Abstract
Free full text

Accounting for cellular-level variation in lysis: implications for virus–host dynamics
ABSTRACT
Viral impacts on microbial populations depend on interaction phenotypes—including viral traits spanning the adsorption rate, latent period, and burst size. The latent period is a key viral trait in lytic infections. Defined as the time from viral adsorption to viral progeny release, the latent period of bacteriophage is conventionally inferred via one-step growth curves in which the accumulation of free virus is measured over time in a population of infected cells. Developed more than 80 years ago, one-step growth curves do not account for cellular-level variability in the timing of lysis, potentially biasing inference of viral traits. Here, we use nonlinear dynamical models to understand how individual-level variation of the latent period impacts virus–host dynamics. Our modeling approach shows that inference of the latent period via one-step growth curves is systematically biased—generating estimates of shorter latent periods than the underlying population-level mean. The bias arises because variability in lysis timing at the cellular level leads to a fraction of early burst events, which are interpreted, artefactually, as an earlier mean time of viral release. We develop a computational framework to estimate latent period variability from joint measurements of host and free virus populations. Our computational framework recovers both the mean and variance of the latent period within simulated infections including realistic measurement noise. This work suggests that reframing the latent period as a distribution to account for variability in the population will improve the study of viral traits and their role in shaping microbial populations.
IMPORTANCE
Quantifying viral traits—including the adsorption rate, burst size, and latent period—is critical to characterize viral infection dynamics and develop predictive models of viral impacts across scales from cells to ecosystems. Here, we revisit the gold standard of viral trait estimation—the one-step growth curve—to assess the extent to which assumptions at the core of viral infection dynamics lead to ongoing and systematic biases in inferences of viral traits. We show that latent period estimates obtained via one-step growth curves systematically underestimate the mean latent period and, in turn, overestimate the rate of viral killing at population scales. By explicitly incorporating trait variability into a dynamical inference framework that leverages both virus and host time series, we provide a practical route to improve estimates of the mean and variance of viral traits across diverse virus–microbe systems.
INTRODUCTION
Viruses have a profound impact on microbial populations through the modulation of population dynamics, eco-evolutionary dynamics, community structure, and ecosystem function (1,–6). Our understanding of the ecological interactions between viruses and microbes depends on the study of viral life history traits that characterize the viral life cycle, such as the adsorption rate, latent period (LP), and burst size (7, 8). During lytic infections, viral adsorption to the host cell is followed by synthesis where the host machinery produces new viral particles. Once assembled, viral particles burst from the cell and can infect new target cells. The time to complete a viral cycle, from virus adsorption to cell burst, is termed the latent period (7). The latent period varies across taxa (8) and can be influenced by environmental factors such as resource availability and host physiological state (9,–12). This key trait affects the population dynamics of virus–microbe pairs, with strong consequences for virus fitness and host population fate (13, 14).
The one-step growth curve, introduced by Ellis and Delbrück in 1939 (15), is an experimental setup used to infer key viral traits from monitoring viral infection of a microbial population within a single round of infection. Typically, the one-step growth curve has the following experimental protocol. First, cells are inoculated with virus under conditions that allow for adsorption to take place without host reproduction or viral replication. Then, unadsorbed viruses are removed to allow for a single round of infection. Finally, free viruses are measured at several time points. From these observations, the latent period is typically reported as the time of first visible burst (Fig. 1), i.e., when free virus concentration first increases (15, 16). Alternatively, the midpoint of the rise might be used to report the latent period (8, 12). Despite widespread use, the first visible burst can lead to biases in estimates of the average latent period in the presence of cellular-level variability in lysis, as it captures the combined influence of early adsorption with early cell lysis events (15, 17,–20).
