Abstract
Objectives
To examine the impact of the COVID-19 pandemic on deprivation-related inequalities in hospitalisations for cardiovascular disease (CVD) conditions in Denmark and England between March 2018 and December 2021.Design
Time-series studies in England and Denmark.Setting
With the approval of National Health Service England, we used English primary care electronic health records, linked to secondary care and death registry data through the OpenSAFELY platform and nationwide Danish health registry data.Participants
We included adults aged 18 and over without missing age, sex or deprivation information. On 1 March 2020, 16 234 700 people in England and 4 491 336 people in Denmark met the inclusion criteria.Primary outcome measures
Hospital admissions with the primary reason for myocardial infarction (MI), ischaemic or haemorrhagic stroke, heart failure and venous thromboembolism (VTE).Results
We saw deprivation gradients in monthly CVD hospitalisations in both countries, with differences more pronounced in Denmark. Based on pre-pandemic trends, in England, there were an estimated 2608 fewer admissions than expected for heart failure in the most deprived quintile during the pandemic compared with an estimated 979 fewer admissions in the least deprived quintile. For all other outcomes, there was little variation by deprivation quintile. In Denmark, there were an estimated 1013 fewer admissions than expected over the pandemic for MI in the most deprived quintile compared with 619 in the least deprived quintile. Similar trends were seen for stroke and VTE, though absolute numbers were smaller. Heart failure admissions were similar to pre-pandemic levels with little variation by deprivation quintile.Conclusions
Overall, we did not find that the pandemic substantially worsened pre-existing deprivation-related differences in CVD hospitalisations, though there were exceptions in both countries.Free full text

Impacts of the COVID-19 pandemic on deprivation-level differences in cardiovascular hospitalisations: a comparison of England and Denmark using the OpenSAFELY platform and National Registry Data
Abstract
Abstract
Objectives
To examine the impact of the COVID-19 pandemic on deprivation-related inequalities in hospitalisations for cardiovascular disease (CVD) conditions in Denmark and England between March 2018 and December 2021.
Design
Time-series studies in England and Denmark.
Setting
With the approval of National Health Service England, we used English primary care electronic health records, linked to secondary care and death registry data through the OpenSAFELY platform and nationwide Danish health registry data.
Participants
We included adults aged 18 and over without missing age, sex or deprivation information. On 1 March 2020, 16234
700 people in England and 4
491
336 people in Denmark met the inclusion criteria.
Primary outcome measures
Hospital admissions with the primary reason for myocardial infarction (MI), ischaemic or haemorrhagic stroke, heart failure and venous thromboembolism (VTE).
Results
We saw deprivation gradients in monthly CVD hospitalisations in both countries, with differences more pronounced in Denmark. Based on pre-pandemic trends, in England, there were an estimated 2608 fewer admissions than expected for heart failure in the most deprived quintile during the pandemic compared with an estimated 979 fewer admissions in the least deprived quintile. For all other outcomes, there was little variation by deprivation quintile. In Denmark, there were an estimated 1013 fewer admissions than expected over the pandemic for MI in the most deprived quintile compared with 619 in the least deprived quintile. Similar trends were seen for stroke and VTE, though absolute numbers were smaller. Heart failure admissions were similar to pre-pandemic levels with little variation by deprivation quintile.
Conclusions
Overall, we did not find that the pandemic substantially worsened pre-existing deprivation-related differences in CVD hospitalisations, though there were exceptions in both countries.
Introduction
Cardiovascular disease (CVD) is the leading cause of death worldwide accounting for one in four deaths in the UK.1 CVD is known to be associated with important ethnic and socioeconomic health inequalities. Individuals living in deprived areas are more likely to have CVD and have a higher risk of dying from CVD compared with those living in less deprived areas.2,4
While the direct effects of the COVID-19 pandemic have been found to disproportionately affect older people, global majority ethnic groups and deprived populations, inequalities in the indirect effects of the pandemic have yet to be fully explored.5,8 Diversion of healthcare resources to pandemic management has negatively affected non-COVID-related healthcare provision including prevention activities, potentially worsening physical and mental health.9 The negative impacts of the pandemic have been compounded by the rising cost-of-living crisis which has further widened socioeconomic inequalities.10 11 During the early pandemic period (2020), there were reports of fewer CVD admissions.712,14 One systematic review examining the impact of the COVID-19 pandemic on CVD-related care15 highlighted reduced and delayed CVD-related hospital admissions, except for cardiac arrests and increased CVD mortality. In the UK, there were steeper drops in unscheduled hospital admissions in the most deprived compared with the least deprived groups, though this was not specific to CVD admissions.7 However, a Swiss study of deprivation and CVD found that there were no changes in the relative patterning of inequalities resulting from the pandemic.16
The UK experienced one of the worst COVID-19 outbreaks and some of the most severe outcomes from COVID-19.17 In contrast, several Scandinavian countries experienced better COVID-19 outcomes and faster healthcare system recovery.18 Denmark imposed strict restrictions earlier than the UK and other countries. 14 Although the UK imposed more stringent and longer-lasting measures, confirmed COVID-19 deaths were higher in the UK compared with Denmark. This suggests that timeliness of intervention rather than duration was of paramount importance in preventing COVID-19 mortality in the UK compared with Denmark19 (online supplemental figure S1, supplementary materialsonline supplemental materials). Comparing inequalities in the indirect effects of the pandemic between countries with different pandemic curves, where different measures were taken at different times, will be important for informing policy for future infectious disease outbreaks and ensuring that future mitigation measures do not exacerbate inequalities.
