|
ACCESS THE FULL ARTICLE
No SPIE Account? Create one
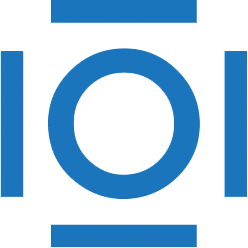
CITATIONS
Cited by 1 patent.
Data modeling
3D modeling
Control systems
Expectation maximization algorithms
Machine learning
Statistical analysis
Statistical modeling