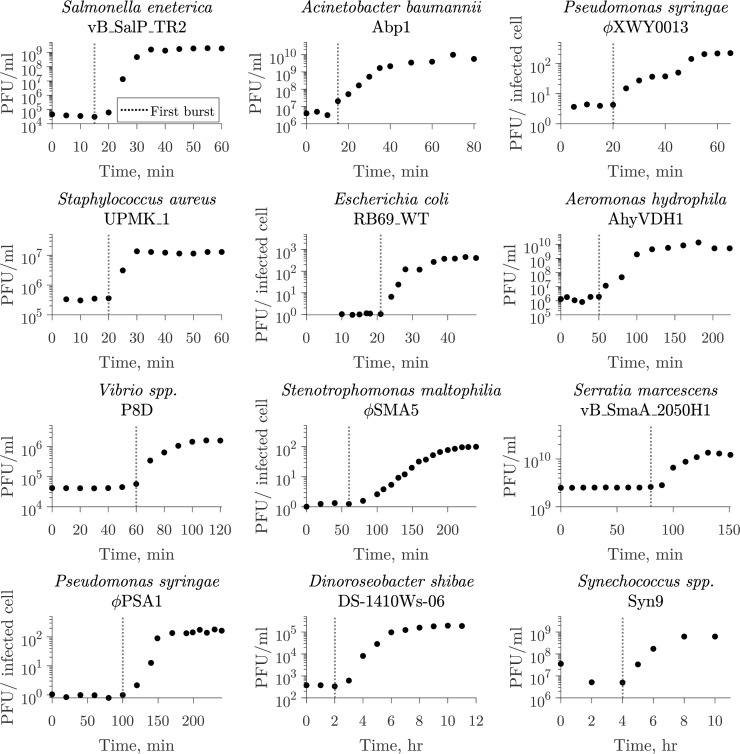
The one-step growth curve protocol for inferring lysis timing. The one-step growth curve is used to estimate burst size and latent period by observing a single round of infection. In such experiments, virus is added to a microbial population and left to adsorb until the majority of the cells are infected. The population is diluted or viruses are removed to prevent the occurrence of new infections. From this point, plaque-forming units (PFUs) are measured over time. The time of first visible burst, when PFU counts start to increase due to cell lysis and viral progeny release, is commonly reported as the latent period (18, 21). The latent period of multiple virus–microbe pairs has been characterized using this method. Here, we show examples of different microbe–virus pairs where the time of first burst was reported as the latent period ranging from 15 min to 4 h. The dotted line represents the reported value in the corresponding study. The list of data sources (10, 22,–32) is available in Table S1.
Indeed, it is already well understood that even when an infected cell population is perfectly synchronized, i.e., ensuring adsorption synchronization, individual cells may burst at different times. This variation could be due to differences in the host phenotype at the time of infection, e.g., physiological states (12, 17, 19, 33, 34), or due to stochasticity at the cellular level (20, 35,–37). For instance, variability in the latent period of genetically identical populations has been found in multiple strains of
Here, we explore the effect of latent period variability on microbe–virus dynamics and its impact on current approaches to infer the latent period in practice. Our findings reveal that the presence of latent period variability results in systematic biases that can lead to the underestimation of latent periods in the one-step growth curve used for viral trait estimation. Hence, established methods infer a more rapid lysis process from population measurements than what is likely to occur when accounting for cellular-level heterogeneity. Instead, by integrating cellular-level heterogeneity in lysis timing in an explicit virus–microbe infection model, we are able to estimate both the mean and variability of lysis timing from population-level data. As we show, expanding current protocols to include measurements of both virus and host abundances via the use of “multi-cycle response curves” provides a route to improved estimates of viral traits and their variability, essential to quantify the impact of viruses on microbial populations in both ecological and therapeutic contexts.
RESULTS
Latent period variability impacts one-step growth curves
We use a coupled system of nonlinear differential equations to model interactions between a population of microbial cells and a lytically infecting viral population (Materials and Methods; Fig. S1). The model considers a microbial population where infected cells burst at different times following an Erlang distribution described by the mean latent period and the coefficient of variation (CV). We perform simulations of one-step growth curves to elucidate the impact of individual-level variation on conventional methods of viral trait estimation (Fig. 2). As described in Materials and Methods, we replicate a classic one-step growth curve protocol where phage is added to a bacterial population in the exponential growth at a multiplicity of infection (MOI) of 0.01, the system is diluted to reduce new infections, and the accumulation of free viruses is measured over time (Fig. 2A).
