see Figure 2. Progress has been driven by the emergence of a data-driven probabilistic modeling paradigm in the 1980s (Gales and Young, 2007; Bellegarda and Monz, 2015) – recently supplemented by deep learning (Hinton et al., 2012) – coupled with an ongoing regime of government-sponsored benchmarking.4 Pieraccini (2012) presents a comprehensive review of the history of spoken language technology up to the release of Siri in 2011.

Figure 2. The evolution of spoken language technology from early military “Command and Control Systems” through current “Voice-Enabled Personal Assistants” (such as Siri) to future “Autonomous Social Agents” (e.g., robots).
At the present time, research into spoken language technology is beginning to focus on the development of voice-based interaction with Embodied Conversational Agents (ECAs) and Autonomous Social Agents (such as robots). In these futuristic scenarios, it is envisioned that spoken language will provide a “natural” conversational interface between human beings and the so-called intelligent systems. However, many challenges need to be addressed in order to meet such a requirement (Baker et al., 2009a; Moore, 2013, 2015), not least how to evolve the complexity of voice-based interfaces from simple structured dialogs to more flexible conversational designs without confusing the user (Bernsen et al., 1998; McTear, 2004; Lopez Cozar Delgado and Araki, 2005; Phillips and Philips, 2006; Moore, 2016b). In particular, seminal work by Nass and Brave (2005) showed how attention needs to be paid to users’ expectations [e.g., selecting the “gender” of a system’s voice (Crowell et al., 2009)], and this has inspired work on “empathic” vocal robots (Breazeal, 2003; Fellous and Arbib, 2005; Haring et al., 2011; Eyssel et al., 2012; Lim and Okuno, 2014; Crumpton and Bethel, 2016). On the other hand, user interface experts, such as Balentine (2007), have argued that such agents should be clearly machines rather than emulations of human beings, particularly to avoid the “uncanny valley effect” (Mori, 1970), whereby mismatched perceptual cues can lead to feelings of repulsion (Moore, 2012). For a voice-enabled robot, this underpins the importance of matching the voice and face (Mitchell et al., 2011).
It has also been argued that the architecture of future spoken language systems needs to be more cognitively motivated if it is to engage meaningfully with human users (Moore, 2007a, 2010; Baker et al., 2009b), or that such systems should take inspiration from the way in which children acquire their communicative skills (ten Bosch et al., 2009).
4. Technology-Based Research Methods
The large number of disciplines concerned with vocal interactivity means that there is an equally wide variety of tools, techniques, and methodologies used in the different areas of research that are relatively novel and emergent, resulting in several avenues for further research, both concerning the development of these methodologies themselves and their use in future studies of vocal interactivity. For example, large-scale data collection is the norm in spoken language technology (Pieraccini, 2012), and several international agencies exist for sharing data between laboratories (for example, the Linguistic Data Consortium5 and the European Language Resource Association).6 Are there other opportunities for sharing data or for inserting technology into non-technological areas? Is it necessary to create new standards in order to facilitate more efficient sharing of research resources?
Likewise, technology for simulating vocalizations is already being used in studies of animal behavior, but different disciplines model vocal interactivity using different paradigms depending on whether they are interested in predicting the outcome of field experiments, eliciting (Benichov et al., 2016) and simulating (Webb, 1995) the behavior in the laboratory, or engineering practical solutions (Moore, 2016a). Vocal interaction may be modeled within a variety of frameworks ranging from traditional behaviorist stimulus–response approaches (for example, using stochastic modeling or deep learning and artificial neural networks) to coupled dynamical systems (using mutual feedback control). In the latter case, vocal interaction is seen as an emergent phenomenon arising from a situated and embodied enactive relationship between cognitive unities, but how can these interactive behaviors be modeled computationally? Are there any mathematical modeling principles that may be applied to all forms of vocal interactivity, and is it possible to derive a common architecture or framework for describing vocal interactivity?
In addition, technological tools offer great potential for studying vocalization in the wild. As Webb (2008) argues, because robots that act in the world, including interacting with other agents, need to solve many of the same problems that natural autonomous agents need to solve, they provide an additional means by which to study natural behaviors of interest. An oft-cited example is that of cricket mating calls: a female will be attracted to the male of her own species who produces the loudest calls. Webb (1995) built a robot capable of reproducing this behavior using a mechanism of phase cancelation and latency comparison. This is noteworthy in that the potentially complex computational problem, of not just locating sounds but also identifying the loudest source and ensuring it is the correct species, can be solved without an explicit representation of any of these factors. This is discussed further by Wilson and Golonka (2013) as an example of embodied cognition: it is the particular morphology of the cricket’s ear channels and interneurons together with particular aspects of the environment (that males of different species will chirp at different frequencies) that solve this problem, foregoing the need for potentially complicated computations.
Robots can also help to elucidate necessary precursors and mechanisms for vocal interaction. For example, computational models have been used to investigate how children are able to solve the “correspondence problem” and map between their own perceptual and vocal experiences to those of the adult speakers with whom they interact (Howard and Messum, 2014; Messum and Howard, 2015). Even physical (robotic) models of a child’s vocal tract have been designed to understand how these early stages of spoken language acquisition might function (Yoshikawa et al., 2003; Ishihara et al., 2009; Miura et al., 2012).
Another prominent example of this line of research is the “symbol grounding problem” (Harnad, 1990), which, in brief, states that amodal symbols manipulated by a formal system, such as a computer program, have no meaning that is intrinsic to the system itself; whatever meaning may exist is instead attributed by an external observer. Some researchers [e.g., Stramandinoli et al. (2012)] argue that robots require such an intrinsic understanding of concepts to achieve natural vocal interaction with humans. Cangelosi (2006), in particular, distinguishes between physical and social symbol grounding: the former concerns the grounding of an individual’s internal representations in sensorimotor experience, while the latter refers to the determination of symbols to be shared between individuals, including their grounded meanings (in other words, social symbol grounding is the creation of a shared vocabulary of grounded symbols).
Both forms of symbol grounding are a problem that natural agents solve to a greater or lesser extent. Both forms have also been investigated in robots. Luc Steels’ language games, for instance, provide a seminal example of robotic investigations into social symbol grounding (Steels, 2001). These games investigated how artificial agents would “generate and self-organise a shared lexicon as well as the perceptually grounded categorisations of the world expressed by this lexicon, all without human intervention or prior specification” (Steels, 2003, p. 310).
Physical symbol grounding, as mentioned, is the problem of grounding an individual’s internal representations in sensorimotor experience. Implementations of these mechanisms are thus not always concerned with cognitive plausibility but rather with implementing a practical solution [see Coradeschi et al. (2013) for a recent review and Thill et al. (2014) for a longer discussion of how the simpler sensorimotor aspects considered in most robotics may affect the degree to which these can comment on human grounding]. Nonetheless, robots have, for example, been used to put forward theories of how abstract concepts can be grounded in a sensorimotor experience (Cangelosi and Riga, 2006). Stramandinoli et al. (2012), in particular, propose a hierarchical structure for concepts; some may be directly grounded in sensorimotor experience, whereas others are indirectly grounded via other concepts.
The previously mentioned review by Coradeschi et al. (2013) also follow Belpaeme and Cowley (2007) in highlighting that social symbol grounding has the necessary mechanisms for the acquisition of language and meaning as a prerequisite. Here, we want to reaffirm the overall implication; to use robots to study vocal interactivity requires the implementation of prerequisite mechanisms. It is, for instance, occasionally argued that a “mirror neuron system” is an evolutionary precursor to language abilities (Arbib, 2005). This opens the discussion to robot (and computational) models of mirror neuron systems, for which we refer to recent reviews (Oztop et al., 2006; Thill et al., 2013). It also follows from at least some theoretical positions on embodiment that the precise body of an agent may play a fundamental role in all matters of cognition, including symbol grounding (Thill and Twomey, 2016). Indeed, Thill et al. (2014) propose that robot implementations need to take this into account explicitly, suggesting that human usage of concepts, as characterized by appropriate analyses of human-produced texts may in fact yield insights into the underlying grounding. A robot, whose own body would ground these concepts in (possibly subtly) different ways, could make use of this information in interaction with human beings. Overall, then, the take-home message is that using robots in vocal interaction requires the researcher to be explicit about all aspects of the necessary model [see Morse et al. (2011), for a similar point].
Once an artificial agent that is capable of vocal interactivity has been created (whether it achieved this as a result of cognitively plausible modeling or not), it is interesting to ask how humans might actually interact with it. Branigan et al. (2011), for example, report on five experiments in which humans interacted either with other humans or (so they were told) a computer. The core behavior of interest was verbal alignment (in which participants, in a dialog, converge on certain linguistic behaviors). Their main insight was that such alignment appeared to depend on beliefs that humans held about their interlocutors (specifically, their communicative capacity); they were, for example, more likely to align on a disfavored term for an object if they believed the interlocutor was a computer. Vollmer et al. (2013) extended this work replacing the computer system with a humanoid robot (an iCub) and found a similar alignment in the domain of manual actions (rather than the lexical domain). Kopp (2010) investigated the establishment of social resonance through embodied coordination involving expressive behavior during conversation between two agents. Such aspects form an important part of human conversation and may determine whether or not they perceive the other agent as social. Kopp (2010) argued that including such mechanisms (e.g., mimicry, alignment, and synchrony) may be a significant factor in improving human–agent interaction. Recently, de Greeff and Belpaeme (2015) have demonstrated the relevance of such factors in robot learning, finding that a robot that uses appropriate social cues tends to learn faster.
