Abstract
Free full text

Route Determination of Sulfur Mustard Using Nontargeted Chemical Attribution Signature Screening
Associated Data
Abstract
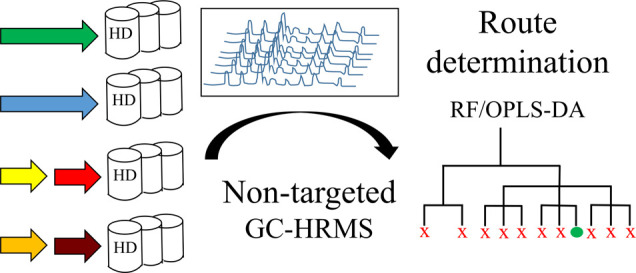
Route determination of sulfur mustard was accomplished through comprehensive nontargeted screening of chemical attribution signatures. Sulfur mustard samples prepared via 11 different synthetic routes were analyzed using gas chromatography/high-resolution mass spectrometry. A large number of compounds were detected, and multivariate data analysis of the mass spectrometric results enabled the discovery of route-specific signature profiles. The performance of two supervised machine learning algorithms for retrospective synthetic route attribution, orthogonal partial least squares discriminant analysis (OPLS-DA) and random forest (RF), were compared using external test sets. Complete classification accuracy was achieved for test set samples (2/2 and 9/9) by using classification models to resolve the one-step routes starting from ethylene and the thiodiglycol chlorination methods used in the two-step routes. Retrospective determination of initial thiodiglycol synthesis methods in sulfur mustard samples, following chlorination, was more difficult. Nevertheless, the large number of markers detected using the nontargeted methodology enabled correct assignment of 5/9 test set samples using OPLS-DA and 8/9 using RF. RF was also used to construct an 11-class model with a total classification accuracy of 10/11. The developed methods were further evaluated by classifying sulfur mustard spiked into soil and textile matrix samples. Due to matrix effects and the low spiking level (0.05% w/w), route determination was more challenging in these cases. Nevertheless, acceptable classification performance was achieved during external test set validation: chlorination methods were correctly classified for 12/18 and 11/15 in spiked soil and textile samples, respectively.
Chemical forensics is the science of attributing chemical samples to sources by analyzing their content of specific compounds or establishing links between samples based on similarities in their chemical profiles.1 It has become important as a tool for the attribution of alleged use of chemical warfare agents (CWA).2−9 The Organization for the Prohibition of Chemical Weapons (OPCW) was recently mandated to attribute the parties responsible for the use of chemical weapons in a recent armed conflict.10 In such investigations, chemical forensics tools can provide important data needed to link separate chemical attacks or determine how specific CWA samples were produced.11 Chemical forensics is also used in police investigations, for example, to determine the origin of seized drugs,12,13 and for fire debris investigations.14 Sample matching based on chemical profiling can establish a common origin of seized materials or even show that they originate from the same production batch or the same synthetic route.3,6,15,16 Chemical attribution signatures (CAS) may include extrinsic markers such as by-products from synthesis and chemical impurities in the starting material. An alternative approach is to examine intrinsic properties of the CWAs, such as elemental isotope ratios in the compound (s) under investigation.17,18
Sulfur mustard (HD) has historically been the most widely used CWA in armed conflicts,19 and its recent use in the Arabic Republic of Syria has been reported.20 HD can be synthesized via several different routes, either directly from ethylene or in a two-step process via the intermediate thiodiglycol (TDG). We have previously used a targeted method based on GC–MS analysis to identify CAS for some of these routes, enabling partial resolution of 11 production routes.21 By this method, all detected chemicals, not present in the blank samples, were manually included in a target library based on their mass spectra and retention indices. Both compounds identified by spectra library search and unidentified compounds were included. This method can source unknown samples correctly to single-step synthesis routes and identify the chlorination method used in two-step routes involving TDG. Unfortunately, GC–MS analyses of HD samples did not provide sufficiently detailed CAS profiles to enable discrimination between the three synthetic routes to TDG included in the study. This indicates that an analytical tool with higher sensitivity and resolution is needed to detect markers specific to particular routes of TDG synthesis. The extraction of significant CAS from large high-resolution datasets also requires an alternative strategy, possibly based on nontargeted screening.