We aimed to examine the impact of the COVID-19 pandemic on deprivation-related inequalities in hospitalisations for CVD conditions in Denmark and England between March 2018 and December 2021.
Methods
Using electronic health record and registry data, we conducted two time-series studies using monthly cross-sectional data separately in England and Denmark. The cohorts for each country were defined using comparable inclusion criteria, exposure and outcome definitions and the same statistical analysis techniques were applied (table 1).
Table 1
England | Denmark | |
Inclusion criteria | Adults aged 18 and over, registered with a GP for at least 3 months prior to study entry. | Adults aged 18 and over, recorded and alive at cohort entry according to the Civil Registration System. |
Exclusion criteria | Missing age, sex or patient level IMD, household size>15![]() | Missing age, sex or income. |
Denominator population entry point | Latest of: Meeting inclusion criteria or 1 March 2018. | Latest of: Meeting inclusion criteria or 1 March 2018. |
Denominator population exit point | Earliest of death, deregistering with their GP or end of study period. | Earliest of death, emigration according to the Civil Registration System or end of study period. |
Exposure | ||
Deprivation measurement | Deprivation quintiles based on IMD in the month of interest. | Deprivation quintiles based on household income in 2020. |
Outcomes | ||
Hospital admissions | Hospital admissions with ICD-10 code for heart failure, MI, stroke or VTE as the primary reason for admission (this refers to primary reason for spell in hospital). | Hospital admissions with ICD-10 code for heart failure, MI, stroke or VTE as the primary reason for admission. |
GP, general practitioner; ICD-10, International Classification of Diseases: Version 2010; IMD, Index of Multiple Deprivation; MI, myocardial infarction; VTE, venous thromboembolism
Data sources
In England, we used: (1) Primary care records managed by the general practice software provider TPP; (2) Office for National Statistics death register data; and (3) secondary care data from National Health Service (NHS) Digital’s Secondary Use Service data containing information on hospitalisations. All data were linked, stored and analysed securely using the OpenSAFELY platform, https://www.opensafely.org/, as part of the NHS England OpenSAFELY COVID-19 service. The population covers 43% of the UK population and is broadly representative of the English population.20 Pseudonymised data included coded diagnoses. All code is shared openly for review and re-use under the Massachusetts Institute of Technology (MIT) open licence (https://github.com/opensafely/covid_collateral_imd). Detailed pseudonymised patient data is potentially re-identifiable and therefore not shared.
In Denmark, all residents are assigned a unique personal identification number (the CPR number) at birth or immigration which makes it possible to link individual information among different data sources. We used data from: (1) the Danish National Patient Registry21 containing all inpatient discharge diagnoses from all Danish hospitals since 1977 and from emergency room and outpatient specialist clinic contacts since 1995 (diagnoses are coded according to the International Classification of Diseases (ICD) 8 from 1977 to 1993 and to the ICD 10 thereafter); (2) the Danish Civil Registration System including vital status and date of death for the entire Danish population; (3) socioeconomic registries maintained by Statistics Denmark including data on family and household socioeconomics, country of origin, educational level, employment status and income; and (4) The Danish Prescription Registry which has recorded all redeemed drug prescriptions from community pharmacies in Denmark since 1995.22
Study population
In England, the study population included adults aged 18 and over registered at a general practice using TPP software with at least 3 months of continuous registration with the practice prior to study entry. In Denmark, the study population included all adults aged 18 and over registered in the Danish Civil Registration System. In both countries, we excluded people with missing age, sex or deprivation information (defined in the exposures section) as this could indicate poor data quality. In England, people were also excluded if their household size was greater than 15 to exclude people living in institutions such as care homes who may have different hospital admission patterns. The measure of household size was a maximum of 15 in Denmark.