Similarly, the properties of the vocal signals themselves have consequences for the overall interaction. For example, Niculescu et al. (2011) investigated the effects of voice pitch on how robots are perceived, finding that a high-pitched “exuberant” voice lead to a more positive perception of the overall interaction than a low-pitched “calm” voice, highlighting the importance of appropriate voice design for the overall quality of a human–robot interaction. Walters et al. (2008), similarly, found that the voice of the robot modulates physical approach behavior of humans to robots, and what distance is perceived as comfortable.
Finally, it is worth highlighting that such communicative systems need not always be inspired by insights from human or animal vocalization; for example, Schwenk and Arras (2014) present a flexible vocal synthesis system for HRI capable of modulating the sounds a robot makes based on both features of the ongoing interaction and internal states of the robot.
5. Conclusion
This paper satisfies two objectives. First, we have presented an appraisal of the state-of-the-art in research on vocal interactivity in-and-between humans, animals, and artificial agents (such as robots). Second, we have identified a set of open research questions, summarized again in Tables 1–3 for convenience. It is worth highlighting that many of these open research questions require an interdisciplinary approach – be it the use of artificial agents to study particular aspects of human or animal vocalization, the study of animal vocal behavior to better distinguish between signaling and language in human beings, or indeed the study of human and/or animal vocal interactivity (including between humans and animals) with a view to designing the next generation of vocally interactive technologies.

Table 1. Summary of research questions identified in this paper that pertain to vocal signals in interaction, grouped by the sections of the paper in which they are discussed.
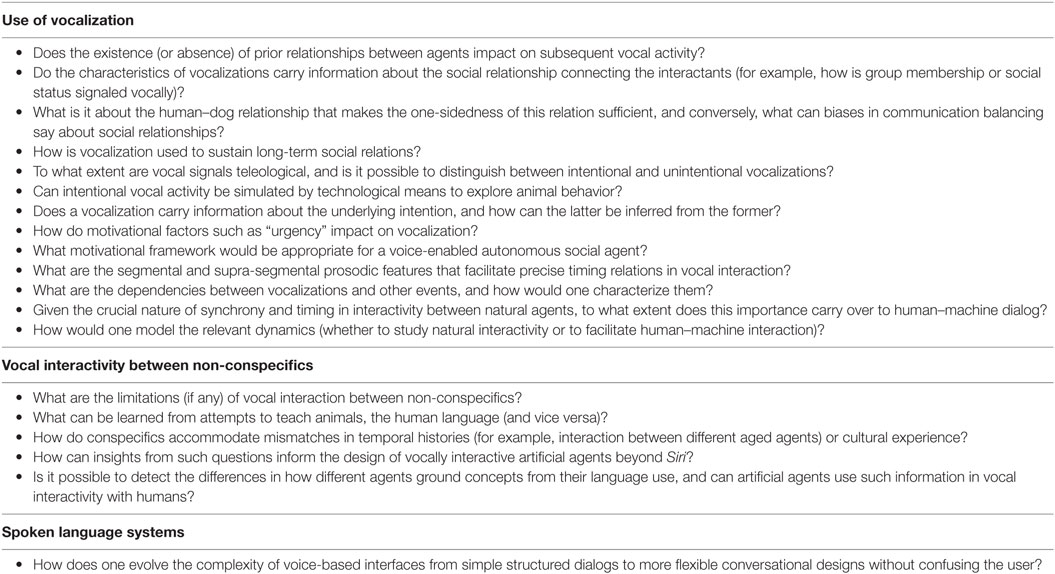
Table 2. Summary of research questions identified in this paper that pertain to vocal interactivity, grouped by the sections of the paper in which they are discussed.

Table 3. Summary of research questions identified in this paper that pertain to technology-based research methods.
The questions we have raised thus serve a dual purpose. Not only do they highlight opportunities for future research aimed at increasing our understanding of the general principles of vocal interactivity per se but they also have the potential to impact on practical applications and the design of new technological solutions. Consider, to give but one example, how current technology is moving toward an increasing number of artifacts that offer both cognitive capabilities and voice-enabled interfaces. How the vocal interactivity of such artifacts should be designed is not obvious, since it is not clear how users might expect to interact with such interfaces. Would they prefer natural language or a more command–style interface? What are the precise underlying mechanisms needed for the artifact to offer the desired capabilities?
Finally, let us close by emphasizing again that addressing many of the questions we raise fully requires an interdisciplinary approach that cuts across the different fields that study different types of vocal interactivity in different types of agent. We believe that the time is now ripe to tackle these challenges, and we expect interdisciplinary efforts at the intersections of the fields that together make up the study of vocal interactivity (as outlined in Figure 1) to blossom in the coming years.
Author Contributions
RKM, RM, and ST contributed more or less equally to the preparation of this manuscript.
Conflict of Interest Statement
The authors declare that the research was conducted in the absence of any commercial or financial relationships that could be construed as a potential conflict of interest.
Funding
This work was supported by the European Commission [grant numbers EU-FP6-507422, EU-FP6-034434, EU-FP7-231868, and EU-FP7-611971] and the UK Engineering and Physical Sciences Research Council [grant number EP/I013512/1].
Footnotes
- ^Stridulation is the act of making sound by rubbing body parts together.
- ^A conspecific is a member of the same species.
- ^Spoken language technology (SLT) includes voice-based human–computer interaction using components, such as automatic speech recognition, text-to-speech synthesis, and dialogue management.
- ^A graph illustrating the history of automatic speech recognition evaluations at the US National Information Technology Laboratory (NIST) can be found at http://www.itl.nist.gov/iad/mig/publications/ASRhistory/.
- ^https://www.ldc.upenn.edu.
- ^http://www.elra.info/en/.
References
Abler, W. L. (1989). On the particulate principle of self-diversifying systems. J. Soc. Biol. Struct. 12, 1–13. doi:10.1016/0140-1750(89)90015-8
Ackermann, H., Hage, S. R., and Ziegler, W. (2014). Brain mechanisms of acoustic communication in humans and nonhuman primates: an evolutionary perspective. Behav. Brain Sci. 37, 529–546. doi:10.1017/S0140525X13003099
Aitchison, J. (2000). The Seeds of Speech: Language Origin and Evolution. Cambridge: Cambridge University Press.
Alonso Lopes, J. C., Magaña, M., Palacín, C., and Martín, C. A. (2010). Correlates of male mating success in great bustard leks: the effects of age, weight, and display effort. Behav. Ecol. Sociobiol. 64, 1589–1600. doi:10.1007/s00265-010-0972-6
Andics, A., Gácsi, M., Faragó, T., Kis, A., and Miklósi, A. (2014). Voice-sensitive regions in the dog and human brain are revealed by comparative fMRI. Curr. Biol. 24, 574–578. doi:10.1016/j.cub.2014.01.058
Appel, H. M., and Cocroft, R. B. (2014). Plants respond to leaf vibrations caused by insect herbivore chewing. Oecologia 175, 1257–1266. doi:10.1007/s00442-014-2995-6
Arbib, M. A. (2005). From monkey-like action recognition to human language: an evolutionary framework for neurolinguistics. Behav. Brain Sci. 28, 105–124. doi:10.1017/S0140525X05000038
Arnold, K., and Zuberbühler, K. (2008). Meaningful call combinations in a non-human primate. Curr. Biol. 18, R202–R203. doi:10.1016/j.cub.2008.01.040
Arnold, K., and Zuberbühler, K. (2012). Call combinations in monkeys: compositional or idiomatic expressions? Brain Lang. 120, 303–309. doi:10.1016/j.bandl.2011.10.001
Baker, J. M., Deng, L., Glass, J., Khudanpur, S., Lee, C.-H., Morgan, N., et al. (2009a). Research developments and directions in speech recognition and understanding, part 1. IEEE Signal Process. Mag. 26, 75–80. doi:10.1109/MSP.2009.932166
Baker, J. M., Deng, L., Khudanpur, S., Lee, C.-H., Glass, J. R., Morgan, N., et al. (2009b). Updated MINDS report on speech recognition and understanding, part 2. IEEE Signal Process. Mag. 26, 78–85. doi:10.1109/MSP.2009.932707
Balentine, B. (2007). It’s Better to Be a Good Machine Than a Bad Person: Speech Recognition and Other Exotic User Interfaces at the Twilight of the Jetsonian Age. Annapolis: ICMI Press.
