Abstract
Free full text

Progress in genetics of type 2 diabetes and diabetic complications
Abstract
Type 2 diabetes results from a complex interaction between genetic and environmental factors. Precision medicine for type 2 diabetes using genetic data is expected to predict the risk of developing diabetes and complications and to predict the effects of medications and life‐style intervention more accurately for individuals. Genome‐wide association studies (GWAS) have been conducted in European and Asian populations and new genetic loci have been identified that modulate the risk of developing type 2 diabetes. Novel loci were discovered by GWAS in diabetic complications with increasing sample sizes. Large‐scale genome‐wide association analysis and polygenic risk scores using biobank information is making it possible to predict the development of type 2 diabetes. In the ADVANCE clinical trial of type 2 diabetes, a multi‐polygenic risk score was useful to predict diabetic complications and their response to treatment. Proteomics and metabolomics studies have been conducted and have revealed the associations between type 2 diabetes and inflammatory signals and amino acid synthesis. Using multi‐omics analysis, comprehensive molecular mechanisms have been elucidated to guide the development of targeted therapy for type 2 diabetes and diabetic complications.
Abstract
Genome‐wide association studies (GWAS) were conducted and new genetic loci were identified that modulate the risk of developing type 2 diabetes and diabetic complications. The polygenic risk score and multi‐omics analysis have begun to decipher the molecular mechanisms of diabetes and its complications.
INTRODUCTION
Diabetes is rapidly increasing due to environmental factors such as changes in diet and decreased exercise. 1 Type 2 diabetes and its complications are associated with premature mortality, causing a public health burden worldwide. 2 The impact of type 2 diabetes on communicable disease has been highlighted. People with type 2 diabetes are at an increased risk of severe COVID‐19 infection.
In type 2 diabetes, both genetic and environmental factors affect the onset of the disease, and although the proportion of involvement of these factors varies from case to case, it is thought to develop when the sum of the effects of both factors exceeds a certain threshold. Type 2 diabetes is caused by a combination of genetic factors that influence insulin secretion and insulin resistance, lifestyle such as obesity, overeating, and lack of exercise. It is estimated from twin family studies that the heritability of type 2 diabetic complications is about 40%. 3 , 4 , 5 By elucidating the molecular mechanisms of genetic factors, we could optimize prevention and treatment, and achieve precision medicine tailored to the individual.
In 2003, about 3billion base pairs of the human genome were sequenced. Comprehensive analysis methods for sequences using microarrays of single nucleotide polymorphisms have been developed, and disease susceptibility genetic loci have been identified by genome‐wide association study (GWAS) on age‐related macular degeneration first in 2005.
6
GWAS is a method of testing the association between the allele frequency of a single‐nucleotide polymorphism genotype and disease. Highly reproducible results have been obtained by analyzing a sufficient number of cases and confirming significant differences.
7
,
8
,
9
,
10
,
11
,
12
Recently, the number of participants for GWAS has exceeded 1 million, and more disease susceptibility genes have been identified using large‐scale meta‐analysis.
13
,
14
,
15
,
16
,
17
,
18
,
19
,
20
,
21
,
22
,
23
,
24
,
25
,
26
,
27
,
28
,
29
,
30
,
31
,
32
,
33
,
34
,
35
,
36
Genome‐wide level associations with P‐values under 5
×
10−8 have been observed in more than 600 genetic loci for diabetes, more than 1,100 loci for obesity.
37
.
In this review, we summarize genetic studies in type 2 diabetes and diabetic complications. We also consider how the polygenic risk score has begun to decipher the mechanisms related to diabetes and its complications. Finally, we explore the multi‐omics analysis that enhances genotype information into biology and facilitates new avenues for treatment.
DEVELOPMENT OF GWAS FOR TYPE 2 DIABETES
Genome‐wide association study has shown statistically that the frequency of disease susceptibility gene polymorphisms differs between patient and control groups. It has been suggested that type 2 diabetes differs in genetic factors and pathophysiology depending on the ethnic population. While Europeans develop type 2 diabetes only after becoming obese, East Asians develop diabetes at a lower weight than Europeans due to the different level of insulin secretion. 38 , 39 To identify ethnically characteristic type 2 diabetes genes, GWAS using large‐scale, high‐density SNPs in each ethnic population is required.
An association study on chromosome 10q, which has been mapped as the type 2 diabetes locus, identified variants in TCF7L2 (T cell factor like 2). 40 In 2007, the first GWAS for type 2 diabetes included thousands of participants and identified 10 genomic loci. 7 , 8 , 9 , 41 , 42 Now the GWAS of type 2 diabetes, mainly from European and Asian populations, has identified more than 600 disease‐susceptible loci (Figure 1).