In both settings, the study period was 1 March 2018 and 31 December 2021. This was to give 2 years of data prior to the start of the pandemic for comparison. The study ended on 31 December 2021 as Danish data were only available up until this date. People entered the study at any time point during the study period as counts of outcomes were measured monthly. Follow-up continued until death or the end of the study period. In England, to measure denominators, people would also end follow-up if they deregistered with their general practitioner.
Study measures
Exposures
The primary exposure was socioeconomic deprivation which was measured by proxy. In England, deprivation was measured using quintiles of the patient-level Index of Multiple Deprivation (IMD) 2019.23 IMD is a lower super output area level (comprising 400 to 1200 people) which is a measure of relative deprivation based on a person’s postcode. The IMD score is based on indicators related to income, education, employment, health, crime, barriers to housing and services and living environment. We were unable to access an equivalent deprivation index in Denmark, so we used one aspect of deprivation; annual household income derived from the Danish Income Statistics Registry and divided into quintiles by year of age due to the variations in income by age (see online supplemental materials for details).24
Differences in outcomes by deprivation quintile were compared before and after the start of the pandemic restrictions. In England, pandemic restrictions were imposed on 23 March 2020,25 equivalent restrictions were imposed in Denmark on 11 March 2020.26 Since behaviours were likely to have changed prior to these dates, we used 1 March 2020 as the cut-off for both countries with time before this date referred to as the pre-pandemic period.
Outcomes
In both countries, we identified CVD-related hospital admissions based on recorded ICD-10 codes for myocardial infarction (MI), ischaemic or haemorrhagic stroke, heart failure and venous thromboembolism (VTE) assigned as the primary reason for admission.
Demographic and clinical characteristics
Demographic characteristics were identified at three time-points to describe the cohorts, these included age categorised into 20year age bands, sex and, in England only, rural-urban classification. In England, comorbidities were identified from primary care records. People with a Systematized Nomenclature of Medicine Clinical Terms (SNOMED CT) code for type 1 or type 2 diabetes mellitus on or before each time-point were considered to have diabetes. People with a SNOMED CT code for asthma in the 3 years prior to each time-point were considered to have asthma. People aged 40 years or over with a SNOMED CT code for chronic obstructive pulmonary disease (COPD) were considered to have COPD. In Denmark, where clinical diagnosis data from primary care are not available, definitions for diabetes, asthma and COPD were based on hospital discharge diagnoses as well as primary-care prescribing data from the Prescription Registry.
Statistical analysis
The characteristics of each cohort, overall and by deprivation quintile, were described on 1 March 2019, 2020 and 2021. On the first day of each month of follow-up (from March 2018 to December 2022, inclusive), the inclusion criteria were assessed and the denominator adult population who met the inclusion criteria was extracted from respective national databases. Each outcome was analysed separately and individuals with outcomes were counted once each month. Individuals with records for the same outcome in multiple months were included each time.
The percentage of people experiencing each outcome was calculated for every study month. We plotted the monthly percentage and the percentage change compared with the previous month (first derivative) by deprivation quintile. To estimate the absolute impact of the pandemic on each outcome, we used Poisson regression adjusted for an indicator of whether it was pre-pandemic or during the pandemic (binary), deprivation quintile, the interaction of both pandemic time and deprivation quintile. We further adjusted for population as an offset and time as a monthly continuous variable to estimate the average count of each outcome, by deprivation quintile, in the 22 months pre-pandemic (May 2018–February 2020) and the 22 months during the pandemic (March 2020–December 2021). We accounted for autocorrelation by including first-order lagged residuals. We used the estimated average counts from the Poisson model to generate rate differences in the numbers of each monthly outcome stratified by deprivation quintile.
We used Python V.3.9.12 for data management and Stata V.17 and R V.4.2.1 for analyses. Code for data management and analysis as well as codelists are archived online https://github.com/opensafely/covid_collateral_imd. All iterations of the prespecified study protocol are archived with version control https://github.com/opensafely/covid_collateral_imd/tree/main/docs.
Information governance and ethical approval
In England, NHS England is the data controller of the NHS England OpenSAFELY COVID-19 service; TPP is the data processor; all study authors using OpenSAFELY have the approval of NHS England.27 This implementation of OpenSAFELY is hosted within the TPP environment which is accredited to the ISO 27001 information security standard and is NHS IG Toolkit compliant.28 Further information can be found in the supplementary materials.
Results
On first March 2020, 16239
645 people in England and 4
491
336 people in Denmark met the inclusion criteria. The characteristics of the study populations were similar, though there were differences in the recorded prevalence of comorbidities. There was a higher recorded prevalence of diabetes in England (England: 7.9% vs Denmark: 6.5%) and a higher recorded prevalence of asthma and COPD in Denmark (table 2). Study population characteristics were similar in 2019 and 2021 (online supplemental material).