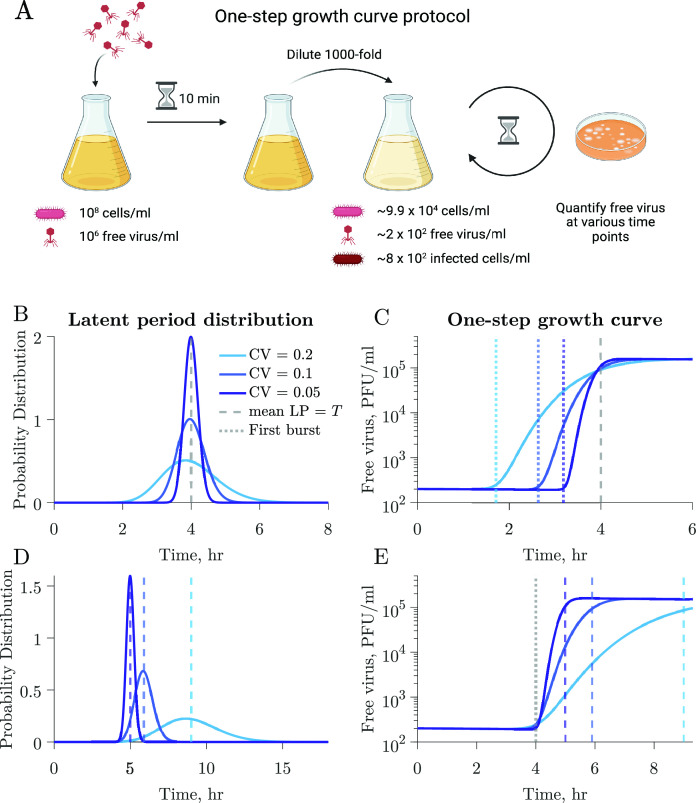
The latent period distribution connects individual variation to population-level microbe–virus dynamics. (A) Using our microbe–virus dynamical model, we simulated one-step growth curves following standard protocols, as described in Materials and Methods. (B) Populations in each simulation have the same traits (Table 1), i.e., microbial growth rate, carrying capacity, adsorption rate, burst size, and LP mean (4 h, dashed gray line), and only differ in the CV of the latent period distribution, which varies across simulations with larger CV depicted in lighter shades of blue. (C) The one-step growth curves for the different simulations show different free virus dynamics. The standard estimates of the latent period, as measured by the time of first burst (dotted lines), vary across simulations. Latent period variability affects the one-step growth curves for otherwise identical populations. (D) In this set of simulations, the populations have the same traits (Table 1), i.e., microbial growth rate, carrying capacity, adsorption rate, and burst size, but differ in latent period distributions with varying latent period mean and CV. (E) Systems with visibly different latent period distributions can result in similar first burst estimates derived from one-step growth curves.
Figure 2B and C shows three different simulations in which all host and viral traits are consistent across scenarios including the average latent period, except for the latent period CV, which varies across 0.05, 0.1, and 0.2 (see Table 1 for complete specification of parameter values). The resulting one-step growth curves are visibly different between simulations. Furthermore, the time of first visible burst, which is conventionally used as a proxy for the average latent period (Fig. 1), varies greatly for the different simulations despite them having the same mean latent period. This also applies to other features of the curve, e.g., the midpoint of the rise. Specifically, in wider distributions (larger CV), the first visible burst occurs earlier because of a higher proportion of cells lysing at shorter times. Hence, the time of first burst leads to a biased estimate of shorter latent periods than the true, underlying latent period for all simulated distributions, including distributions with small CV. These results indicate that reporting the time of first burst as the latent period is a misleading conflation: doing so systematically underestimates the population mean, and this bias worsens with increasing cellular-level variability.