The aim of nontargeted screening is to include as many compounds as possible by combining a broad sample extraction method with a general analytical method that can detect chemicals with diverse chemical properties.22 The analytical data is processed without prior assumptions about possible target compounds. Nontargeted screening has successfully been used in environmental chemistry to discover potentially toxic chemicals and investigate changes in levels of pollutants over time. For example, it was used to screen groundwater sites23 and sewage sludge22 for pollutants or to study environmental contaminants.24 Identification of analytes is often preferred in nontargeted screening; the level of identification can range from simply determining an exact mass to structural confirmation using reference standards.25
Efforts to verify alleged uses of chemical weapons can be expected to require chemical analysis of CWA in environmental samples.26 CWA and/or their degradation products can be extracted from contaminated samples of water, soil, or solid materials from the site of a chemical attack.27 There have been a few prior studies on route attribution of CWAs in relevant matrices, focusing on compounds related to production methods for Russian VX in food samples,28,29 acephate and CWA-related compounds extracted from dust,30 nerve agents extracted from indoor furnitures,31 and the sarin surrogate dimethyl methylphosphonate extracted from painted wallboards32 and office media.5
Chemometric data analysis and machine learning algorithms are powerful tools for the extraction of information from the high-dimensional and complex data normally generated by chemical profiling methods. Based on learning datasets, statistical models can be built in order to classify unknown samples. To be useful in court, the statistical models have to be validated and the classification results translated into a quantitative measure of evidence for or against a proposition. The use of likelihood ratio methods for evidence evaluation is established in forensic statistics,33 and it has also been suggested as a tool for assessment of propositions using multivariate chem-bio forensic data.34
The aim of the study presented here was to develop a sensitive analytical methodology based on high-resolution mass spectrometry to allow extraction of CAS suitable for discriminating between HD synthesis routes. A general nontargeted data processing method was used to enable efficient processing of large data matrices and thereby improve CAS detection.
Experimental Procedures
Chemicals
Ethyl acetate (99.8% purity), dichloromethane (99.8% purity), and dibenzothiophene (98% purity) were purchased from Merck, Darmstadt, Germany. Soil (Clean Sandy Loam certified reference material) from Sigma–Aldrich, MO, U.S., and a textile (unbleached cotton, 200 g/m2) were also used in the experiments.
Synthesis of HD
Crude samples of HD representing 11 production methods (Figure Figure11) were prepared in house. HD was prepared via the intermediate thiodiglycol (TDG) in routes 1 to 9 (R1–R9). The TDG was produced by three methods and was subsequently transformed into HD using three chlorination protocols, resulting in nine two-step HD production methods that are collectively referred to as TDG routes. Two additional routes (R10 and R11) were included in which gaseous ethylene is directly transformed into HD. Four replicate batches of HD were synthesized by each route and were used to construct attribution models. Crude HD for a test set (one replicate of each of the TDG routes, R1–R9) was synthesized independently, approximately two years after the crude HD training set. However, test set samples for the ethylene routes were obtained from pooled training set samples representing R10 and R11. All synthesis HD batches were stored at room temperature for 1 week and then diluted to 1 mg/mL in dichloromethane, followed by storage at −20 °C.
Sample Preparation
An overview of the samples included in this study is presented in Table 1, and an overview of the study’s workflow is shown in Figure Figure33. Crude HD samples, used for training and test sets, were diluted in dichloromethane to a concentration of 500 ng/μL. An internal standard, dibenzothiophene (1 ng/μL), was added to all samples and used to evaluate performance in terms of peak integration parameters and isotope ratio filters, and to enable semiquantification of CAS by TIC area comparison.