Barsalou, L., Santos, A., Simmons, W., and Wilson, C. (2008). “Language and simulation in conceptual processing,” in Symbols, Embodiment, and Meaning, eds M. De Vega, A. Glenberg, and A. Graesser (Oxford: Oxford University Press), 245–283.
Bellegarda, J. R., and Monz, C. (2015). State of the art in statistical methods for language and speech processing. Comput. Speech Lang. 35, 163–184. doi:10.1016/j.csl.2015.07.001
Belpaeme, T., and Cowley, S. J. (2007). Foreword: extending symbol grounding. Interact. Stud. 8, 1–6. doi:10.1075/is.8.1.02bel
Benichov, J. I., Benezra, S. E., Vallentin, D., Globerson, E., Long, M. A., and Tchernichovski, O. (2016). The forebrain song system mediates predictive call timing in female and male zebra finches. Curr. Biol. 26, 309–318. doi:10.1016/j.cub.2015.12.037
Bernsen, N. O., Dybkjaer, H., and Dybkjaer, L. (1998). Designing Interactive Speech Systems: From First Ideas to User Testing. London: Springer.
Berwick, R. C., Friederici, A. D., Chomsky, N., and Bolhuis, J. J. (2013). Evolution, brain, and the nature of language. Trends Cogn. Sci. 17, 89–98. doi:10.1016/j.tics.2012.12.002
Berwick, R. C., Okanoya, K., Beckers, G. J. L., and Bolhuis, J. J. (2011). Songs to syntax: the linguistics of birdsong. Trends Cogn. Sci. 15, 113–121. doi:10.1016/j.tics.2011.01.002
Bickhard, M. H. (2007). Language as an interaction system. New Ideas Psychol. 25, 171–187. doi:10.1016/j.newideapsych.2007.02.006
Blumstein, D. T. (1999). Alarm calling in three species of marmots. Behaviour 136, 731–757. doi:10.1163/156853999501540
Blumstein, D. T., and Armitage, K. (1997). Alarm calling in yellow-bellied marmots: I. The meaning of situationally variable alarm calls. Anim. Behav. 53, 143–171. doi:10.1006/anbe.1996.0285
Bohannon, J. N., and Marquis, A. L. (1977). Children’s control of adult speech. Child Dev. 48, 1002–1008. doi:10.2307/1128352
Bolund, E., Schielzeth, H., and Forstmeier, W. (2012). Singing activity stimulates partner reproductive investment rather than increasing paternity success in zebra finches. Behav. Ecol. Sociobiol. 66, 975–984. doi:10.1007/s00265-012-1346-z
Bowling, D. L., and Fitch, W. T. (2015). Do animal communication systems have phonemes? Trends Cogn. Sci. 19, 555–557. doi:10.1016/j.tics.2015.08.011
Branigan, H. P., Pickering, M. J., Pearson, J., McLean, J. F., and Brown, A. (2011). The role of beliefs in lexical alignment: evidence from dialogs with humans and computers. Cognition 121, 41–57. doi:10.1016/j.cognition.2011.05.011
Breazeal, C. (2003). Emotion and sociable humanoid robots. Int. J. Hum. Comput. Stud. 59, 119–155. doi:10.1016/S1071-5819(03)00018-1
Brumm, H., and Slater, P. J. (2006). Animals can vary signal amplitude with receiver distance: evidence from zebra finch song. Anim. Behav. 72, 699–705. doi:10.1016/j.anbehav.2006.01.020
Brzoska, J. (1982). Vocal response of male European water frogs (Rana Esculenta complex) to mating and territorial calls. Behav. Processes 7, 37–47. doi:10.1016/0376-6357(82)90051-1
Bugnyar, T., Reber, S. A., and Buckner, C. (2016). Ravens attribute visual access to unseen competitors. Nat. Commun. 7, 10506. doi:10.1038/ncomms10506
Camras, L. A. (2011). Differentiation, dynamical integration and functional emotional development. Emot. Rev. 3, 138–146. doi:10.1177/1754073910387944
Candiotti, A., Zuberbühler, K., and Lemasson, A. (2012). Context-related call combinations in female Diana monkeys. Anim. Cogn. 15, 327–339. doi:10.1007/s10071-011-0456-8
Candiotti, A., Zuberbühler, K., and Lemasson, A. (2013). Voice discrimination in four primates. Behav. Processes 99, 67–72. doi:10.1016/j.beproc.2013.06.010
Cangelosi, A. (2006). The grounding and sharing of symbols. Pragmat. Cogn. 14, 275–285. doi:10.1075/pc.14.2.08can
Cangelosi, A., and Riga, T. (2006). An embodied model for sensorimotor grounding and grounding transfer: experiments with epigenetic robots. Cogn. Sci. 30, 673–689. doi:10.1207/s15516709cog0000_72
Charlton, B. D., Ellis, W. A. H., Brumm, J., Nilsson, K., and Fitch, W. T. (2012). Female koalas prefer bellows in which lower formants indicate larger males. Anim. Behav. 84, 1565–1571. doi:10.1016/j.anbehav.2012.09.034
Chen, Y., Matheson, L. E., and Sakata, J. T. (2016). Mechanisms underlying the social enhancement of vocal learning in songbirds. Proc. Natl. Acad. Sci. U.S.A. 113, 6641–6646. doi:10.1073/pnas.1522306113
Cheney, D. L., and Seyfarth, R. M. (1985). Vervet monkey alarm calls: manipulation through shared information? Behaviour 94, 150–166. doi:10.1163/156853985X00316
Chersi, F., Thill, S., Ziemke, T., and Borghi, A. M. (2010). Sentence processing: linking language to motor chains. Front. Neurorobot. 4:4. doi:10.3389/fnbot.2010.00004
Clara, E., Tommasi, L., and Rogers, L. J. (2008). Social mobbing calls in common marmosets (Callithrix jacchus): effects of experience and associated cortisol levels. Anim. Cogn. 11, 349–358. doi:10.1007/s10071-007-0125-0
Clarke, E., Reichard, U. H., and Zuberbühler, K. (2006). The syntax and meaning of wild gibbon songs. PLoS ONE 1:e73. doi:10.1371/journal.pone.0000073
Clay, Z., and Zuberbühler, K. (2011). Bonobos extract meaning from call sequences. PLoS ONE 6:e18786. doi:10.1371/journal.pone.0018786
Coradeschi, S., Loutfi, A., and Wrede, B. (2013). A short review of symbol grounding in robotic and intelligent systems. Künstliche Intelligenz 27, 129–136. doi:10.1007/s13218-013-0247-2
Crockford, C., Wittig, R. M., and Zuberbühler, K. (2014). An intentional vocalization draws others’ attention: a playback experiment with wild chimpanzees. Anim. Cogn. 18, 581–591. doi:10.1007/s10071-014-0827-z
Crowell, C. R., Scheutz, M., Schermerhorn, P., and Villano, M. (2009). “Gendered voice and robot entities: perceptions and reactions of male and female subjects,” in Proceedings of the 2009 IEEE/RSJ International Conference on Intelligent Robots and Systems (IROS’09) (Piscataway, NJ: IEEE), 3735–3741.
Crumpton, J., and Bethel, C. L. (2016). A survey of using vocal prosody to convey emotion in robot speech. Int. J. Soc. Robot. 8, 271–285. doi:10.1007/s12369-015-0329-4
Cummins, F. (2014). Voice, (inter-)subjectivity, and real time recurrent interaction. Front. Psychol. 5:760. doi:10.3389/fpsyg.2014.00760
Cynx, J., Lewis, R., Tavel, B., and Tse, H. (1998). Amplitude regulation of vocalizations in noise by a songbird, Taeniopygia guttata. Anim. Behav. 56, 107–113. doi:10.1006/anbe.1998.0746
de Greeff, J., and Belpaeme, T. (2015). Why robots should be social: enhancing machine learning through social human-robot interaction. PLoS ONE 10:e0138061. doi:10.1371/journal.pone.0138061
De Looze, C., Scherer, S., Vaughan, B., and Campbell, N. (2014). Investigating automatic measurements of prosodic accommodation and its dynamics in social interaction. Speech Commun. 58, 11–34. doi:10.1016/j.specom.2013.10.002
Doupe, A. J., and Kuhl, P. K. (1999). Birdsong and human speech: common themes and mechanisms. Annu. Rev. Neurosci. 22, 567–631. doi:10.1146/annurev.neuro.22.1.567
Dove, G. (2011). On the need for embodied and dis-embodied cognition. Front. Psychol. 1:242. doi:10.3389/fpsyg.2010.00242
Dowling, J., and Webster, M. S. (2016). An experimental test of duet function in a fairy-wren (Malurus) with moderate cuckoldry rates. Behav. Ecol. 27, 228–236. doi:10.1093/beheco/arv144
Ekman, P. (1999). “Basic emotions,” in Handbook of Cognition and Emotion, eds T. Dalgleish and M. Power (New York: John Wiley), 301–320.