We further illustrate the influence of latent period variability on key features of the one-step growth curve by comparing three simulations with varying latent period distributions, each characterized by distinct mean and CV (Fig. 2D). Host and viral traits, excluding latent period-associated traits, remain the same across all simulations (see Table 1 for parameter values). Despite distinct underlying latent period distributions, including differences in the mean latent period, the simulated one-step growth curves consistently result in the same time of first burst (Fig. 2E, dotted line).
Based on these observations, we revisited data from references 13, 35 to compare the differences between population and cellular-level measurements of lysis timing. In these studies, the lysis time, i.e., the time to lysis after prophage induction, was measured using population-scale turbidity assays (13) and single-cell lysis event microscopy observations (35) of isogenic
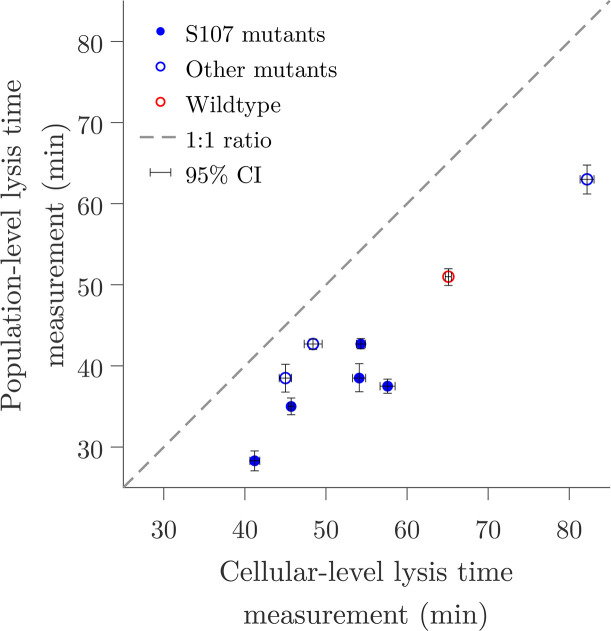
Population-level measurements systematically underestimate the lysis time in
Free virus and host temporal dynamics provide better resolution than one-step growth curves to discern between latent period distributions
Next, we set out to explore the link between viral population dynamics arising from one-step growth curve protocols and the shape of the underlying latent period distribution. We systematically varied both the mean and CV of the latent period distribution. In each case, we simulated the one-step growth curve protocol and compared the resulting viral population dynamics to a reference (i.e., the true but unknown mean and CV of the virus–host pair). For example, given a reference latent period of 10 h and CV of 0.25, we calculated the sum of relative errors in viral population dynamics for each combination of latent period and CV (Fig. 4A). Growth curves resulting from combinations featuring a higher mean and larger CV will resemble those with a smaller mean and smaller CV (Fig. 4A). This, coupled with experimental uncertainty, results in a space of latent period distributions difficult to distinguish from each other when comparing one-step growth curves (Fig. 4B and C). These results suggest that one-step growth curve protocols lack the resolution needed for accurate characterization of latent period distributions.
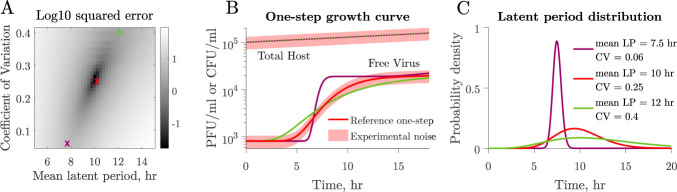
Latent period distribution identifiability when using one-step growth curves. (A) Simulated one-step growth curves (see Materials and Methods) obtained from microbe–virus pairs with different underlying latent period distributions can resemble each other. When we compare a reference curve (red cross) to curves obtained from systems with different distributions, we observe that curves that resemble the reference the most are found along an ascending slope. These correspond to combinations of larger mean, larger CV (green cross) or smaller mean, smaller CV (purple cross). (B) Example of different combinations of latent period mean and CV that produce similar curves. One-step growth curves become harder to differentiate when taking experimental noise into account. Changes in host density, represented by colony-forming units per volume unit, resulting from viral lysis in a single cycle of infection are insignificant owing to the low multiplicity of infection (MOI) utilized in protocols. (C) Corresponding latent period distributions for panels A and B. All nonlatent period traits, i.e., microbial growth rate, carrying capacity, adsorption rate, and burst size, are the same across simulations (Table 2).