Table 1
crude
HD samples | spiked matrix samplesa | |||
---|---|---|---|---|
synthesis routes | training set | test set | training setb | test set |
R1 | 4 | 1 | 6 + 6 | 2 + 2 |
R2 | 4 | 1 | 6 + 6 | 2 + 2 |
R3 | 4 | 1 | 6 + 6 | 2 + 2 |
R4 | 4 | 1 | 6 + 6 | 2 + 2 |
R5 | 4 | 1 | 6 + 6 | 2 + 2 |
R6 | 4 | 1 | 6 + 6 | 2 + 2 |
R7 | 4 | 1 | 6 + 6 | 2 + 2 |
R8 | 4 | 1 | 6 + 6 | 2 + 2 |
R9 | 4 | 1 | 6 + 6 | 2 + 2 |
R10 | 4 | 1c | 6 + 6 | 2 + 2 |
R11 | 4 | 1c | 6 + 6 | 2 + 2 |
All matrix training samples were spiked with pooled crude HD samples. Pooled crude HD samples representing each route (R1–R11) were prepared by mixing 250 μL of each replicate sample for the route in question. The use of pooled crude HD samples made it possible to add exactly the same solution to all six soil and textile matrix samples. Spiked matrix samples were prepared by adding 50 μL of the appropriate pooled crude HD sample (1 mg/mL) to 0.1 ± 0.01 g of soil or textile, theoretically resulting in the addition of HD to a level of 0.05% w/w. Spiked matrix training samples were prepared in triplicate on two occasions, giving six replicates for each material. The matrix test set was prepared by adding 50 μL of the crude HD test set batches (R1–R9, 1 mg/mL) to 0.1 g ± 0.01 g of soil and textile samples in duplicates. All spiked matrix samples were stored in closed glass vials at room temperature overnight. Spiked soil samples were extracted by adding 1 mL of ethyl acetate and shaking (VWR Mini Shaker, PA, U.S.) at 200 rpm for 30 min, followed by centrifugation (Allegra 25 F Beckman Coulter, Bromma, Sweden) at 2500 rpm for 3 min. Ethyl acetate was then decanted, and the extraction process was repeated once, after which the two ethyl acetate extracts were combined. Spiked textile samples were extracted by ultrasonication (Sonorex Digitec Bandlin, Berlin, Germany) in 3 mL of ethyl acetate for 10 min. The ethyl acetate phase was then removed, and the material was extracted once more, after which the two extracts were combined. The combined extracts were filtered (Chromacol PTFE 1 μm, Sigma–Aldrich, MO, U.S.) and concentrated to a volume of 50 μL under a stream of nitrogen at 40 °C. At least one blank sample of each matrix was prepared on each sample preparation occasion. The blank matrix samples were spiked with 50 μL DCM and thereafter treated identically to the spiked matrix samples.
Chemical Analysis
Samples were analyzed with a Trace 1310 gas chromatograph coupled to an Exactive GC Orbitrap mass spectrometer (Thermo Scientific, MA, U.S.). A DB-5MS column (25 m, ID 0.25, 0.25, Agilent, CA, U.S.) was used to separate the compounds. The sample (1 μL) was injected splitless at 200 °C with helium as the carrier gas at a constant flow of 1.2 mL/min. The GC program started at 40 °C for 1 min, followed by a 10 °C/min increase to 300 °C and a hold at 300 °C for 5 min, giving a runtime of 32 min. Mass spectrometric scans were performed in the range of 30–750 m/z with a resolution of 30,000. The temperatures of the transfer line and ion source were set at 250 and 230 °C, respectively. Daily tuning and calibration ensured the quality of the mass spectrometry, and the instrument’s performance was monitored by daily analyses of QC samples.26 Crude HD and spiked matrix samples were analyzed in random order, and every sixth analysis run was done using a solvent blank sample. When analyzing spiked matrix samples, a solvent blank sample and a sample preparation blank were analyzed after every sixth nonblank sample.
Nontargeted Data Processing
The chromatographic data was processed by peak detection, retention time alignment, and peak integration followed by isotope ratio filtering. This resulted in a processed dataset with peak areas from extracted ions at specific retention times corresponding to different compounds. Data processing was done in Tracefinder (version 4.1, Thermo Scientific, MA, U.S.) using the analysis mode for unknown screening, which enables nontargeted screening of data. Peak picking was done with the deconvolution plugin (version 1.3, Thermo Scientific, MA, U.S.). The retention time alignment window was set to 10 s, the accurate mass tolerance to 10 ppm, the signal-to-noise (s/n) threshold to 5, the total ion-chromatogram intensity threshold to 500,000, the ion overlap window to 90–99%, and the response threshold to 10,000.