Table 2
Characteristic | England*N=16![]() ![]() | DenmarkN=4![]() ![]() | |
Age category | 18–40 years | 5![]() ![]() | 1![]() ![]() |
41–60 years | 5![]() ![]() | 1![]() ![]() | |
61–80 years | 4![]() ![]() | 1![]() ![]() | |
>80 years | 1![]() ![]() | 201![]() | |
Sex | Female | 8![]() ![]() | 2![]() ![]() |
Male | 8![]() ![]() | 2![]() ![]() | |
Deprivation† | 1 (most deprived) | 3![]() ![]() | 864![]() |
2 | 3![]() ![]() | 903![]() | |
3 | 3![]() ![]() | 906![]() | |
4 | 3![]() ![]() | 908![]() | |
5 (least deprived) | 3![]() ![]() | 908![]() | |
Rural-urban | Rural | 3![]() ![]() | – |
Urban | 12![]() ![]() | – | |
Diabetes mellitus¶ | 1![]() ![]() | 292![]() | |
Asthma‡ | 1![]() ![]() | 619![]() | |
COPD§ | 533![]() | 417![]() |
COPDchronic obstructive pulmonary disease
When stratified by deprivation quintile, in England people in the most deprived quintile were younger with 44% aged 18–40 years old versus 28.8% of the least deprived quintile. In Denmark, age was taken into account in the deprivation quintiles, therefore age distributions were similar across deprivation quintiles. In both countries, COPD and diabetes were more prevalent in the most deprived quintile (COPD: England: Most deprived: 4.6% vs least deprived: 2.3%, Denmark: Most deprived: 11.5% vs least deprived: 7.6%, diabetes: England: Most deprived: 9.5% vs least deprived: 6.7%, Denmark: Most deprived: 8.8%, least deprived: 4.3%) (tables3 tables3 4 4).
Table 3
Deprivation quintile | ||||||
1 (most deprived)N=3![]() ![]() | 2N=3![]() ![]() | 3N=3![]() ![]() | 4N=3![]() ![]() | 5 (least deprived)N=3![]() ![]() | ||
Age category | 18–40 years | 1 423![]() | 1 324![]() | 1 244![]() | 1 048![]() | 867![]() |
41–60 years | 1 051![]() | 1 058![]() | 1 153![]() | 1 103![]() | 1 023![]() | |
61–80 years | 613![]() | 734![]() | 931![]() | 929![]() | 886![]() | |
>80 years | 141![]() | 180![]() | 240![]() | 243![]() | 239![]() | |
Sex | Female | 1 604![]() | 1 654![]() | 1 816![]() | 1 704![]() | 1 551![]() |
Male | 1 626![]() | 1 642![]() | 1 754![]() | 1 620![]() | 1 464![]() | |
Rural-urban | Rural | 141![]() | 486![]() | 1 044![]() | 999![]() | 842![]() |
Urban | 3 089![]() | 2 811![]() | 2 526![]() | 2 325![]() | 2 174![]() | |
Diagnosis of diabetes mellitus | 305![]() | 281![]() | 281![]() | 241![]() | 199![]() | |
Diagnosis of asthma | 298![]() | 287![]() | 309![]() | 285![]() | 258![]() | |
Diagnosis of COPD | 147![]() | 116![]() | 110![]() | 90![]() | 68![]() |
COPDchronic obstructive pulmonary diseaseIMDIndex of Multiple Deprivation
Table 4
Deprivation quintile | ||||||
1 (most deprived)N=864![]() | 2N=903![]() | 3N=906![]() | 4N=908![]() | 5 (least deprived)N=908![]() | ||
Age category | 18–40 years | 292![]() | 324![]() | 327![]() | 328![]() | 328![]() |
41–60 years | 303![]() | 309![]() | 309![]() | 310![]() | 309![]() | |
61–80 years | 228![]() | 229![]() | 229![]() | 229![]() | 229![]() | |
>80 years | 40![]() | 40![]() | 40![]() | 40![]() | 40![]() | |
Sex | Female | 448![]() | 495![]() | 454![]() | 444![]() | 438![]() |
Male | 415![]() | 407![]() | 451![]() | 464![]() | 470![]() | |
Rural-urban | Rural | – | – | – | – | – |
Urban | – | – | – | – | – | |
Diagnosis of diabetes mellitus | 76![]() | 67![]() | 59![]() | 49![]() | 38![]() | |
Diagnosis of asthma | 113![]() | 126![]() | 128![]() | 127![]() | 123![]() | |
Diagnosis of COPD | 99![]() | 91![]() | 82![]() | 75![]() | 69![]() |
COPDchronic obstructive pulmonary disease
Hospital admissions overall
In both countries, there were similar proportions of the population admitted to hospital for each CVD outcome, although patterns by deprivation level differed between countries.