As an alternative, we propose the use of a “multi-cycle response curve” protocol. In this protocol, phages are mixed with a susceptible bacterial population at low MOI. Next, both free virus and host cells are quantified at various time points after phage addition (Fig. 5). In contrast to the one-step growth curve simulations, there is neither an incubation period nor a subsequent dilution. While some combinations of the mean latent period and CV result in similar viral population dynamics (Fig. 5B), the corresponding host population dynamics for these combinations are markedly different from each other (Fig. 5C). These observations imply that population-level temporal dynamics data of hosts and viruses have the potential to be used to predict individual-level variation in viral traits.
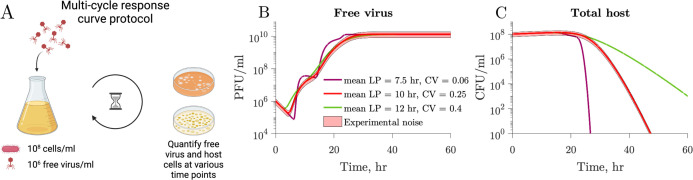
Multi-cycle response curves provide a better alternative for latent period distribution identification. (A) We simulate an experimental protocol where infecting viral particles are added to a microbial population at MOI 0.01 (see Materials and Methods). Free virus and host cells are quantified at multiple time points after infection. Unlike one-step growth curve protocols, there is no removal of free viral particles after an incubation period. The simulated time captures multiple rounds of infection. (B) Free virus dynamics of three simulations with different latent period distributions but otherwise the same viral and host parameters (Table 2). Note that multiple rounds of infection are observed. (C) Corresponding host dynamics for the three simulations. While the one-step growth curves for the same parameters are highly similar (Fig. 4), the multi-cycle response curves differ from each other.
Inferring latent period distributions from temporal dynamics
We developed a computational framework with the goal of inferring latent period distributions from multi-cycle response curves (Fig. 6A). This framework involves fitting host and viral data to our population model of lytic infections (equation 1), achieved by selecting combinations of model parameters that minimize the error between observed data and the model. The framework is comprised of two steps: (i) using a likelihood function to narrow the initial search space of parameters and (ii) implementing a Bayesian Markov chain Monte Carlo (MCMC) search. The Bayesian MCMC search is guided by prior distributions informed by the likelihood function used in the initial step (see Materials and Methods for further details). Using this approach, we can estimate host traits, i.e., growth rate (
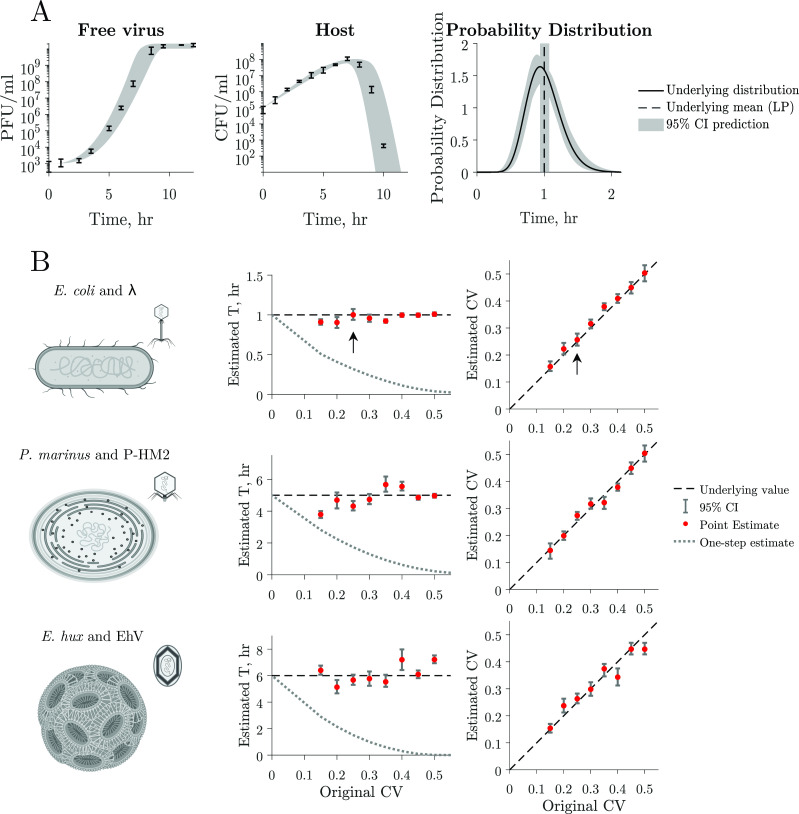
Latent period distribution estimated from simulated multi-cycle response curves. (A) Estimation of the latent period distribution of a virus–microbe system by fitting a nonlinear dynamical model to the simulated time series with added noise. In this example, we estimate the latent period distribution of free virus and host time series simulated data with added noise (left and middle panels). Thus, we have a priori knowledge of the underlying latent period distribution (black curve) of the system to evaluate our framework. We can accurately estimate the original mean (mean LP = 1 h, black line) and CV = 0.25 and therefore estimate the original latent period distribution (compare black curve and confidence interval estimations). Gray shaded region indicates 95% confidence intervals. (B) We use parameter values that capture the interactions of three biologically relevant systems: Escherichia coli and