The extracted peaks were automatically time-aligned and integrated in the unknown screening view using the Avalon detection algorithm and the nearest RT detection method with seven smoothing points. Data representing compounds present in blank samples were manually removed from each crude HD dataset. The datasets for spiked soil and textile samples were processed and manually merged after removing peaks found in blank samples. The variation in the spiked matrix data was higher than in the crude HD data, so the m/z deviation threshold was increased to 0.01 to permit merging.
Isotope ratio filters
Data analysis was done both with and without isotope ratio filtration using sulfur and/or chlorine isotope filters, 1.9958 ± 10 ppm and 1.99705 ± 10 ppm, respectively. The internal standard was used as a control compound to ensure that peak detection was performed correctly and the sulfur isotope ratio filter settings were appropriate. Filtration was done to extract all peaks corresponding to analytes containing chlorine, sulfur, or both. It thus removed all other peaks, including matrix-associated peaks irrelevant to HD route classification. Mass defect filters have previously been used to select for metabolites based on their specific isotope ratios.35
Machine Learning
Data from the crude HD samples were initially analyzed by principal component analysis (PCA) to get an overview of the variation in the data and detect potential outliers. Subsequent analyses were performed in parallel using two machine learning algorithms: orthogonal partial least squares discriminant analysis (OPLS-DA)36 and random forest (RF),37 which were performed in Simca (version 15, Sartorius Stedim Biotech, Germany) and the R software (R38 version 4.0.3 with a random forest 4.6–1439 package), respectively.
OPLS-DA
Prior to analysis, the data was normalized by total area normalization, log-transformed, and scaled to unit variance. The data was too complex to allow classification of 11 routes with a single OPLS-DA model; full classification required a hierarchic decision tree guided by multiple OPLS-DA models. A similar approach was previously used to classify production routes of the nerve agent Russian VX, based on data for spiked food samples.28 The hierarchic decision tree method (Figure Figure22) first uses a classification model, M1, which distinguishes between the TDG routes (R1–R9) and the ethylene routes (R10 and R11). A second classification model, M2, was constructed to classify HD samples attributed to the TDG two-step routes (by M1) to the method of chlorination used in their synthesis, i.e., to attribute samples to one of the groups R(1, 4, 7), R(2, 5, 8), or R(3, 6, 9). Finally, data from HD samples sharing the same chlorination method was modeled to classify them based on the method used to synthesize TDG (M3a–c). Five models were constructed for the crude HD samples and another five for the spiked matrix samples. The OPLS-DA models were evaluated by cross-validation with all sample replicates included in the same cross-validation group to avoid overfitting. The classification models were also assessed in terms of their numbers of latent variables (OPLS components) and using three measures of performance: the variation in the data matrix explained by the model (R2X), the variation in the response matrix explained by the model (R2Y), and the variation in the response matrix predicted by the model (Q2). CAS important for class separation were identified by their predictive variable importance in projection (VIPpredictive) values.40
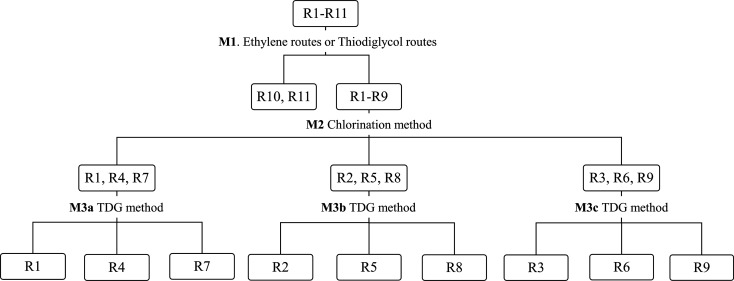
Hierarchical decision tree where the first model (M1) differentiates between ethylene route and TDG route samples. The second model (M2) differentiates between the three chlorination methods, while M3a–M3c differentiate between methods of TDG synthesis. This model tree was applied to the datasets for the crude HD samples and the spiked matrix samples
RF
The data was normalized by total area normalization. RF can handle complex datasets, so a classification model with 11 classes was constructed. The number of trees was set to 10,000 in all RF models, and the number of randomly sampled variables was optimized by comparing the out of bag (OOB)-estimated error rates for preliminary RF models with varying numbers of randomly sampled variables. The OOB-estimated error rate is the mean of the errors for each training set sample, calculated from decision trees generated while excluding the sample in question from the bootstrap sample. The number of randomly sampled variables in the final classification models is ranged from 3 to 75. To allow direct performance comparison with OPLS-DA, RF models were also used together with the hierarchical decision tree. In RF, CAS important for class separation were identified by considering their Gini impurity.37,41 The Gini impurity metric measures the purity of the classification tree nodes; variables with lower Gini values are more important in RF models.