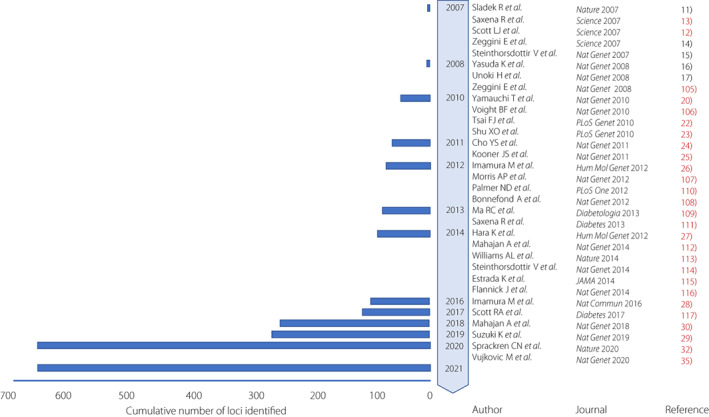
Timeline of key discoveries of type 2 diabetes genetics by genome‐wide association study (GWAS). GWAS identified genetic loci for type 2 diabetes at the genome‐wide significance level (P<
5.0
×
10−8). Cumulative count of discovered loci per year are presented.
KCNQ1 is a disease susceptibility locus with a relatively high odds ratio of 1.4 identified in the Japanese population in 2008, but it is highly reproducible, including in the European population. 10 , 11 KCNQ1 protein is one of the voltage‐dependent potassium channels and has been reported to cause long QT syndrome by altering repolarization in cardiomyocytes. 43 , 44 In 2010, a large‐scale GWAS was performed using 459,359 SNPs in 4,470 people with diabetes. The GWAS identified UBE2E2 and C2CD4A/B as disease susceptibility loci for type 2 diabetes. 13 A ubiquitin‐proteasome system has been reported to play a pivotal role in insulin secretion. 45 In addition, the genetic score using 13 disease susceptibility loci of type 2 diabetes was developed to explain the difference of the risk of diabetes. 13 .
A genome‐wide association study identified five novel loci in a Han Chinese population, 15 , 16 , 17 and seven novel loci in a South Asian population. 18 One locus was newly identified by imputation with the sequencing information of the 1,000 Genome Project and three new loci of disease susceptibility to type 2 diabetes were identified by large‐scale GWAS in a Japanese population. 19 , 25 A larger meta‐analysis identified seven new disease‐susceptible loci of type 2 diabetes, two of which were relatively characteristic of the East Asian population. 31 .
LARGE‐SCALE GWAS FOR TYPE 2 DIABETES IN JAPANESE POPULATION
To elucidate genetic and environmental factors of type 2 diabetes through the close collaboration of large cohorts of Japan (Biobank Japan, J‐MICC, JPHC‐NEXT, Tohoku Medical Megabank) and researchers in the medical and information sciences, it is important to propose a new standard for research on multifactorial diseases. In the Japanese population, a large GWAS meta‐analysis was performed in 36,614 case and 155,150 control participants. 36 GWAS was conducted on 12 million variants using a reference panel of 1,000 Genomes Project Phase 3 for imputation. Thus 88 regions and 115 independent signals associated with type 2 diabetes were identified. Only eight loci were common with the new loci reported by 900,000 participants in the European population, and the remaining 20 loci were specific to the Japanese population. 33 This shows that no matter how large the GWAS size of the European population becomes, it is not possible to identify the disease susceptibility loci specific to the Japanese population. Pathway analysis revealed that a pathway of maturity‐onset diabetes of the young is common to Japanese and European populations. The pathway of insulin secretion regulation was specific to the Japanese population.
In the GWAS in the Japanese population, including Biobank Japan, 15 new missense variants were identified. 36 It was newly reported that the variant of incretin‐related drug target gene GLP1R R131Q (rs3765467 GA/AA genotype) was associated with the risk of developing type 2 diabetes. 36 Healthy individuals with the GA/AA genotype had an increased incretin effect and the genotype is expected to be a drug reactivity marker. 46 The frequency of the GA/AA genotype of rs3765467 is 32% in the Japanese population, but it is extremely rare in the European population and difficult to identify even in GWAS with a 900,000 European population. 33
EAST ASIAN AND TRANS‐ETHNIC GWAS FOR TYPE 2 DIABETES
A meta‐analysis of a large‐scale GWAS of 400,000 people was conducted to identify common type 2 diabetes loci in the East Asian population. 35 The number of cases of type 2 diabetes surpassed that of the European GWAS at that time. The Japanese population made the largest contribution to the number of cases. In total, 183 risk loci were associated with type 2 diabetes and 61 loci were novel. In addition to loci that are significant in both European and East Asian populations, loci that are specific to the East Asian population were identified. For example, TCF7L2 had the same orientation and magnitude of effect in both populations. On the other hand, DGKD and ZNF257 had an effect only in the East Asian population. The effect sizes of the allele adjusted for BMI were highly correlated to those of the allele unadjusted for BMI in the East Asian population. It was speculated that changes in fat distribution may play a larger role in causing type 2 diabetes than BMI in the East Asian population. In addition, the FTO region, which is known to be most strongly related to BMI, was similarly attenuated by BMI correction in both East Asian and European populations.