In England, across all outcomes, differences by deprivation level were small, although people in the most deprived quintile had the highest percentage of admissions for all outcomes. Across all outcomes, we observed a drop in admissions at the start of the pandemic and then a recovery to at least pre-pandemic levels by August 2020. This pattern did not vary by deprivation level. The largest decline in admissions was for heart failure. (Figure 1A and online supplemental figure S2), (online supplemental materials).
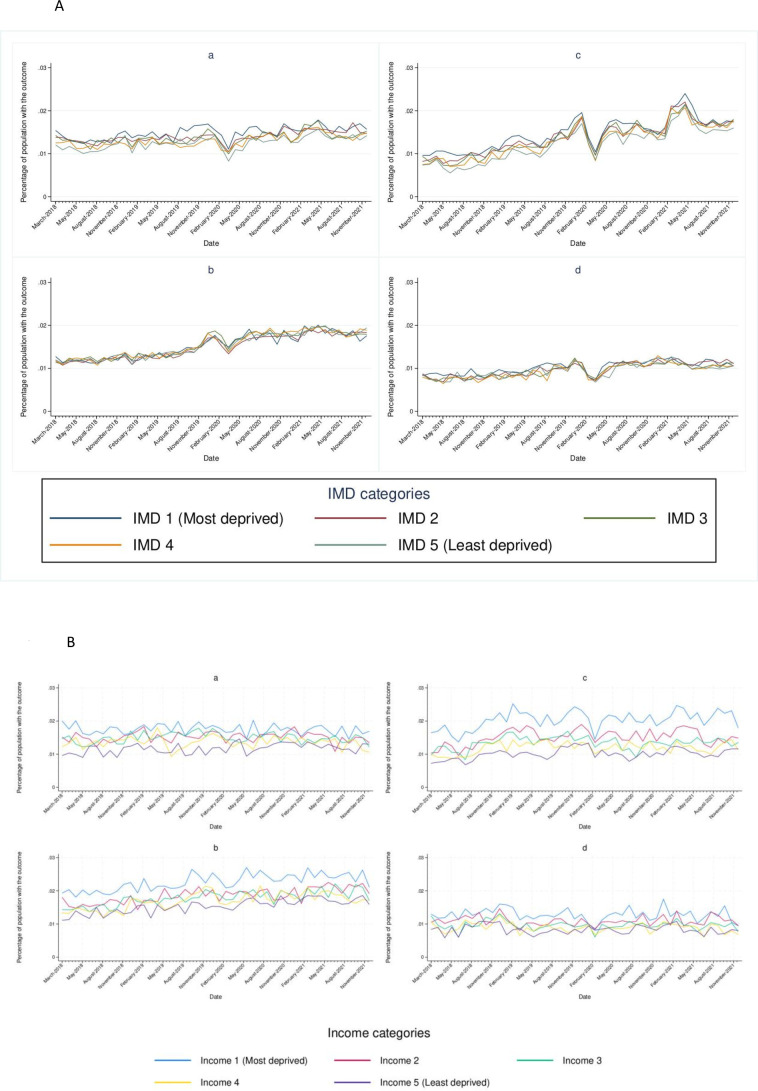
In Denmark, variation by deprivation quintile was more pronounced than in England for all outcomes. Overall, individuals in the most deprivation quintile had the highest proportion of admissions with admissions decreasing with decreasing deprivation. The biggest deprivation-related differences were seen for heart failure. The drop in admissions in March 2020 was greatest for individuals in the most deprived quintile with smaller drops seen in the less deprived quintiles. (Figure 1B and online supplemental figure S3), (online supplemental materials).
Hospitalisations during the pandemic
Poisson regression models indicated that, within deprivation quintiles, the number of admissions during the pandemic (1 March 2020 to 31 December 2021) was lower than expected and that there were small deprivation gradients in both England and Denmark.