Elie, J. E., Mariette, M. M., Soula, H. A., Griffith, S. C., Mathevon, N., and Vignal, C. (2010). Vocal communication at the nest between mates in wild zebra finches: a private vocal duet? Anim. Behav. 80, 597–605. doi:10.1016/j.anbehav.2010.06.003
Engesser, S., Crane, J. M. S., Savage, J. L., Russell, A. F., and Townsend, S. W. (2015). Experimental evidence for phonemic contrasts in a nonhuman vocal system. PLoS Biol. 13:e1002171. doi:10.1371/journal.pbio.1002171
Eskelinen, H. C., Winship, K. A., Jones, B. L., Ames, A. E. M., and Kuczaj, S. A. (2016). Acoustic behavior associated with cooperative task success in bottlenose dolphins (Tursiops truncatus). Anim. Cogn. 19, 789–797. doi:10.1007/s10071-016-0978-1
Esposito, A., and Esposito, A. (2011). “On speech and gestures synchrony,” in Analysis of Verbal and Nonverbal Communication and Enactment., Volume 6800 of Lecture Notes in Computer Science, eds A. Esposito, A. Vinciarelli, K. Vicsi, C. Pelachaud, and A. Nijholt (Berlin, Heidelberg: Springer), 252–272.
Eyssel, F., Kuchenbrandt, D., Bobinger, S., de Ruiter, L., and Hegel, F. (2012). “‘If you sound like me, you must be more human’: on the interplay of robot and user features on human-robot acceptance and anthropomorphism,” in Proceedings of the Seventh Annual ACM/IEEE International Conference on Human-Robot Interaction – HRI ’12 (New York, NY: ACM Press), 125.
Fang, G., Jiang, F., Yang, P., Cui, J., Brauth, S. E., and Tang, Y. (2014). Male vocal competition is dynamic and strongly affected by social contexts in music frogs. Anim. Cogn. 17, 483–494. doi:10.1007/s10071-013-0680-5
Feldman, J. A. (2008). From Molecules to Metaphor: A Neural Theory of Language. Cambridge: Bradford Books.
Fellous, J., and Arbib, M. (2005). Who Needs Emotions? The Brain Meets the Robot. Oxford, NY: Oxford University Press.
Fernald, A. (1985). Four-month-old infants prefer to listen to motherese. Infant Behav. Dev. 8, 181–195. doi:10.1016/S0163-6383(85)80005-9
Fichtel, C., and van Schaik, C. P. (2006). Semantic differences in sifaka (Propithecus verreauxi) alarm calls: a reflection of genetic or cultural variants? Ethology 112, 839–849. doi:10.1111/j.1439-0310.2006.01239.x
Ficken, M. S., and Popp, J. W. (1996). A comparative analysis of passerine mobbing calls. Auk 113, 370–380. doi:10.2307/4088904
Fitch, W. T. (2000). The evolution of speech: a comparative review. Trends Cogn. Sci. 4, 258–267. doi:10.1016/S1364-6613(00)01494-7
Fitch, W. T. (2013). Rhythmic cognition in humans and animals: distinguishing meter and pulse perception. Front. Syst. Neurosci. 7:68. doi:10.3389/fnsys.2013.00068
Fitch, W. T., and Reby, D. (2001). The descended larynx is not uniquely human. Proc. Biol. Sci. 268, 1669–1675. doi:10.1098/rspb.2001.1704
Friston, K., and Frith, C. (2015). A duet for one. Conscious. Cogn. 36, 390–405. doi:10.1016/j.concog.2014.12.003
Friston, K., and Kiebel, S. (2009). Predictive coding under the free-energy principle. Philos. Trans. R. Soc. Lond. B Biol. Sci. 364, 1211–1221. doi:10.1098/rstb.2008.0300
Fusaroli, R., Raczaszek-Leonardi, J., and Tylén, K. (2014). Dialog as interpersonal synergy. New Ideas Psychol. 32, 147–157. doi:10.1016/j.newideapsych.2013.03.005
Gales, M., and Young, S. J. (2007). The application of hidden Markov models in speech recognition. Found. Trends Signal Process. 1, 195–304. doi:10.1561/2000000004
Ganger, J., and Brent, M. R. (2004). Reexamining the vocabulary spurt. Dev. Psychol. 40, 621–632. doi:10.1037/0012-1649.40.4.621
Garrod, S., Gambi, C., and Pickering, M. J. (2013). Prediction at all levels: forward model predictions can enhance comprehension. Lang. Cogn. Neurosci. 29, 46–48. doi:10.1080/01690965.2013.852229
Gil, D., and Gahr, M. (2002). The honesty of bird song: multiple constraints for multiple traits. Trends Ecol. Evol. 17, 133–141. doi:10.1016/S0169-5347(02)02410-2
Gillespie-Lynch, K., Greenfield, P. M., Feng, Y., Savage-Rumbaugh, S., and Lyn, H. (2013). A cross-species study of gesture and its role in symbolic development: implications for the gestural theory of language evolution. Front. Psychol. 4:160. doi:10.3389/fpsyg.2013.00160
Gisiner, R., and Schusterman, R. J. (1991). California sea lion pups play an active role in reunions with their mothers. Anim. Behav. 41, 364–366. doi:10.1016/S0003-3472(05)80488-9
Goldfield, B. A., and Reznick, J. S. (1990). Early lexical acquisition: rate, content, and the vocabulary spurt. J. Child Lang. 17, 171–183. doi:10.1017/S0305000900013167
Gopnik, A., Meltzoff, A. N., and Kuhl, P. K. (2001). The Scientist in the Crib. New York City, US: Perennial.
Grafe, T. U., Bitz, J. H., and Wink, M. (2004). Song repertoire and duetting behaviour of the tropical boubou, Laniarius aethiopicus. Anim. Behav. 68, 181–191. doi:10.1016/j.anbehav.2003.11.004
Greene, E., and Meagher, T. (1998). Red squirrels, Tamiasciurus hudsonicus, produce predator-class specific alarm calls. Anim. Behav. 55, 511–518. doi:10.1006/anbe.1997.0620
Gridi-Papp, M., Rand, A. S., and Ryan, M. J. (2006). Animal communication: complex call production in the túngara frog. Nature 441, 38. doi:10.1038/441038a
Griesser, M. (2009). Mobbing calls signal predator category in a kin group-living bird species. Proc. Biol. Sci. 276, 2887–2892. doi:10.1098/rspb.2009.0551
Griffiths, P. E., and Scarantino, A. (2005). “Emotions in the wild: the situated perspective on emotion,” in Cambridge Handbook of Situated Cognition, eds P. Robbins and M. Aydede (Cambridge: Cambridge University Press), 437–453.
Hage, S. R., Jiang, T., Berquist, S. W., Feng, J., and Metzner, W. (2013). Ambient noise induces independent shifts in call frequency and amplitude within the Lombard effect in echolocating bats. Proc. Natl. Acad. Sci. U.S.A. 110, 4063–4068. doi:10.1073/pnas.1211533110
Halfwerk, W., Lea, A., Guerra, M., Page, R., and Ryan, M. J. (2016). Vocal responses to noise reveal the presence of the Lombard effect in a frog. Behav. Ecol. 27, 669–676. doi:10.1093/beheco/arv204
Hall, M. L. (2004). A review of hypotheses for the functions of avian duetting. Behav. Ecol. Sociobiol. 55, 415–430. doi:10.1007/s00265-003-0741-x
Hall, M. L., Kingma, S. A., and Peters, A. (2013). Male songbird indicates body size with low-pitched advertising songs. PLoS ONE 8:e56717. doi:10.1371/journal.pone.0056717
Hanggi, E. B., and Schusterman, R. J. (1990). Kin recognition in captive California sea lions (Zalophus californianus). J. Compa. Psychol. 104, 368–372. doi:10.1037/0735-7036.104.4.368
Haring, M., Bee, N., and Andre, E. (2011). “Creation and evaluation of emotion expression with body movement, sound and eye color for humanoid robots,” in RO-MAN (Atlanta: IEEE), 204–209.
Harnad, S. (1990). The symbol grounding problem. Physica D 42, 335–346. doi:10.1016/0167-2789(90)90087-6
Harrington, F. H., and Mech, L. D. (1983). Wolf pack spacing: howling as a territory-independent spacing mechanism in a territorial population. Behav. Ecol. Sociobiol. 12, 161–168. doi:10.1007/BF00343208
Hauser, M. D., Chomsky, N., and Fitch, W. T. (2002). The faculty of language: what is it, who has it, and how did it evolve? Science 298, 1569–1579. doi:10.1126/science.298.5598.1569
Hinton, G., Deng, L., Yu, D., Dahl, G. E., Mohamed, A., Jaitly, N., et al. (2012). Deep neural networks for acoustic modeling in speech recognition: the shared views of four research groups. IEEE Signal Process. Mag. 29, 82–97. doi:10.1109/MSP.2012.2205597
Holler, J., Schubotz, L., Kelly, S., Hagoort, P., Schuetze, M., and Özyürek, A. (2014). Social eye gaze modulates processing of speech and co-speech gesture. Cognition 133, 692–697. doi:10.1016/j.cognition.2014.08.008
Hooper, S., Reiss, D., Carter, M., and McCowan, B. (2006). Importance of contextual saliency on vocal imitation by bottlenose dolphins. Int. J. Comp. Psychol. 19, 116–128.