Regarding the KCNQ1 region that showed the most significant correlation with type 2 diabetes, nine signals were identified in the KCNQ1 region. 35 In the previously reported ANK1/NKX6‐3 region, three independent signals were found this time. 35 Of these, signal 1 (rs33981001) was adjacent to a variant that regulates the gene expression of NKX6‐3 on the islet of Langerhans of the pancreas. On the other hand, signal 2 (rs62508166) was adjacent to a variant that regulates the gene expression of ANK1 in subcutaneous adipose tissue and skeletal muscle. It is speculated that two different signals in the same genetic region affect the risk of developing type 2 diabetes by modulating the expression of different genes in different tissues.
Among the loci identified in this study, the locus where the effect on the risk of developing type 2 diabetes differs between men and women was the aldehyde dehydrogenase 2 (ALDH2) locus (rs12231737). 35 The ALDH2 locus was strongly associated with the risk of developing type 2 diabetes in men, but not at all in women. It has been reported that the ALDH2 locus is related to adaptive evolution in the East Asian population. 47 , 48 In addition, ALDH2 protein is an enzyme that regulates alcohol metabolism. The allele was associated with tolerance to alcohol, a high risk of developing type 2 diabetes, obesity, blood pressure, and serum triglycerides.
Trans‐ethnic GWAS for type 2 diabetes and diabetic complications was conducted. Vujkovic et al. 34 investigated type 2 diabetes genetic susceptibility via multi‐ancestry meta‐analysis of 228,499 cases and 1,178,783 controls in the DIAMANTE and Million Veteran Program (MVP), including Biobank Japan and other studies. In total, 568 associations and 318 novel loci for type 2 diabetes were identified. Novel loci in the diabetic complications were reported. The polygenic risk score of diabetic retinopathy was shown to strongly associate with an increased risk of diabetic retinopathy. 34
GENOMIC ANALYSIS OF DIABETIC KIDNEY DISEASE
Diabetic kidney disease is a multifactorial disease that develops with the involvement of multiple genetic factors and environmental factors. 49 The elucidation of genetic factors has been advanced, narrowing several related genetic regions by analysis using family lineage. 50 As a candidate gene, the erythropoietin gene was associated with diabetic kidney disease. Disease susceptibility genetic loci have been identified in diabetic kidney disease by GWAS. The functions of the identified genes have been elucidated using human kidney, cultured cells, and model mice. 51
In 2009, a GWAS of diabetic nephropathy in type 1 diabetes in the European population reported four loci that did not reach genome‐wide levels. In the Japanese population with type 2 diabetes, GWAS identified the ACACB (acetyl coenzyme A carboxylase β) locus with a P‐value of over 5×
10−8.
52
In the Genetics of Nephropathy an International Effort (GENIE) in diabetic nephropathy of type 1 diabetes in European population, AFF3 (AF4/FMR2 member 3) and RGMA/MCTP2 (RGM domain family, member A/Multiple C2 domains, transmembrane 2) loci were significantly associated at the genome‐wide level
53
(Figure 2). AFF3 protein has been reported to be involved in TGF‐β signaling. The CDCA7/SP3 (cell division cycle associated 7/transcription factor Sp3) locus was significantly associated with end‐stage renal failure in women with type 1 diabetes.
54
The locus of GLRA3 (glycine receptor, alpha‐3 subunit) is identified to be associated with albuminuria in type 1 diabetes.
55
The protein encoded by GLRA3 is a ligand‐gated chloride channel responsible for mediating the inhibitory effects of glycine.
56
Glycine is the predominant amino acid stimulator of glucagon secretion in pancreatic α‐cells, acting through the glycine receptors. In the European population, intergenic locus of SCAF8/CNKSR3 (SR‐like carboxyl‐terminal domain associated factor 8 gene/connector enhancer of KSR family of scaffold proteins gene) was identified.
57
The protein encoded by CNKSR3 is related to aldosterone signals. The UMOD locus showed a genome‐wide significant association with eGFR (estimated glomerular filtration ratio) among those with diabetes.
58
Taira et al.
59
identified the FTO locus and found that it confers susceptibility to diabetic nephropathy. The FTO locus has been reportedly associated with obesity.
60
The FTO variants confer susceptibility to chronic kidney disease through the mechanisms mediated by obesity.
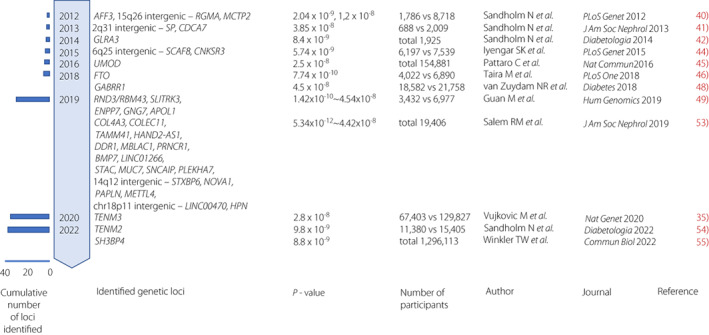
Timeline of key discoveries of genetics of diabetic kidney disease by genome‐wide association study (GWAS). GWAS identified genetic loci for type 2 diabetes at the genome‐wide significance level (P<
5.0
×
10−8). Cumulative count of discovered loci per year are presented.