England
In England, admissions for heart failure, MI and VTE were lower than expected with the gap between observed and expected largest for people in the most deprived quintile and smallest for those in the least deprived quintile. For heart failure admissions, the gap between observed and expected admissions was largest for individuals in the most deprived quintile and narrowed with decreasing deprivation. For people living in areas classified in the most deprived quintile, heart failure admissions were 17.8% lower than expected which in absolute terms translated to an estimated 2608 fewer admissions between 1 March 2020 and 31 December 2021. In the least deprived quintile, heart failure admissions were 9% lower than expected translating to an estimated 979 fewer admissions between 1 March 2020 and 31 December 2021. For MI, variation by deprivation level followed a similar pattern, although differences were smaller. For VTE, there were estimated to be fewer admissions than expected, though there was little variation by deprivation quintile. For stroke, there were slightly more admissions than expected, also with little variation by deprivation quintile (figure 2 and online supplemental table S4), (online supplemental materials).
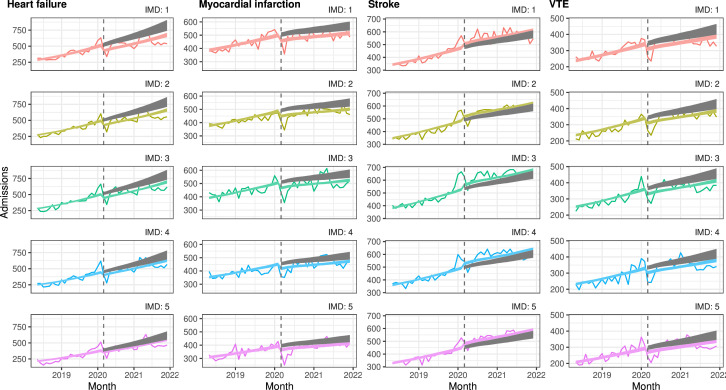
Denmark
In Denmark, admissions for MI were lower than expected. As a proportion of the number of expected admissions, the gap between observed and expected admissions over the pandemic period was largest for people in the least deprived quintile where admissions were 24% lower than expected compared with the most deprived quintile where admissions were 22% lower than expected. However, in absolute terms, differences were greatest in the most deprived quintile with 1013 fewer admissions during the pandemic compared with 619 fewer admissions in the least deprived quintile. For all other outcomes admissions during the pandemic were similar to pre-pandemic levels with little variation by deprivation level (figure 3 and online supplemental table S5), (online supplemental materials).
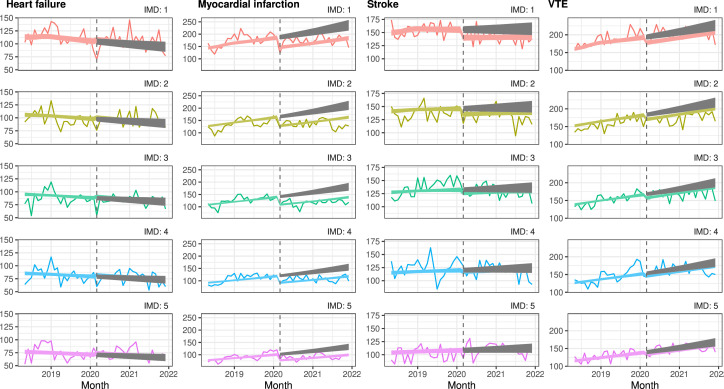
Discussion
In this descriptive observational study set in England and Denmark, we found that deprivation-level differences in cardiovascular hospitalisations were not exacerbated by the pandemic, with a few exceptions. In England, overall, there were fewer heart failure admissions during the pandemic than expected and reductions increased with increasing deprivation. In Denmark, there were fewer stroke and VTE admissions than expected during the pandemic in the most deprived quintile. In England, overall cardiovascular admissions increased over time whereas in Denmark admissions remained stable.
In both England and Denmark, people in the most deprived quintile had a higher prevalence of diabetes and COPD; in England, the mean age of people in the most deprived group was lower than for those in other deprivation quintiles. In England, we observed a deprivation gradient across our outcomes which was comparable to that observed for other health outcomes.3 However, differences by deprivation level were substantially more marked in Denmark. This could be due to the different measures of deprivation used. In Denmark, we used household-level income, while in England, we used IMD (a small area level measure based on the average deprivation level of an area assessed across a range of seven domains including income). IMD’s sensitivity and specificity to income deprivation is low,29 some people’s deprivation levels could have been misclassified. Assuming such misclassification was not differential, this could bias any differences towards the null which could explain the smaller differences between deprivation levels in England compared with Denmark.