As our main interest is in inferring the latent period distribution, we test the accuracy of our approach at predicting these distributions using simulated data. Using simulated data allows us to have a priori knowledge of the underlying latent period distribution. We use our nonlinear dynamical model to generate host and viral time series. We sample the time series to obtain 10 data points equally spaced in time, consistent with current experimental standards, and add 30% normally distributed noise to mirror uncertainty in experimental measurements (Materials and Methods). To test our framework on a variety of biologically relevant data, we generate dynamics using three different parameter sets that represent (i) E. coli and
Consider first the viral population dynamics, host population dynamics, and latent period distribution associated with the E. coli and
The latent period mean and CV values predicted using our framework are close to those with which data were generated for all simulations and all parameter sets for E. coli and
DISCUSSION
Here, we explored how individual-level variation in the latent period impacts virus–host dynamics and its consequences on the inference of viral life history traits, including the mean and variability of the latent period distribution. To do so, we developed a model of virus–host interactions that incorporates latent period variability. We show that latent period estimates obtained from current viral life history trait estimation protocols—specifically the one-step growth curve—can deviate systematically and substantially from the actual mean latent period. The rationale is that variability in the latent period can lead to a subpopulation of infected cells lysing early, which is then misinterpreted as a shorter mean latent period for the population as a whole. Instead, we propose using host and viral dynamics to infer the population latent period via a “multi-cycle response curve” protocol. By fitting nonlinear population models, we show that it is possible to recover unbiased estimates of the mean and variability of latent periods along with accurate estimates of other viral and cellular life history traits.
The present approach extends efforts leveraging model fitting to characterize viral life history traits (12, 39,–42). Similar to the modeling structure proposed here, other models of virus–host dynamics have incorporated explicit treatment of multiple infection compartments as an approximation to the delay between infection and lysis (12, 41, 43). In these cases, the model interpretation does not link variability in the latent period with impacts on the estimate of the latent period itself. Inaccurate estimates of viral latent periods can lead to incorrect assumptions about virus turnover, potentially leading to overestimates of viral-induced mortality at population scales. Instead, accurate and unbiased estimates of viral traits are required to inform ecological and ecosystem models (7, 42, 44, 45).