Horowitz, A., and Hecht, J. (2016). Examining dog-human play: the characteristics, affect, and vocalizations of a unique interspecific interaction. Anim. Cogn. 19, 779–788. doi:10.1007/s10071-016-0976-3
Hotchkin, C. F., Parks, S. E., and Weiss, D. J. (2013). Vocal modifications in primates: effects of noise and behavioral context on vocalization structure. Proc. Meet. Acoust. 19, 010061. doi:10.1121/1.4799257
Howard, I. S., and Messum, P. (2014). Learning to pronounce first words in three languages: an investigation of caregiver and infant behavior using a computational model of an infant. PLoS ONE 9:e110334. doi:10.1371/journal.pone.0110334
Hurd, C. R. (1996). Interspecific attraction to the mobbing calls of black-capped chickadees (Parus atricapillus). Behav. Ecol. Sociobiol. 38, 287–292. doi:10.1007/s002650050244
Insley, S. J. (2001). Mother-offspring vocal recognition in northern fur seals is mutual but asymmetrical. Anim. Behav. 61, 129–137. doi:10.1006/anbe.2000.1569
Ishihara, H., Yoshikawa, Y., Miura, K., and Asada, M. (2009). How caregiver’s anticipation shapes infant’s vowel through mutual imitation. IEEE Trans. Auton. Ment. Dev. 1, 217–225. doi:10.1109/TAMD.2009.2038988
Jarvis, E. D. (2004). Learned birdsong and the neurobiology of human language. Ann. N. Y. Acad. Sci. 1016, 749–777. doi:10.1196/annals.1298.038
Jarvis, E. D. (2006a). “Evolution of vocal learning systems in birds and humans,” in Evolution of Nervous Systems, Vol. 2, ed. J. Kaas (Tokyo: Academic Press), 213–228.
Jarvis, E. D. (2006b). Selection for and against vocal learning in birds and mammals. Ornithol. Sci. 5, 5–14. doi:10.2326/osj.5.5
Jones, T., Lawson, S., and Mills, D. (2008). “Interaction with a zoomorphic robot that exhibits canid mechanisms of behaviour,” in IEEE International Conference on Robotics and Automation, 2008. ICRA 2008 (Washington, DC), 2128–2133.
Joslin, P. W. B. (1967). Movements and homesites of timber wolves in Algonquin Park. Am. Zool. 7, 279–288. doi:10.1093/icb/7.2.279
Kaminski, J., Call, J., and Fischer, J. (2004). Word learning in a domestic dog: evidence for “fast mapping”. Science 304, 1682–1683. doi:10.1126/science.1097859
Kershenbaum, A., Blumstein, D. T., Roch, M. A., Akçay, Ç, Backus, G., Bee, M. A., et al. (2016). Acoustic sequences in non-human animals: a tutorial review and prospectus. Biol. Rev. Camb. Philos. Soc. 91, 13–52. doi:10.1111/brv.12160
Kershenbaum, A., Ilany, A., Blaustein, L., and Geffen, E. (2012). Syntactic structure and geographical dialects in the songs of male rock hyraxes. Proc. Biol. Sci. 279, 2974–2981. doi:10.1098/rspb.2012.0322
Kershenbaum, A., Sayigh, L. S., and Janik, V. M. (2013). The encoding of individual identity in dolphin signature whistles: how much information is needed? PLoS ONE 8:e77671. doi:10.1371/journal.pone.0077671
King, S. L., Harley, H. E., and Janik, V. M. (2014). The role of signature whistle matching in bottlenose dolphins, Tursiops truncatus. Anim. Behav. 96, 79–86. doi:10.1016/j.anbehav.2014.07.019
King, S. L., and Janik, V. M. (2013). Bottlenose dolphins can use learned vocal labels to address each other. Proc. Natl. Acad. Sci. U.S.A. 110, 13216–13221. doi:10.1073/pnas.1304459110
Kirsch, J. A., Gntrkn, O., and Rose, J. (2008). Insight without cortex: lessons from the avian brain. Conscious. Cogn. 17, 475–483; Social Cognition, Emotion, and Self-Consciousness. doi:10.1016/j.concog.2008.03.018
Knight, C., Studdert-Kennedy, M., and Hurford, J. R. (2000). The Evolutionary Emergence of Language. Cambridge: Cambridge University Press.
Kobayasi, K. I., and Okanoya, K. (2003). Context-dependent song amplitude control in Bengalese finches. Neuroreport 14, 521–524. doi:10.1097/01.wnr.0000059626.96928.52
Kopp, S. (2010). Social resonance and embodied coordination in face-to-face conversation with artificial interlocutors. Speech Commun. 52, 587–597. doi:10.1016/j.specom.2010.02.007
Kuhl, P. K. (1981). Discrimination of speech by nonhuman animals: basic auditory sensitivities conducive to the perception of speech-sound categories. J. Acoust. Soc. Am. 70, 340. doi:10.1121/1.386782
Kuhl, P. K. (2000). A new view of language acquisition. Proc. Natl. Acad. Sci. U.S.A. 97, 11850–11857. doi:10.1073/pnas.97.22.11850
Kuhl, P. K. (2004). Early language acquisition: cracking the speech code. Nat. Rev. Neurosci. 5, 831–843. doi:10.1038/nrn1533
Leonard, M. L., and Horn, A. G. (2005). Ambient noise and the design of begging signals. Proc. Biol. Sci. 272, 651–656. doi:10.1098/rspb.2004.3021
Levinson, S. C. (2006). “On the human “interaction engine”,” in Roots of Human Sociality: Culture, Cognition and Interaction, eds N. J. Enfield and S. C. Levinson (Oxford: Berg), 39–69.
Levinson, S. C. (2015). Turn-taking in human communication? Origins and implications for language processing. Trends Cogn. Sci. 20, 6–14. doi:10.1016/j.tics.2015.10.010
Liebal, K., Waller, B. M., Burrows, A. M., and Slocombe, K. E. (2013). Primate Communication: A Multimodal Approach. Cambridge: Cambridge University Press.
Lieberman, P. (1984). The Biology and Evolution of Language. Cambridge, MA: Harvard University Press.
Lim, A., and Okuno, H. G. (2014). The MEI robot: towards using motherese to develop multimodal emotional intelligence. IEEE Trans. Auton. Ment. Dev. 6, 126–138. doi:10.1109/TAMD.2014.2317513
Lind, H., Dabelsteen, T., and Gregor, P. K. M. C. (1996). Female great tits can identify mates by song. Anim. Behav. 52, 667–671. doi:10.1006/anbe.1996.0211
Lindblom, B. (1990). “Explaining phonetic variation: a sketch of the h&h theory,” in Speech Production and Speech Modelling, eds W. J. Hardcastle and A. Marchal (Dordrecht: Kluwer Academic Publishers), 403–439.
Lipkind, D., Marcus, G. F., Bemis, D. K., Sasahara, K., Jacoby, N., Takahasi, M., et al. (2013). Stepwise acquisition of vocal combinatorial capacity in songbirds and human infants. Nature 498, 104–108. doi:10.1038/nature12173
Lombard, E. (1911). Le sign de l’élévation de la voix. Ann. Maladies Oreille Larynx Nez Pharynx 37, 101–119.
Lopez Cozar Delgado, R., and Araki, M. (2005). Spoken, Multilingual and Multimodal Dialogue Systems: Development and Assessment. West Sussex: Wiley.
Lyon, C., Nehaniv, C. L., and Cangelosi, A. (2007). Emergence of Communication and Language. London: Springer.
Ma, Z. S. (2015). Towards computational models of animal communications, an introduction for computer scientists. Cogn. Syst. Res. 33, 70–99. doi:10.1016/j.cogsys.2014.08.002
MacNeilage, P. (1998). The frame/content theory of evolution of speech production. Behav. Brain Sci. 21, 499–546. doi:10.1017/S0140525X98001265
Manabe, K., Sadr, E. I., and Dooling, R. J. (1998). Control of vocal intensity in budgerigars (Melopsittacus undulatus): differential reinforcement of vocal intensity and the Lombard effect. J. Acoust. Soc. Am. 103, 1190–1198. doi:10.1121/1.421227
Manser, M. B. (2001). The acoustic structure of suricates’ alarm calls varies with predator type and the level of response urgency. Proc. Biol. Sci. 268, 2315–2324. doi:10.1098/rspb.2001.1773
Maturana, H. R., and Varela, F. J. (1987). The Tree of Knowledge: The Biological Roots of Human Understanding. Boston, MA: New Science Library/Shambhala Publications.