Compared with the expected admissions, reductions in actual admissions between the pre-pandemic and pandemic periods were greater in England compared with Denmark which generally experienced little change. This is consistent with other studies of CVD admissions and specifically for non-ST-elevation acute coronary syndromes in 2020.7 14 Our study updates these findings to demonstrate that this pattern continued into 2021. There are potential explanations for this; the speed of response was quicker in Denmark which resulted in less stringent restrictions in Denmark compared with England (online supplemental materials). There were fewer COVID-19 deaths in Denmark compared with England.30 This may have meant cardiology services in hospitals remained similar during the pandemic as the health service may not have been so overwhelmed, whereas in England there was extreme disruption to primary care and secondary care cardiology services which would affect preventative care31 and health-seeking for acute CVD events. In addition, some heart failure services moved into the community in England which may have resulted in fewer hospital admissions.32
Although studies have investigated the impact of the pandemic on cardiovascular admissions,7 14 only a few studies have specifically investigated whether the pandemic impacted cardiovascular admissions by deprivation level.33,35 Two studies, in the USA and Catalonia, compared socioeconomic differences in heart failure admissions between 2019 and 2020 found that the impact of the pandemic was similar across income groups.30 33 These results are similar to our findings from Denmark where the impact of the pandemic was similar across deprivation groups in contrast to England where the reduction in heart failure admissions during the pandemic was larger in the most deprived. One study set in the USA found that the impact of the pandemic on stroke admissions was similar across income groups.34 This was consistent with our findings in England, whereas in Denmark there were slightly fewer admissions during the pandemic in the most deprived group but differences were small. As these studies are set in different countries, there could be many reasons for the observed differences in admissions.
Strengths and limitations
Our study was large, encompassing 20million people across two countries. Our study period ran until the end of 2021, longer than most previous studies (which largely ended in 2020),33,35 allowing us to describe the longer-term impacts of the pandemic, although we acknowledge there could still be impacts later than 2021. Our study design allowed us to compare the impact in two countries that both have a free-at-the-point-of-use health service but different responses to the pandemic. This is important for future pandemic preparedness and understanding the optimal response that does not further inequalities. However, an important limitation was that our measures of deprivation were different in the two countries with the measure in England capturing more aspects of deprivation than the Danish measure resulting in potential misclassification. Another limitation was that some information was not available in both countries, thus we could not examine cardiovascular mortality or ethnicity as this was unavailable in Denmark. Finally, since our results are descriptive, they help to generate hypotheses of potential mechanisms of differences observed but do not provide insight into the causes of any observed differences.
Conclusions
During the pandemic, we did not observe a worsening of the socioeconomic gradient on cardiovascular admissions in England and Denmark. There were some exceptions, most notably greater reductions in heart failure admissions in the most deprived groups in England. While it is positive that the pandemic has not worsened socioeconomic differences in cardiovascular admissions, further work is needed to understand the reasons for the differences seen in heart failure admissions in England.
Acknowledgements
Thanks to Dr Edward Parker for his help with output checking. This work uses data provided by patients and collected by the UK National Health Service (NHS) as part of their care and support. We are very grateful for all the support received from the TPP Technical Operations team throughout this work and for generous assistance from the information governance and database teams at NHS England and the NHS England Transformation Directorate. We have a publicly available website https://opensafely.org/ where we invite individuals to contact us regarding this study or the broader OpenSAFELY project.
Footnotes
Funding: This work was funded by the London School of Hygiene and Tropical Medicine COVID-19 Response Grant (reference: DONAT15912). This research was supported by the National Core Studies which is funded by UK Research and Innovation, the NIHR and the Health and Safety Executive (grant ref MC_PC_20059). In addition, the OpenSAFELY platform is supported by grants from the Wellcome Trust (222097/Z/20/Z); MRC (MR/V015757/1, MC_PC-20059, MR/W016729/1); NIHR (NIHR135559, COV-LT2-0073) and Health Data Research UK (HDRUK2021.000, 2021.0157). SVK acknowledges funding from a NRS Senior Clinical Fellowship (SCAF/15/02), the Medical Research Council (MC_UU_00022/2) and the Scottish Government Chief Scientist Office (SPHSU17). DMP was supported by a Medical Research Council fellowship (MR/W02148X/1). SL was supported by a Wellcome Trust Senior Research Fellowship in Clinical Science (205039/Z/16/Z). SL was also supported by Health Data Research UK (Grant number: LOND1) which is funded by the UK Medical Research Council, Engineering and Physical Sciences Research Council, Economic and Social Research Council, Department of Health and Social Care (England), Chief Scientist Office of the Scottish Government Health and Social Care Directorates, Health and Social Care Research and Development Division (Welsh Government), Public Health Agency (Northern Ireland), British Heart Foundation and Wellcome Trust. LAT is funded by an NIHR Research Professorship (NIHR302405). CW-G is supported by a Wellcome Career Development award (225868/Z/22/Z). AM acknowledges support from the Bennett Foundation, Wellcome Trust, NIHR Oxford Biomedical Research Centre, NIHR Applied Research Collaboration Oxford and Thames Valley, Mohn-Westlake Foundation. RM is supported by Barts Charity (MGU0504). The findings and conclusions in this report are those of the authors and do not necessarily represent the views of the funders. We are very grateful for all the support received from the TPP Technical Operations team throughout this work and for generous assistance from the information governance and database teams at National Health Service (NHS) England and the NHS England Transformation Directorate.We have a publicly available website https://opensafely.org/ where we invite individuals to contact us regarding this study or the broader OpenSAFELY project.