The Surrogate markers for Micro‐ and Macrovascular hard endpoints for Innovative diabetes Tools (SUMMIT) consortium has conducted an analysis of 40,000 individuals with diabetic kidney disease and type 2 diabetes. Seven phenotypes of diabetic kidney disease were compared based on stage of the disease. In the comparison of individuals with microalbumin urine and those without microalbumin urine, the GABRR1 (Rho1 subunit of the GABA type A receptor) loci reached a genome‐wide significant level. 61 For the association with glomerular estimated filtration, five loci were significant at genome‐wide levels, including the APOL1 and ENPP7 locus. 62 APOL1 (apolipoprotein L1) G1 and G2 alleles gene contribute to 60% of non‐diabetic end‐stage kidney disease in African Americans. 63 The protein encoded by ENPP7 is an intestinal phosphodiesterase that converts sphingomyelin to ceramide and phosphocholine. ENPP7 protein is reported to affect cholesterol absorption. 64 Studies suggest that the high‐density lipoprotein cholesterol levels are risk factors for diabetic kidney disease. 65
A meta‐analysis of GWAS including 19,406 individuals with type 1 diabetes from the Diabetic Nephropathy Collaborative Research Initiative (DNCRI) identified 16 novel loci including COL4A3, COLEC11, DDR1, and BMP7. A common missense variant in the COL4A3 gene showed the strongest association. 66 Variants in the COL4A3 gene are responsible for Alport syndrome, a progressive inherited nephropathy. The COLEC11 gene encodes a collectin‐11 protein. DDR1 encodes the discoidin domain‐containing receptor 1. Collectin‐11 protein has a role in host defense against pathogens and DDR1 protein binds collagens. BMP7 (bone morphogenetic protein 7) is a member of TGF‐β superfamily and has a role in renal development and fibrosis. TENM2 and TENM3 loci are reported to associate with diabetic nephropathy. 67 , 68 TENM2 (teneurin transmembrane protein 2) is involved in cell–cell adhesion. TENM3 has been associated with kidney disorders in UK Biobank. 69
GENETICS OF DIABETIC RETINOPATHY
Diabetic retinopathy causes blindness among individuals with diabetes. 70 , 71 Beside candidate gene analysis and linkage analysis, GWAS were performed in various populations. It was reported that the locus of CAMK4 (calcium/calmodulin‐dependent protein kinase IV) was found to be associated with diabetic retinopathy in a GWAS performed in a Mexican population without reaching genome‐wide significance. 72 Huang et al. 73 conducted GWAS in a Taiwanese population and identified loci including ARHGAP22 reaching genome‐wide significance (Figure 3). ARHGAP22 (Rho GTPase‐activating protein 22) is implicated in endothelial cell angiogenesis and increased capillary permeability. Grassi et al. performed GWAS in individuals with severe diabetic retinopathy in two large cohorts of type 1 diabetes: Genetics of Kidney in Diabetes (GoKinD) and EDIC. The study included 973 cases and assessed them to identify an intergenic locus without reaching genome‐wide significance. 74 Burdon et al. 75 reported genetic variation near GRB2 (growth factor receptor bound‐2) locus associated with sight‐threatening diabetic retinopathy. GRB2 protein was reported to be expressed in all the retina layers, including blood vessels. GRB2 binds phosphorylated IRS‐1 (insulin receptor substrate 1) and activates the MAPK pathway by insulin stimulation. GRB2 is involved in VEGF signaling. GWAS of severe diabetic retinopathy suggested evidence for the involvement of the locus of NOX4 (NADPH oxidase 4) in the European population. 76 NOX4 protein acts as an oxygen sensor and catalyzes the reduction of molecular oxygen. In a GWAS in European and African cohorts, the locus in an intron of NVL (nuclear valosin‐containing protein‐like) was found to confer susceptibility to diabetic retinopathy in European population. NVL is expressed widely in the retina and encodes the AAA (ATPases associated with the cellular activities) superfamily. 77
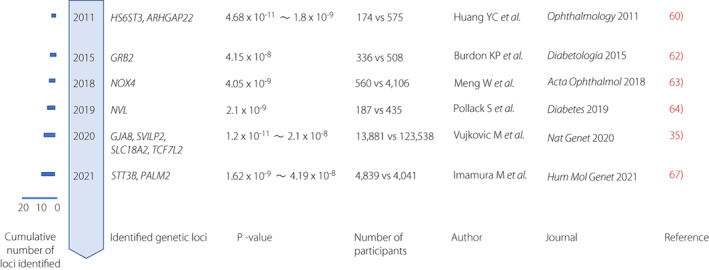
Timeline of key discoveries of genetics of diabetic retinopathy by genome‐wide association study (GWAS). GWAS identified genetic loci for type 2 diabetes at the genome‐wide significance level (P<
5.0
×
10−8). Cumulative count of discovered loci per year are presented.