Mavridis, N. (2014). A review of verbal and non-verbal human? robot interactive communication. Rob. Auton. Syst. 63, 22–35. doi:10.1016/j.robot.2014.09.031
McCarthy, D. (1954). “Language development in children,” in Manual of Child Psychology, 2nd Edn, ed. L. Carmichael (New York: John Wiley & Sons), 492–630.
McComb, K., Shannon, G., Sayialel, K. N., and Moss, C. (2014). Elephants can determine ethnicity, gender, and age from acoustic cues in human voices. Proc. Natl. Acad. Sci. U.S.A. 111, 5433–5438. doi:10.1073/pnas.1321543111
McCowan, B., Hanser, S. F., and Doyle, L. R. (1999). Quantitative tools for comparing animal communication systems: information theory applied to bottlenose dolphin whistle repertoires. Anim. Behav. 57, 409–419. doi:10.1006/anbe.1998.1000
McTear, M. F. (2004). Spoken Dialogue Technology: Towards the Conversational User Interface. London: Springer.
Mehrabian, A. (1996). Pleasure-arousal-dominance: a general framework for describing and measuring individual differences in temperament. Curr. Psychol. 14, 261–292. doi:10.1007/BF02686918
Mennill, D. J. (2006). Aggressive responses of male and female rufous-and-white wrens to stereo duet playback. Anim. Behav. 71, 219–226. doi:10.1016/j.anbehav.2005.05.006
Mennill, D. J., Boag, P. T., and Ratcliffe, L. M. (2003). The reproductive choices of eavesdropping female black-capped chickadees, Poecile atricapillus. Naturwissenschaften 90, 577–582. doi:10.1007/s00114-003-0479-3
Messum, P., and Howard, I. S. (2015). Creating the cognitive form of phonological units: the speech sound correspondence problem in infancy could be solved by mirrored vocal interactions rather than by imitation. J. Phon. 53, 125–140. doi:10.1016/j.wocn.2015.08.005
Mitchell, W. J., Szerszen, K. A. Sr., Lu, A. S., Schermerhorn, P. W., Scheutz, M., and MacDorman, K. F. (2011). A mismatch in the human realism of face and voice produces an uncanny valley. Iperception 2, 10–12. doi:10.1068/i0415
Miura, K., Yoshikawa, Y., and Asada, M. (2012). Unconscious anchoring in maternal imitation that helps find the correspondence of a caregiver’s vowel categories. Adv. Robot. 21, 1583–1600. doi:10.1163/156855307782148596
Miyagawa, S., Ojima, S., Berwick, R. C., and Okanoya, K. (2014). The integration hypothesis of human language evolution and the nature of contemporary languages. Front. Psychol. 5:564. doi:10.3389/fpsyg.2014.00564
Moore, R. K. (2007a). PRESENCE: a human-inspired architecture for speech-based human-machine interaction. IEEE Trans. Comput. 56, 1176–1188. doi:10.1109/TC.2007.1080
Moore, R. K. (2007b). Spoken language processing: piecing together the puzzle. Speech Commun. 49, 418–435. doi:10.1016/j.specom.2007.01.011
Moore, R. K. (2010). “Cognitive approaches to spoken language technology,” in Speech Technology: Theory and Applications, eds F. Chen and K. Jokinen (New York, Dordrecht, Heidelberg, London: Springer), 89–103.
Moore, R. K. (2012). A Bayesian explanation of the ‘Uncanny Valley’ effect and related psychological phenomena. Sci. Rep. 2, 864. doi:10.1038/srep00864
Moore, R. K. (2013). “Spoken language processing: where do we go from here?” in Your Virtual Butler, LNAI, Vol. 7407, ed. R. Trappl (Heidelberg: Springer), 111–125.
Moore, R. K. (2015). “From talking and listening robots to intelligent communicative machines,” in Robots That Talk and Listen, Chap. 12, ed. J. Markowitz (Boston, MA: De Gruyter), 317–335.
Moore, R. K. (2016a). “A real-time parametric general-purpose mammalian vocal synthesiser,” in INTERSPEECH, San Francisco, CA.
Moore, R. K. (2016b). “Is spoken language all-or-nothing? Implications for future speech-based human-machine interaction,” in Dialogues with Social Robots Enablements, Analyses, and Evaluation, eds K. Jokinen and G. Wilcock (Springer Lecture Notes in Electrical Engineering (LNEE)). Available at: http://www.springer.com/us/book/9789811025846#aboutBook
Moore, R., and Morris, A. (1992). “Experiences collecting genuine spoken enquiries using WOZ techniques,” in Proceedings of the workshop on Speech and Natural Language (HLT ’91) (Stroudsburg, PA: Association for Computational Linguistics), 61–63.
Moore, R. K., and ten Bosch, L. (2009). “Modelling vocabulary growth from birth to young adulthood,” in INTERSPEECH (Brighton), 1727–1730.
Morse, A. F., Benitez, V. L., Belpaeme, T., Cangelosi, A., and Smith, L. B. (2015). Posture affects how robots and infants map words to objects. PLoS ONE 10:e0116012. doi:10.1371/journal.pone.0116012
Morse, A. F., Herrera, C., Clowes, R., Montebelli, A., and Ziemke, T. (2011). The role of robotic modelling in cognitive science. New Ideas Psychol. 29, 312–324. doi:10.1016/j.newideapsych.2011.02.001
Moulin-Frier, C., Nguyen, S. M., and Oudeyer, P.-Y. (2013). Self-organization of early vocal development in infants and machines: the role of intrinsic motivation. Front. Psychol. 4:1006. doi:10.3389/fpsyg.2013.01006
Nass, C., and Brave, S. (2005). Wired for Speech: How Voice Activates and Advances the Human-Computer Relationship. Cambridge: MIT Press.
Nazzi, T., and Bertoncini, J. (2003). Before and after the vocabulary spurt: two modes of word acquisition? Dev. Sci. 6, 136–142. doi:10.1111/1467-7687.00263
Nguyen, N., and Delvaux, V. (2015). Role of imitation in the emergence of phonological systems. J. Phon. 53, 46–54. doi:10.1016/j.wocn.2015.08.004
Niculescu, A., van Dijk, B., Nijholt, A., and See, S. L. (2011). “The influence of voice pitch on the evaluation of a social robot receptionist,” in International Conference on User Science and Engineering (i-USEr) (Shah Alam: IEEE), 18–23.
Nolfi, S., and Mirolli, M. (2010). Evolution of Communication and Language in Embodied Agents. Berlin, Heidelberg: Springer.
Nonaka, S., Takahashi, R., Enomoto, K., Katada, A., and Unno, T. (1997). Lombard reflex during PAG-induced vocalization in decerebrate cats. Neurosci. Res. 29, 283–289. doi:10.1016/S0168-0102(97)00097-7
Oller, D. K. (2004). Evolution of Communication Systems: A Comparative Approach. Cambridge: MIT Press.
Osmanski, M. S., and Dooling, R. J. (2009). The effect of altered auditory feedback on control of vocal production in budgerigars (Melopsittacus undulatus). J. Acoust. Soc. Am. 126, 911–919. doi:10.1121/1.3158928
Ouattara, K., Lemasson, A., and Zuberbühler, K. (2009). Campbell’s monkeys concatenate vocalizations into context-specific call sequences. Proc. Natl. Acad. Sci. U.S.A. 106, 22026–22031. doi:10.1073/pnas.0908118106
Oztop, E., Kawato, M., and Arbib, M. (2006). Mirror neurons and imitation: a computationally guided review. Neural Netw. 19, 254–271; The Brain Mechanisms of Imitation Learning. doi:10.1016/j.neunet.2006.02.002
Pepperberg, I. M. (2010). Vocal learning in Grey parrots: a brief review of perception, production, and cross-species comparisons. Brain Lang. 115, 81–91. doi:10.1016/j.bandl.2009.11.002
Perez, E. C., Elie, J. E., Soulage, C. O., Soula, H. A., Mathevon, N., and Vignal, C. (2012). The acoustic expression of stress in a songbird: does corticosterone drive isolation-induced modifications of zebra finch calls? Horm. Behav. 61, 573–581. doi:10.1016/j.yhbeh.2012.02.004
Peterson, R. S., and Bartholomew, G. A. (1969). Airborne vocal communication in the California sea lion, Zalophus californianus. Anim. Behav. 17, 17–24. doi:10.1016/0003-3472(69)90108-0
Pfaff, J. A., Zanette, L., MacDougall-Shackleton, S. A., and MacDougall-Shackleton, E. A. (2007). Song repertoire size varies with HVC volume and is indicative of male quality in song sparrows (Melospiza melodia). Proc. Biol. Sci. 274, 2035–2040. doi:10.1098/rspb.2007.0170
Phillips, M., and Philips, M. (2006). “Applications of spoken language technology and systems,” in IEEE/ACL Workshop on Spoken Language Technology (SLT), eds M. Gilbert and H. Ney (Aruba: IEEE), 7.