The use of multi-cycle response curves leverages information in both viral and host population time series to connect cellular-level processes to population-level dynamics. In doing so, early increases in virus population dynamics are linked to the early components of variable latent period distributions rather than an indication of a systematically faster lytic process. This feature is likely relevant across virus–host systems. However, more work is needed to identify the mechanisms underlying variability, including those that impact other viral traits. For example, while correlations between latent period and burst size have been observed, with phage strains with longer latent periods having larger burst sizes (13), recent studies suggest that variation in (induced) lysis time does not contribute to observed burst size variability (46, 47). Caution in the interpretation of multi-cycle response curves is required, as viral traits often depend on the host growth rate, which can vary in experimental timescales (12). In addition, the current method could be extended in multiple ways, e.g., to include virion decay on the timescale of the protocol, which may be relevant for extending the method in situ and/or in vivo where reduced viral stability is expected, or to include coinfection of a single cell by multiple viruses.
In summary, for more than 80 years, the one-step growth curve has been the standard to characterize essential viral life history traits: the latent period and burst size (15). As we have shown, this protocol comes with a significant caveat: leading to estimates of more rapid lysis when neglecting the impacts of latent period variability. Our work suggests that reframing the latent period as a distribution is essential to improve viral life history trait estimates and connect viral traits to population-level dynamics. In practice, the use of multi-cycle response curves leverages host and viral time series to reduce biases in trait inferences. Moving forward, we are optimistic that embedding measurements of both viral and host time series in the context of mathematical models with explicit treatment of variability can be used to improve inference in experiments and estimates of viral traits in environmental and therapeutic contexts.
MATERIALS AND METHODS
Latent period distribution incorporated into a nonlinear population model of lytic viral infections
We use a coupled system of nonlinear differential equations to model virus–host dynamics, including susceptible cells (
To incorporate variability in the latent period, we assume that before entering the actively infected stage (
where
The number of
Model implementation
One-step growth curve
When using our model to simulate one-step growth curves, we replicate the protocol in experimental one-step growth curves by simulating the addition of phage to a microbial population in the exponential growth phase. We initialize the system with
TABLE 1
Model parameter values for one-step growth curvesa
Value | Unit | |
---|---|---|
Parameter | ||
![]() | 0.1 | h−1 |
![]() | 1 × 109 | CFU/mL |
![]() | 1 × 10−7 | mL/(CFU × h) |
![]() | 200 | |
![]() | b | h−1 |
![]() | b | |
Initial condition | ||
![]() | 108 | CFU/mL |
![]() | 106 | PFU/mL |
Multi-cycle response curve
In our simulation of multi-cycle response curves, we initialize the system with
TABLE 2
Model parameter values for one-step growth curves and multi-cycle response curvesa
Value | Unit | |
---|---|---|
Parameter | ||
![]() | 0.025 | h−1 |
![]() | 1 × 109 | CFU/mL |
![]() | 4 × 10−9 | mL/(CFU × h) |
![]() | 100 | |
![]() | b | h−1 |
![]() | b | |
Initial condition | ||
![]() | 108 | CFU/mL |
![]() | 106 | PFU/mL |
To simulate the dynamics of a variety of host and viral systems, we use reference parameter values for three different microbe–virus pairs: E. coli and
TABLE 3
Model parameter values for three distinct microbe–virus pairsa
Value for: | Unit | |||
---|---|---|---|---|
E. coli and λ | P. marinus and P-HM2 cyanophage | E. hux and EhV | ||
Parameter | ||||
![]() | 1.2 | 0.035 | 0.015 (49) | h−1 |
![]() | 1 × 108 | 3 × 109 | 1 × 109 | CFU/mL |
![]() | 1 × 10−8 | 9.3 × 10−10 | 1.5 × 10−7 (50) | mL/(CFU × h) |
![]() | 200 | 40 | 800 (51) | |
![]() | 1 | 0.2 | 0.1667 (51) | h−1 |
Initial condition | ||||
![]() | 105 | 108 | 105 | CFU/mL |
![]() | 103 | 106 | 103 | PFU/mL |
Latent period distribution inference
We use a computational framework to estimate latent period distributions from host and free virus temporal dynamics data by fitting to our population model of lytic infections (equation 1). Our framework predicts all host and viral-associated parameters comprised in the model, i.e., host growth rate (
In step i, rough parameter ranges are found using a grid search for the maximum likelihood parameter combination from a range of biologically plausible parameter values (52). In step ii, we implement MCMC using the Turing package in Julia (53) and inform prior distributions using the predictions obtained in step i. The resulting posteriors are then used as priors for a second round of MCMC. Details on the prior distributions and convergence analysis can be found in the supplemental information. We obtain 95% confidence intervals by sampling the MCMC posterior distributions. We test our framework by fitting data generated with added noise, as explained above, for which the underlying latent period distribution is known.