Four loci were found to have effects on diabetes retinopathy in GWAS meta‐analysis. 34 The strongest signal for interaction to retinopathy was observed for GJA8. 34 Deletion of GJA8 gene leads to eye abnormalities and retinopathy in premature infants, inherited cataracts, and visual impairment. 78 , 79 TCF7L2 is found to be a locus associated with both type 2 diabetes and diabetic retinopathy. The product of SLC18A2 gene is involved in the transport of monoamines. The protein encoded by SVILP1 has been reported to be associated with thiamine (vitamin B1) metabolism. Imamura et al. 80 conducted a GWAS in the Japanese population and identified novel loci near STT3B (Oligosaccharyl transferase Complex Catalytic Subunit B) and PALM2 (paralemin‐2) to be associated with diabetic retinopathy. Deletion of STT3B mRNA causes a congenital neurological disease with a lack of visual tracking and the optic nerve hypoplasia.
GENETICS OF DIABETIC NEUROPATHY
Diabetic neuropathy is the most common complication and increases the risk of foot ulcer and lower‐extremity amputation. 81 Diabetic neuropathy is presented as peripheral and autonomous neuropathy. Several gene polymorphisms in candidate gene have been studied including ACE (angiotensin converting enzyme). 82
Meng et al.
83
conducted GWAS in 699 diabetic foot ulcers in the Genetics of Diabetes Audit and Research in Tayside Scotland (GoDARTs; Figure 4). The control was defined as an individual with diabetes who had never been recorded as having a foot ulcer but who had a positive monofilament test result recorded in their e‐health records. The MAPK14 locus reached genome‐wide significance with a P‐value of 2.45×
10−8. MAPK14 protein is one of the p38 MAPKs and plays a role in the cellular response to proinflammatory cytokines. Tang et al.
84
performed GWAS in the Action to Control Cardiovascular Risk in Diabetes (ACCORD) clinical trial including 4,384 individuals with diabetic peripheral neuropathy. The minor allele of the rs13417782 decreased the odds of diabetic peripheral neuropathy by 36%. This protective allele was associated with a higher expression of a gene SCN2A. The protein encoded by SCN2A is voltage‐gated sodium channel Nav1.2. The NRP2 locus was identified in GWAS meta‐analysis of 40,475 individuals with diabetic neuropathy.
46
NRP2 encodes neuropilin‐2 which is a receptor involved in angiogenesis and neural regeneration. Recently, metagenomic sampling of diabetic ulcers was performed and microbial dynamics were assessed to determine relationships to clinical events.
85
,
86
Larger scale GWAS and a metagenomic study is clearly needed to fully understand the mechanisms of the development of diabetic neuropathy.
POLYGENIC RISK SCORE FOR PREDICTION OF THE DEVELOPMENT OF DIABETES AND THE THERAPEUTIC EFFECTS OF DIABETIC COMPLICATIONS
The polygenic risk score (PRS) is calculated by the weighted sum of risk allele counts of the variants, using their effect sizes from GWASs as their weights. 87 The power of PRSs has increased due to larger GWAS sample sizes and advanced computational algorithms compared with classical genetic risk score. 88 The polygenic risk score can be constructed that identifies clinically meaningful genetic predisposition for diseases and the response to their treatments. Large‐scale GWAS and biobank information have been used to demonstrate the usefulness of PRS with the significant variants and non‐significant weakly influential variants. It was reported that approximately 20% of people with high PRS have a triplicate risk of cardiovascular disease, atrial fibrillation, type 2 diabetes, Crohn's disease, and breast cancer. 89 It is estimated that the risk in the high‐risk group is similar to the risks of developing monogenic diseases with positive genetic testing. The clinical application of PRS for the prediction of the development of diabetes and diabetic complications is expected (Figure 5).
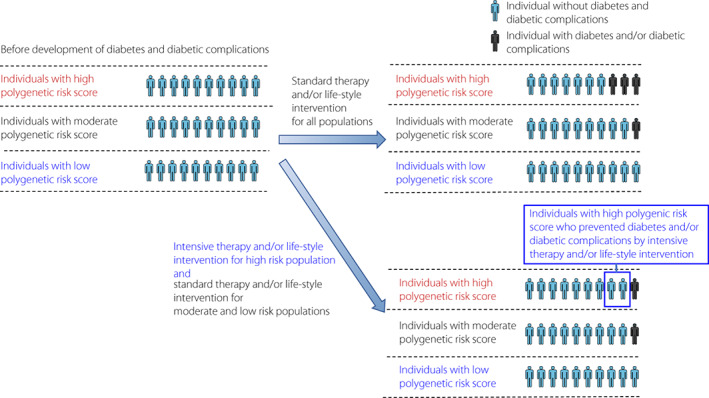
Predicting diabetes and diabetic complications using polygenic risk score. Intensive therapy and/or life‐style intervention for high‐risk group reduces the development of diabetes and diabetic complications compared with standard therapy and/or life‐style intervention for all groups.