Pickering, M. J., and Garrod, S. (2007). Do people use language production to make predictions during comprehension? Trends Cogn. Sci. 11, 105–110. doi:10.1016/j.tics.2006.12.002
Pinker, S., and Jackendoff, R. (2005). The faculty of language: what’s special about it? Cognition 95, 201–236. doi:10.1016/j.cognition.2004.08.004
Pisanski, K., Cartei, V., McGettigan, C., Raine, J., and Reby, D. (2016). Voice modulation: a window into the origins of human vocal control? Trends Cogn. Sci. 20, 304–318. doi:10.1016/j.tics.2016.01.002
Plutchik, R. (1980). “A general psychoevolutionary theory of emotion,” in Emotion: Theory, Research and Experience: Vol. 1. Theories of Emotion, eds R. Plutchik and H. Kellerman (New York: Academic Press), 3–33.
Pongrácz, P., Molnár, C., and Miklósi, Á (2006). Acoustic parameters of dog barks carry emotional information for humans. Appl. Anim. Behav. Sci. 100, 228–240. doi:10.1016/j.applanim.2005.12.004
Poole, J. H., Tyack, P. L., Stoeger-Horwath, A. S., and Watwood, S. (2005). Elephants prove capable of vocal learning. Nature 434, 455–456. doi:10.1038/434455a
Potash, L. M. (1972). Noise-induced changes in calls of the Japanese quail. Psychon. Sci. 26, 252–254. doi:10.3758/BF03328608
Premack, D., and Woodruff, G. (1978). Does the chimpanzee have a theory of mind? Behav. Brain Sci. 1, 515–526. doi:10.1017/S0140525X00076512
Proops, L., McComb, K., and Reby, D. (2009). Cross-modal individual recognition in domestic horses (Equus caballus). Proc. Natl. Acad. Sci. U.S.A. 106, 947–951. doi:10.1073/pnas.0809127105
Rainey, H. J., Zuberbühler, K., and Slater, P. J. B. (2004). Hornbills can distinguish between primate alarm calls. Proc. Biol. Sci. 271, 755–759. doi:10.1098/rspb.2003.2619
Ranganath, R., Jurafsky, D., and McFarland, D. A. (2013). Detecting friendly, flirtatious, awkward, and assertive speech in speed-dates. Comput. Speech Lang. 27, 89–115. doi:10.1016/j.csl.2012.01.005
Ravignani, A., Bowling, D., and Fitch, W. T. (2014). Chorusing, synchrony and the evolutionary functions of rhythm. Front. Psychol. 5:1118. doi:10.3389/fpsyg.2014.01118
Ravignani, A., Fitch, W. T., Hanke, F. D., Heinrich, T., Hurgitsch, B., Kotz, S. A., et al. (2016). What pinnipeds have to say about human speech, music, and the evolution of rhythm. Front. Neurosci. 10:274. doi:10.3389/fnins.2016.00274
Reiss, D., and McCowan, B. (1993). Spontaneous vocal mimicry and production by bottlenose dolphins (Tursiops truncatus): evidence for vocal learning. J. Comp. Psychol. 107, 301–312. doi:10.1037/0735-7036.107.3.301
Ridley, A. R., Child, M. F., and Bell, M. B. V. (2007). Interspecific audience effects on the alarm-calling behaviour of a kleptoparasitic bird. Biol. Lett. 3, 589–591. doi:10.1098/rsbl.2007.0325
Rizzolatti, G., and Craighero, L. (2004). The mirror-neuron system. Annu. Rev. Neurosci. 27, 169–192. doi:10.1146/annurev.neuro.27.070203.144230
Roy, B. C., Frank, M. C., DeCamp, P., Miller, M., and Roy, D. (2015). Predicting the birth of a spoken word. Proc. Natl. Acad. Sci. U.S.A. 112, 201419773. doi:10.1073/pnas.1419773112
Russell, J. A. (1980). A circumplex model of affect. J. Pers. Soc. Psychol. 39, 1161–1178. doi:10.1037/h0077714
Saffran, J. R. (2003). Statistical language learning: mechanisms and constraints. Curr. Dir. Psychol. Sci. 12, 110–114. doi:10.1111/1467-8721.01243
Saffran, J. R., Aslin, R. N., and Newport, E. (1996). Statistical learning by 8-month old infants. Science 274, 1926–1928. doi:10.1126/science.274.5294.1926
Sasahara, K., Cody, M. L., Cohen, D., and Taylor, C. E. (2012). Structural design principles of complex bird songs: a network-based approach. PLoS ONE 7:e44436. doi:10.1371/journal.pone.0044436
Schel, A. M., Candiotti, A., and Zuberbühler, K. (2010). Predator-deterring alarm call sequences in Guereza colobus monkeys are meaningful to conspecifics. Anim. Behav. 80, 799–808. doi:10.1016/j.anbehav.2010.07.012
Schel, A. M., Townsend, S. W., Machanda, Z., Zuberbühler, K., and Slocombe, K. E. (2013). Chimpanzee alarm call production meets key criteria for intentionality. PLoS ONE 8:e76674. doi:10.1371/journal.pone.0076674
Scherer, K. R. (2003). Vocal communication of emotion: a review of research paradigms. Speech Commun. 40, 227–256. doi:10.1016/S0167-6393(02)00084-5
Schusterman, R. J. (1977). Temporal patterning in sea lion barking (Zalophus californianus). Behav. Biol. 20, 404–408. doi:10.1016/S0091-6773(77)90964-6
Schwenk, M., and Arras, K. O. (2014). “R2-D2 reloaded: a flexible sound synthesis system for sonic human-robot interaction design,” in The 23rd IEEE International Symposium on Robot and Human Interactive Communication (Edinburgh: IEEE), 161–167.
Scott-Phillips, T. (2015). Speaking Our Minds: Why Human Communication Is Different, and How Language Evolved to Make It Special. London: Palgrave MacMillan.
Searcy, W. A., and Yasukawa, K. (1996). “Song and female choice,” in Ecology and Evolution of Acoustic Communication in Birds, eds D. E. Kroodsma, and E. H. Miller (Ithaca, NY: Comstock Publishing Associates), 454–473.
Seyfarth, R. M., and Cheney, D. L. (2003). Meaning and emotion in animal vocalizations. Ann. N. Y. Acad. Sci. 1000, 32–55. doi:10.1196/annals.1280.004
Seyfarth, R. M., Cheney, D. L., and Marler, P. (1980). Monkey responses to three different alarm calls: evidence of predator classification and semantic communication. Science 210, 801–803. doi:10.1126/science.7433999
Shannon, R. V. (2016). Is birdsong more like speech or music? Trends Cogn. Sci. 20, 245–247. doi:10.1016/j.tics.2016.02.004
Smith, L. B., and Yu, C. (2008). Infants rapidly learn word referent mappings via cross-situational statistics. Cognition 106, 1558–1568. doi:10.1016/j.cognition.2007.06.010
Soltis, J., Leighty, K. A., Wesolek, C. M., and Savage, A. (2009). The expression of affect in African elephant (Loxodonta africana) rumble vocalizations. J. Comp. Psychol. 132, 222–225. doi:10.1037/a0015223
Stark, R. (1980). “Stages of speech development in the first year of life,” in Child Phonology, eds G. H. Yeni-Komshian, J. F. Kavanagh, and C. A. Ferguson (New York: Academic Press), 113–142.