Prepub: Prepublication history and additional supplemental material for this paper are available online. To view these files, please visit the journal online (https://doi.org/10.1136/bmjopen-2024-088710).
Provenance and peer review: Not commissioned; externally peer reviewed.
Patient consent for publication: Not applicable.
Patient and public involvement: Patients and/or the public were not involved in the design, or conduct, or reporting, or dissemination plans of this research.
Data availability free text: Access to the underlying identifiable and potentially re-identifiable pseudonymised electronic health record data is tightly governed by various legislative and regulatory frameworks and restricted by best practice. The data in the National Health Service (NHS) England OpenSAFELY COVID-19 service is drawn from general practice data across England TPP is the data processor. TPP developers initiate an automated process to create pseudonymised records in the core OpenSAFELY database which are copies of key structured data tables in the identifiable records. These pseudonymised records are linked onto key external data resources that have also been pseudonymised via SHA-512 one-way hashing of NHS numbers using a shared salt. University of Oxford, Bennett Institute for Applied Data Science developers and PIs, who hold contracts with NHS England, have access to the OpenSAFELY pseudonymised data tables to develop the OpenSAFELY tools. These tools in turn enable researchers with OpenSAFELY data access agreements to write and execute code for data management and data analysis without direct access to the underlying raw pseudonymised patient data and to review the outputs of this code. All code for the full data management pipeline—from raw data to completed results for this analysis—and for the OpenSAFELY platform as a whole is available for review at github.com/OpenSAFELY.
Ethics approval: This study was approved by the Health Research Authority (REC reference 20/LO/0651) and by the London School of Hygiene and Tropical Medicine Ethics Board (reference 21863). In Denmark, registry studies do not require ethical approval, but are reported to the Data Protection Board (Case number: 2016-051- 000001/1793).
References
Review Process File
Articles from BMJ Open are provided here courtesy of BMJ Publishing Group
Full text links
Read article at publisher's site: https://doi.org/10.1136/bmjopen-2024-088710
Similar Articles
To arrive at the top five similar articles we use a word-weighted algorithm to compare words from the Title and Abstract of each citation.
Trends in weight gain recorded in English primary care before and during the Coronavirus-19 pandemic: An observational cohort study using the OpenSAFELY platform.
PLoS Med, 21(6):e1004398, 24 Jun 2024
Cited by: 3 articles | PMID: 38913709 | PMCID: PMC11249215
Excess years of life lost to COVID-19 and other causes of death by sex, neighbourhood deprivation, and region in England and Wales during 2020: A registry-based study.
PLoS Med, 19(2):e1003904, 15 Feb 2022
Cited by: 22 articles | PMID: 35167587 | PMCID: PMC8846534
Ethnic differences in the indirect effects of the COVID-19 pandemic on clinical monitoring and hospitalisations for non-COVID conditions in England: a population-based, observational cohort study using the OpenSAFELY platform.
EClinicalMedicine, 61:102077, 29 Jun 2023
Cited by: 1 article | PMID: 37434746 | PMCID: PMC10331810
Medicines associated with dependence or withdrawal: a mixed-methods public health review and national database study in England.
Lancet Psychiatry, 6(11):935-950, 03 Oct 2019
Cited by: 40 articles | PMID: 31588045 | PMCID: PMC7029276
Review Free full text in Europe PMC
Funding
Funders who supported this work.
Chief Scientist Office (1)
Inequalities in Health
Professor Alastair Leyland, University of Glasgow
Grant ID: SPHSU17
London School of Hygiene and Tropical Medicine (1)
Grant ID: DONAT15912
National Institute for Health Research (NIHR) (2)
INTEGRATE: Using routinely-collected healthcare data to inform clinical guidance and improve population health
Prof Laurie Tomlinson, London School of Hygiene and Tropical Medicine
Grant ID: NIHR302405
Grant ID: NIHR135559
UK Research and Innovation (1)
Grant ID: MC_PC-20059
Wellcome Trust (3)
Grant ID: 20059
Using linked electronic health data to improve eczema diagnosis and outcomes
Professor Sinead Langan, London School of Hygiene & Tropical Medicine
Grant ID: 205039/Z/16/Z
Grant ID: 222097/Z/20/Z