Genome‐wide association studies have been mainly conducted in the European population, and about 80% of the participants are Europeans. 90 , 91 , 92 In type 2 diabetes, PRS created from data in a cohort of the European population have been reported to be less predictable when adapted to the Japanese population. 90 Since the frequency of variants involved in the onset and progression of the disease and the linkage around the causative variants differ among ethnic groups, it might not be possible to calculate the effect of the causative variant using GWAS data from different ethnic groups. For the clinical application of research results of genomic medicine and precision medicine, data obtained from large‐scale cohorts of each population are important.
In the ACCORD trial about diabetic complications, a subtype with an increased effect of intensive therapy was identified.
93
GWAS has been performed in the subtype classified by cluster HbA1c trajectory analysis. PRS using GWAS data were created to identify subtypes. In subtypes classified by PRS, it was reported that intensive therapy inhibited the development of coronary artery disease. In addition, in the ADVANCE (The Action in Diabetes and Vascular Disease: Preterax and Diamicron Modified Release Controlled Evaluation) trial, a multi‐PRS combining 10 PRS such as diabetes, obesity, and albuminuria with 598 single‐nucleotide polymorphisms was created. Multi‐PRS was useful for predicting the development of diabetic complications. In the high‐risk group, cardiovascular death was 4.4‐fold higher compared with the low‐risk group (HR 0.61 [95% CI 0.40, 0.93] for 4.5year follow‐up). In the high‐risk group, intensive therapy that strictly lowers plasma glucose levels and blood pressure was shown to have a larger effect of decreasing complications compared with the low‐risk group.
94
The number needed to treat (NNT) was 12 in the high‐risk group.
MULTI‐OMICS ANALYSIS FOR DIABETES AND DIABETIC COMPLICATIONS
We could now integrate information across multiple data types to gain a comprehensive molecular understanding to guide development of targeted therapy. 95 In relation to environmental factors, the role of genetic predisposition will be clarified by integrating information at each level of cells and tissues through multi‐omics analysis. Multi‐omics profiling is an important step toward precision medicine in metabolic diseases. 96 , 97 Multi‐omics techniques, in particular proteomics and metabolomics together with genomics, have the potential to enhance our understanding of the molecular process that develops type 2 diabetes and influences disease progression (Table 1). Ninty‐nine plasma proteins were associated with the incidence of developing type 2 diabetes in 2,940 participants. 98 Four plasma proteins were associated with pre‐diabetes in 439 participants. The biological process concerning these proteins were inflammatory signals, and lipoprotein metabolism. 99
Table 1
Findings by multi‐omics analysis, highlighting gaps in current knowledge about diabetes and diabetic complications
Disease | Omics analysis | Current research | Findings | Future research needs |
---|---|---|---|---|
Diabetes | Genomics | GWAS |
Insulin secretion Insulin resistance Polygenic risk score for type 2 diabetes |
Trans‐ethnic larger analysis Whole genome sequence Identification of rare variant |
Diabetic complications | Genomics | GWAS |
Increased inflammatory signals Increased oxidative stress Polygenic risk score for diabetic complications |
Replication in larger cohort Whole genome sequence Identification of rare variant |
Diabetes | Proteomics |
Targeted and non‐targeted approaches LC–MS, GC–MS Affinity‐based assay |
Increased inflammatory signals Altered lipoprotein metabolism Serum protein signatures for diabetes |
Replication in independent cohort Randomization study design Longitudinal time point sample collection |
Diabetic complications | Proteomics |
Targeted and non‐targeted approaches LC–MS, GC–MS Affinity‐based assay |
Increased inflammatory signals Serum and urine protein signatures for diabetic kidney disease |
Replication in independent cohort Incorporation of tissue‐based investigation Machine learning to select the target molecule |
Diabetes | Metabolomics |
Targeted and non‐targeted approaches NMR, LC–MS, GC–MS |
Increased BCAA synthesis Increased lipid synthesis |
Replication in independent cohort Randomization study design Longitudinal time point sample collection |
Diabetic complications | Metabolomics |
Targeted and non‐targeted approaches NMR, LC–MS, GC–MS |
Increased amino acid synthesis Altered HDL particles in diabetic nephropathy |
Replication in independent cohort Incorporation of tissue‐based investigation Machine learning to select the target molecule |
BCAA, branched‐chain amino acid; GC–MS, mass spectrometry with gas chromatographic separation; GWAS, genome‐wide association study; HDL, high density lipoprotein; LC–MS, mass spectrometry with liquid chromatographic separation; NMR, nuclear magnetic resonance.