ACKNOWLEDGMENTS
We thank Stephen Beckett and Adriana Lucia-Sanz for the code review. Illustrations were created with BioRender.com.
This research was supported in part through research cyberinfrastructure resources and services provided by the Partnership for an Advanced Computing Environment (PACE) at the Georgia Institute of Technology, Atlanta, GA, USA. The research effort was enabled by support from grants from the Simons Foundation (J.S.W., Award ID 722153), the Blaise Pascal Chair of the Ile-de-France region (J.S.W.), the Chateaubriand Fellowship (M.D.-M.), and the National Council of Humanities, Science and Technology of Mexico (M.D.-M.). Funding sources had no role or influence on the study design, analysis, interpretation, or submission.
Footnotes
DATA AVAILABILITY
We implement the model (equation 1) in Julia v1.7.2 (54) using the Differential Equations package v7.1 (55) and Matlab R2023a (56). All code for simulations and plotting is available at https://github.com/WeitzGroup/LatentPeriodVariability and archived at https://doi.org/10.5281/zenodo.11085440.
SUPPLEMENTAL MATERIAL
The following material is available online at https://doi.org/10.1128/mbio.01376-24.
Supplemental Information
mbio.01376-24-s0001.pdf:Supplemental text, tables, and figures.
ASM does not own the copyrights to Supplemental Material that may be linked to, or accessed through, an article. The authors have granted ASM a non-exclusive, world-wide license to publish the Supplemental Material files. Please contact the corresponding author directly for reuse.
REFERENCES
Articles from mBio are provided here courtesy of American Society for Microbiology (ASM)
Full text links
Read article at publisher's site: https://doi.org/10.1128/mbio.01376-24
Citations & impact
Impact metrics
Alternative metrics

Discover the attention surrounding your research
https://www.altmetric.com/details/165515887
Article citations
A nanoluciferase-encoded bacteriophage illuminates viral infection dynamics of Pseudomonas aeruginosa cells.
ISME Commun, 4(1):ycae105, 01 Jan 2024
Cited by: 1 article | PMID: 39296778 | PMCID: PMC11409504
Similar Articles
To arrive at the top five similar articles we use a word-weighted algorithm to compare words from the Title and Abstract of each citation.
Evolutionary comparison between viral lysis rate and latent period.
J Theor Biol, 345:32-42, 17 Dec 2013
Cited by: 17 articles | PMID: 24361326
A nanoluciferase-encoded bacteriophage illuminates viral infection dynamics of Pseudomonas aeruginosa cells.
ISME Commun, 4(1):ycae105, 01 Jan 2024
Cited by: 1 article | PMID: 39296778 | PMCID: PMC11409504
Host Traits Drive Viral Life Histories across Phytoplankton Viruses.
Am Nat, 191(5):566-581, 16 Mar 2018
Cited by: 13 articles | PMID: 29693441
Look Who's Talking: T-Even Phage Lysis Inhibition, the Granddaddy of Virus-Virus Intercellular Communication Research.
Viruses, 11(10):E951, 16 Oct 2019
Cited by: 28 articles | PMID: 31623057 | PMCID: PMC6832632
Review Free full text in Europe PMC
Funding
Funders who supported this work.
Blaise Pascal Chaire of the Ile-de-France Region
Chateaubriand Fellowship Program
Consejo Nacional de Humanidades Ciencias y Tecnologías
Consejo Nacional de Humanidades Ciencias y Tecnologías (CONAHCYT)
Simons Foundation (1)
Grant ID: 722153
Simons Foundation (SF) (1)
Grant ID: 722153