Steels, L. (2001). Language games for autonomous robots. IEEE Intell. Syst. 16, 16–22. doi:10.1109/5254.956077
Steels, L. (2003). Evolving grounded communication for robots. Trends Cogn. Sci. 7, 308–312. doi:10.1016/S1364-6613(03)00129-3
Stephan, C., and Zuberbühler, K. (2008). Predation increases acoustic complexity in primate alarm calls. Biol. Lett. 4, 641–644. doi:10.1098/rsbl.2008.0488
Stramandinoli, F., Marocco, D., and Cangelosi, A. (2012). The grounding of higher order concepts in action and language: a cognitive robotics model. Neural Netw. 32, 165–173; Selected Papers from {IJCNN} 2011. doi:10.1016/j.neunet.2012.02.012
Takahashi, D. Y., Narayanan, D. Z., and Ghazanfar, A. A. (2013). Coupled oscillator dynamics of vocal turn-taking in monkeys. Curr. Biol. 23, 2162–2168. doi:10.1016/j.cub.2013.09.005
Talkington, W. J., Rapuano, K. M., Hitt, L. A., Frum, C. A., and Lewis, J. W. (2012). Humans mimicking animals: a cortical hierarchy for human vocal communication sounds. J. Neurosci. 32, 8084–8093. doi:10.1523/JNEUROSCI.1118-12.2012
Tchernichovski, O., Mitra, P. P., Lints, T., and Nottebohm, F. (2001). Dynamics of the vocal imitation process: how a zebra finch learns its song. Science 291, 2564–2569. doi:10.1126/science.1058522
Templeton, C. N., and Greene, E. (2007). Nuthatches eavesdrop on variations in heterospecific chickadee mobbing alarm calls. Proc. Natl. Acad. Sci. U.S.A. 104, 5479–5482. doi:10.1073/pnas.0605183104
Templeton, C. N., Greene, E., and Davis, K. (2005). Allometry of alarm calls: black-capped chickadees encode information about predator size. Science 308, 1934–1937. doi:10.1126/science.1108841
Templeton, C. N., Mann, N. I., Ríos-Chelén, A. A., Quiros-Guerrero, E., Macías Garcia, C., and Slater, P. J. (2013). An experimental study of duet integration in the happy wren, Pheugopedius felix. Anim. Behav. 86, 821–827. doi:10.1016/j.anbehav.2013.07.022
ten Bosch, L., Boves, L., Van Hamme, H., and Moore, R. K. (2009). A computational model of language acquisition: the emergence of words. Fundam. Inform. 90, 229–249. doi:10.3233/FI-2009-0016
ten Cate, C. (2014). On the phonetic and syntactic processing abilities of birds: from songs to speech and artificial grammars. Curr. Opin. Neurobiol. 28, 157–164. doi:10.1016/j.conb.2014.07.019
ten Cate, C., and Okanoya, K. (2012). Revisiting the syntactic abilities of non-human animals: natural vocalizations and artificial grammar learning. Philos. Trans. R. Soc. Lond. B Biol. Sci. 367, 1984–1994. doi:10.1098/rstb.2012.0055
Thill, S., Caligiore, D., Borghi, A. M., Ziemke, T., and Baldassarre, G. (2013). Theories and computational models of affordance and mirror systems: an integrative review. Neurosci. Biobehav. Rev. 37, 491–521. doi:10.1016/j.neubiorev.2013.01.012
Thill, S., and Lowe, R. (2012). “On the functional contributions of emotion mechanisms to (artificial) cognition and intelligence,” in Proceedings of the Fifth Conference on Artificial General Intelligence, LNAI 7716, eds J. Bach, B. Goertzel, and M. Ilké (Heidelberg: Springer), 322–331.
Thill, S., Padó, S., and Ziemke, T. (2014). On the importance of a rich embodiment in the grounding of concepts: perspectives from embodied cognitive science and computational linguistics. Top. Cogn. Sci. 6, 545–558. doi:10.1111/tops.12093
Thill, S., and Twomey, K. E. (2016). What’s on the inside counts: a grounded account of concept acquisition and development. Front. Psychol. 7:402. doi:10.3389/fpsyg.2016.00402
Tomasello, M., Carpenter, M., Call, J., Behne, T., and Moll, H. (2005). Understanding and sharing intentions: the origins of cultural cognition. Behav. Brain Sci. 28, 675–735. doi:10.1017/S0140525X05000129
Townsend, S. W., Koski, S. E., Byrne, R. W., Slocombe, K. E., Bickel, B., Boeckle, M., et al. (2016). Exorcising Grice’s ghost: an empirical approach to studying intentional communication in animals. Biol. Rev. doi:10.1111/brv.12289
Trillmich, F. (1981). Mutual mother-pup recognition in galápagos fur seals and sea lions: cues used and functional significance. Behaviour 78, 21–42. doi:10.1163/156853981X00248
Vallet, E., Beme, I., and Kreutzer, M. (1998). Two-note syllables in canary songs elicit high levels of sexual display. Anim. Behav. 55, 291–297. doi:10.1006/anbe.1997.0631
Vernes, S. C. (2016). What bats have to say about speech and language. Psychon. Bull. Rev. 1–7. doi:10.3758/s13423-016-1060-3
Vinciarelli, A., Pantic, M., and Bourlard, H. (2009). Social signal processing: survey of an emerging domain. Image Vis. Comput. 27, 1743–1759. doi:10.1016/j.imavis.2008.11.007
Vollmer, A.-L., Wrede, B., Rohlfing, K. J., and Cangelosi, A. (2013). “Do beliefs about a robot’s capabilities influence alignment to its actions?” in IEEE Third Joint International Conference on Development and Learning and Epigenetic Robotics (ICDL), 2013 (Osaka), 1–6.
Volodin, I. A., Volodina, E. V., Lapshina, E. N., Efremova, K. O., and Soldatova, N. V. (2014). Vocal group signatures in the goitred gazelle Gazella subgutturosa. Anim. Cogn. 17, 349–357. doi:10.1007/s10071-013-0666-3
von Humboldt, W. (1836). Uber die verschiedenheit des menschlichen sprachbaues und ihren einfuss auf die geistige entwickelung des menschengeschlechts. Berlin: Royal Academy of Science.
Wagner, P., Malisz, Z., and Kopp, S. (2014). Gesture and speech in interaction: an overview. Speech Commun. 57, 209–232. doi:10.1016/j.specom.2013.09.008
Waiblinger, S., Boivin, X., Pedersen, V., Tosi, M. V., Janczak, A. M., Visser, E. K., et al. (2006). Assessing the human-animal relationship in farmed species: a critical review. Appl. Anim. Behav. Sci. 101, 185–242. doi:10.1016/j.applanim.2006.02.001
Walters, M., Syrdal, D., Koay, K., Dautenhahn, K., and te Boekhorst, R. (2008). “Human approach distances to a mechanical-looking robot with different robot voice styles,” in IEEE Int. Symposium on Robot and Human Interactive Communication (Munich, Germany), 707–712.
Watson, S. K., Townsend, S. W., Schel, A. M., Wilke, C., Wallace, E. K., Cheng, L., et al. (2015). Vocal learning in the functionally referential food grunts of chimpanzees. Curr. Biol. 25, 495–499. doi:10.1016/j.cub.2014.12.032
Weary, D. M., and Krebs, J. R. (1992). Great tits classify songs by individual voice characteristics. Anim. Behav. 43, 283–287. doi:10.1016/S0003-3472(05)80223-4
Webb, B. (1995). Using robots to model animals: a cricket test. Rob. Auton. Syst. 16, 117–134. doi:10.1016/0921-8890(95)00044-5
Webb, B. (2008). Using robots to understand animal behavior. Adv. Study Behav. 38, 1–58. doi:10.1016/S0065-3454(08)00001-6
Weiss, M., Hultsch, H., Adam, I., Scharff, C., and Kipper, S. (2014). The use of network analysis to study complex animal communication systems: a study on nightingale song. Proc. Biol. Sci. 281, 20140460. doi:10.1098/rspb.2014.0460
Wermter, S., Page, M., Knowles, M., Gallese, V., Pulvermüller, F., and Taylor, J. (2009). Multimodal communication in animals, humans and robots: an introduction to perspectives in brain-inspired informatics. Neural Netw. 22, 111–115. doi:10.1016/j.neunet.2009.01.004
Wilson, A. D., and Golonka, S. (2013). Embodied cognition is not what you think it is. Front. Psychol. 4:58. doi:10.3389/fpsyg.2013.00058
Wilson, M., and Knoblich, G. (2005). The case for motor involvement in perceiving conspecifics. Psychol. Bull. 131, 460–473. doi:10.1037/0033-2909.131.3.460
Yorzinski, J. L., and Vehrencamp, S. L. (2009). The effect of predator type and danger level on the mob calls of the American crow. Condor 111, 159–168. doi:10.1525/cond.2009.080057
Yoshikawa, Y., Asada, M., Hosoda, K., and Koga, J. (2003). A constructivist approach to infants’ vowel acquisition through mother–infant interaction. Conn. Sci. 15, 245–258. doi:10.1080/09540090310001655075
Zuberbühler, K. (2000). Referential labelling in Diana monkeys. Anim. Behav. 59, 917–927. doi:10.1006/anbe.1999.1317
Zuberbühler, K. (2001). Predator-specific alarm calls in Campbell’s monkeys, Cercopithecus campbelli. Behav. Ecol. Sociobiol. 50, 414–422. doi:10.1007/s002650100383
Zuberbühler, K. (2002). A syntactic rule in forest monkey communication. Anim. Behav. 63, 293–299. doi:10.1006/anbe.2001.1914
Keywords: vocal interaction, speech technology, spoken language, human–robot interaction, animal calls, vocal learning, language evolution, vocal expression
Citation: Moore RK, Marxer R and Thill S (2016) Vocal Interactivity in-and-between Humans, Animals, and Robots. Front. Robot. AI 3:61. doi: 10.3389/frobt.2016.00061
Received: 20 February 2016; Accepted: 28 September 2016;
Published: 25 October 2016
Edited by:
Katerina Pastra, Cognitive Systems Research Institute, GreeceCopyright: © 2016 Moore, Marxer and Thill. This is an open-access article distributed under the terms of the Creative Commons Attribution License (CC BY). The use, distribution or reproduction in other forums is permitted, provided the original author(s) or licensor are credited and that the original publication in this journal is cited, in accordance with accepted academic practice. No use, distribution or reproduction is permitted which does not comply with these terms.
*Correspondence: Roger K. Moore, ci5rLm1vb3JlQHNoZWZmaWVsZC5hYy51aw==