The progression of diabetic kidney disease and diabetic retinopathy is variable, and little is known about the factors that protect against functional decline. Using targeted proteomics profiling, Niewczas et al. identified a kidney risk inflammatory signature consisting of 17 proteins enriched in tumor necrosis factor‐receptor superfamily members using plasma from cohorts with type 1 and type 2. The signature was associated with a 10‐year risk of end‐stage renal disease. 100 Using untargeted proteomic profiling, Kobayashi et al. measured 1,129 proteins by an affinity‐based method in the serum of individuals from two cohorts, the Joslin Kidney Study and the Pima Indian Kidney Study. Eleven candidate proteins were identified. The biological process concerning these proteins were a neuron development‐related process, organic acid binding, and cell–cell junction assembly. 101 Md Dom and colleagues identified plasma proteins associated with protection against diabetic kidney disease progression in individuals with type 1 and type 2 diabetes. 102 It is reported that three plasma proteins, fibroblast growth factor 20, angiopoietin‐1, and tumor necrosis factor ligand super family member 12, were elevated. 102 In 1,775 participants with type 2 diabetes, the combined score from the data of 273 urinary proteins measured by mass spectrometry has a high accuracy in predicting the new onset of albuminuria, after adjustments for HbA1c, blood pressure, baseline albuminuria, and eGFR. 103 Wu et al. 104 constructed machine learning‐assisted models to identify a biomarker signature that predicts high risk participants with diabetes developing diabetic kidney disease based on clinical information, metabolomics, lipidomics, and single nucleotide polymorphism datasets. The biomarker signature of 10 features was useful when identifying subjects at high risk of renal impairment in type 2 diabetes (AUC (area under the curve) 0.76). 104
Concerning metabolomics analysis, many studies have been conducted and identified the associations between type 2 diabetes and branched‐chain amino acid (BCAA), and aromatic amino acid. 105 BCAA was found to be associated with an increased risk of type 2 diabetes during a 12‐year follow‐up in the Malmo diet and Cancer study. 106 Blood metabolites related to amino acid metabolism and fatty acid metabolism have been reported to be associated with diabetic kidney disease. 105 High‐density lipoprotein (HDL) particles and their composition have been associated with higher eGFR in type 2 diabetes. 107 , 108 Yoshioka et al. 109 performed an untargeted metabolomic analysis of patients with stage G3 diabetic kidney disease and identified increased urinary lysophosphatidylcholine in fast functional decline. It has been reported that blood metabolites related to amino acid metabolism, and fatty acid metabolism were associated with diabetic retinopathy. 110 , 111 These metabolites could serve as biomarkers to stratify individuals according to their risk of disease progression. However, the number of participants was small in these studies, which resulted in less consistent findings.
PROSPECTS FOR THE REALIZATION OF PRECISION MEDICINE
Genome‐wide association studies have identified genetic predisposition in each stage of kidney damage with indicators of kidney function. Comprehensive analysis of genetic predisposition is shifting from GWAS centered on polymorphism analysis to whole‐genome sequencing using next‐generation sequencers (Table 1). 112 , 113 In diabetes and diabetic complications, more variants specific to race are thought to be found in variants with an allele frequency of <5% and a low frequency of 1% or more. Because rare variants have a strong effect on the onset of disease, they are less frequent due to negative selection pressures, some of which are difficult to identify, and a larger number of cases is considered necessary.
Proteomics and metabolomics studies have been conducted and they have identified the association between type 2 diabetes and inflammatory signals and amino acid synthesis. For a comprehensive understanding of type 2 diabetes, replication in an independent cohort, a randomization study design, and longitudinal sample collection are needed. In addition, proteomics and metabolomics studies showed that inflammatory signals and amino acid synthesis are increased in diabetic complications. To elucidate the genetic predisposition of diabetic kidney disease, it is important to increase the number of cases to be analyzed and to obtain information on phenotypes with high accuracy. Wu et al. 104 developed machine learning‐assisted models to identify a biomarker signature which was useful when identifying subjects at high risk of renal impairment in type 2 diabetes. Replication in independent cohort and the incorporation of tissue‐based investigation, and utilization of machine learning to select the target molecule will elucidate the mechanistic implications of biomarkers. It is expected that polygenic risk scores and biomarkers developed from multi‐omics analysis will improve the predictability of the disease onset, and that the disease can be overcome by the development of new therapeutic drugs utilizing these data. 114 , 115
An atlas of disease risk genes has been created in 180,000 people from Biobank Japan, 360,000 from the UK Biobank, and 140,000 from FinnGen. 116 The utilization of a gene atlas will enable the precise classification of diseases. In addition to the optimization and repositioning of existing treatments, new drug discovery such as Adiporon, an agonist of the adiponectin receptor that is greatly involved in the pathophysiology of type 2 diabetes and diabetic complications, is important for the realization of genomic medicine suitable for the lifestyle of individuals. 117
CONCLUSION
Many genetic loci related to diabetes and diabetic complications have been discovered, and it is expected that the mechanism of disease onset will be elucidated and its implementation in medical care will progress by utilizing the polygenic risk score and multi‐omics analysis. For this challenge to succeed and sustain, it is important to communicate with society. 118 Ethical considerations should be addressed so that participants’ right to know and not know are adequately protected, and genomic research is truly beneficial.
AUTHOR CONTRIBUTIONS
N.S., and T.Y., wrote the manuscript. All authors contributed to the article and approved the submitted version.
DISCLOSURE
The authors declare no conflict of interest.
Approval of the research protocol: N/A.
Informed consent: N/A.
Registry and the registration no. of the study/trial: N/A.
Animal studies: N/A.
ACKNOWLEDGMENTS
Type 2 diabetes GWAS in Japanese population is the result of the kindness and efforts of many collaborators. Dr Ken Suzuki (Manchester University, The University of Tokyo, Osaka University), Prof. Masato Akiyama (Kyushu University), Prof. Yoichiro Kamatani (The University of Tokyo), Dr Momoko Horikoshi (RIKEN), Prof. Yukinori Okada (Osaka University, The University of Tokyo), Dr Takashi Kadowaki (Toranomon Hospital, The University of Tokyo) gave us tremendous guidance, and we were able to obtain genome data from Biobank Japan, Tohoku Medical Megabank Organization, Iwate Tohoku Medical Megabank Organization, and Japan multi‐purpose research cohort. In type 2 diabetes GWAS in East Asian population, Dr Momoko Horikoshi became the head of the Japan research group and made great efforts. We would like to take this opportunity to express our sincere gratitude to all our collaborators. This work was supported by grants from the Japan Agency for Medical Research and Development (JP21tm0424218).
REFERENCES
Articles from Journal of Diabetes Investigation are provided here courtesy of Wiley
Full text links
Read article at publisher's site: https://doi.org/10.1111/jdi.13970
Read article for free, from open access legal sources, via Unpaywall:
https://onlinelibrary.wiley.com/doi/pdfdirect/10.1111/jdi.13970
Citations & impact
Impact metrics
Citations of article over time
Alternative metrics

Discover the attention surrounding your research
https://www.altmetric.com/details/141287914
Smart citations by scite.ai
Explore citation contexts and check if this article has been
supported or disputed.
https://scite.ai/reports/10.1111/jdi.13970
Article citations
Multi-Omics Analysis Revealed the rSNPs Potentially Involved in T2DM Pathogenic Mechanism and Metformin Response.
Int J Mol Sci, 25(17):9297, 27 Aug 2024
Cited by: 0 articles | PMID: 39273245 | PMCID: PMC11394919
Polyunsaturated fatty acids and diabetic microvascular complications: a Mendelian randomization study.
Front Endocrinol (Lausanne), 15:1406382, 07 Aug 2024
Cited by: 0 articles | PMID: 39170741 | PMCID: PMC11335686
Genome-Wide Polygenic Risk Score Predicts Incident Type 2 Diabetes in Women With History of Gestational Diabetes.
Diabetes Care, 47(9):1622-1629, 01 Sep 2024
Cited by: 0 articles | PMID: 38940851
AI-enhanced integration of genetic and medical imaging data for risk assessment of Type 2 diabetes.
Nat Commun, 15(1):4230, 18 May 2024
Cited by: 0 articles | PMID: 38762475 | PMCID: PMC11102564
Differences in gut microbiota between Dutch and South-Asian Surinamese: potential implications for type 2 diabetes mellitus.
Sci Rep, 14(1):4585, 26 Feb 2024
Cited by: 1 article | PMID: 38403716 | PMCID: PMC10894869
Go to all (10) article citations
Data
Data behind the article
This data has been text mined from the article, or deposited into data resources.
BioStudies: supplemental material and supporting data
SNPs (3)
- (2 citations) dbSNP - rs3765467
- (1 citation) dbSNP - rs12231737
- (1 citation) dbSNP - rs13417782
Similar Articles
To arrive at the top five similar articles we use a word-weighted algorithm to compare words from the Title and Abstract of each citation.
Perspectives on genetic studies of type 2 diabetes from the genome-wide association studies era to precision medicine.
J Diabetes Investig, 15(4):410-422, 23 Jan 2024
Cited by: 2 articles | PMID: 38259175 | PMCID: PMC10981147
Review Free full text in Europe PMC
Genome-wide association studies for diabetic macular edema and proliferative diabetic retinopathy.
BMC Med Genet, 19(1):71, 08 May 2018
Cited by: 29 articles | PMID: 29739359 | PMCID: PMC5941644
Novel susceptibility loci identified in a genome-wide association study of type 2 diabetes complications in population of Latvia.
BMC Med Genomics, 14(1):18, 11 Jan 2021
Cited by: 10 articles | PMID: 33430853 | PMCID: PMC7802349
Evaluation of type 2 diabetes genetic risk variants in Chinese adults: findings from 93,000 individuals from the China Kadoorie Biobank.
Diabetologia, 59(7):1446-1457, 06 Apr 2016
Cited by: 29 articles | PMID: 27053236 | PMCID: PMC4901105
Funding
Funders who supported this work.
Japan Agency for Medical Research and Development (1)
Grant ID: JP21tm0424218