Prediction performance
All RF and OPLS-DA models were validated using corresponding HD test sets.
Results and Discussion
Attribution of chemical samples to the production method is based on CAS profiling, i.e., identification and analysis of trace components that are diagnostic for specific production conditions or starting materials used. We evaluated a nontargeted GC–HRMS method for the analysis of crude HD samples and the scope for using the data generated to build classification models (Figure Figure33, top left).
The models’ performance was first tested by classifying test set samples originating from different synthetic batches of crude HD (Figure Figure33, bottom left), This procedure was repeated on soil and textile matrix samples, spiked with crude HD (Figure Figure33, right). The large number of compounds detected in this study illustrated the advantages of GC–HRMS and facilitated the detection of CAS related to the 11 HD routes. A total of 2713 compounds were detected in the crude HD samples, as compared to 103 compounds using targeted GC–MS.21 In addition, the s/n of the 103 previously detected compounds was significantly improved by using HRMS. Another advantage of HRMS is the ability to apply isotope ratio filtration. This facilitated the extraction of relevant CAS; we expected that the most useful CAS in this context would be chlorinated or sulfur-containing compounds. Isotope filtration reduced the number of compounds to consider and improved the quality of the OPLS-DA classification models (data not shown) and was thus applied throughout the study.
Crude HD Samples: Route Attribution through Nontargeted HRMS
The isotope ratio filtered data contained 714 potential CAS whose normalized peak areas were modeled in parallel using two machine learning algorithms, OPLS-DA and RF. The nontargeted method gave classification models of high quality (M1crude and M2crude, Table 2). Both OPLS-DA and RF successfully distinguished ethylene routes (R10 and R11) from TDG routes (R1–R9) and predicted the chlorination methods of TDG samples with 100% classification accuracy (Table 2). These results are consistent with previous findings.21 A significant advantage of nontargeted data processing in this context is that there is no need to create a target library of the detected compounds for each route.
Table 2
OPLS-DA | RF | ||||||||
---|---|---|---|---|---|---|---|---|---|
classification model | attribution capacity | class | comp.a | R2X (cum) | R2Y (cum) | Q2(cum) | prediction | OOB error (%) | prediction |
M1crude | ethylene or TDG routes | R(1–9) | 1 + 2 + 0 | 0.72 | 0.99 | 0.95 | 9/9 | 2.3 | 9/9 |
R(10, 11) | 2/2 | 2/2 | |||||||
M2crude | chlorination methods | R(1, 4, 7) | 2 + 2 + 0 | 0.61 | 0.98 | 0.95 | 3/3 | 3/3 | |
R(2, 5, 8) | 3/3 | 0.0 | 3/3 | ||||||
R(3, 6, 9) | 3/3 | 3/3 | |||||||
M3a crude | TDG synthesis methods of R(1, 4, 7) samples | R1 | 2 + 2 + 0 | 0.70 | 0.98 | 0.90 | 1/1 | 1/1 | |
R4 | 0/1 | 33.3 | 1/1 | ||||||
R7 | 0/1 | 1/1 | |||||||
M3bcrude | TDG synthesis methods of R(2, 5, 8) samples | R2 | 2 + 1 + 0 | 0.63 | 0.98 | 0.88 | 1/1 | 1/1 | |
R5 | 1/1 | 16.7 | 1/1 | ||||||
R8 | 0/1 | 1/1 | |||||||
M3ccrude | TDG synthesis methods of R(3, 6, 9) samples | R3 | 2 + 1 + 0 | 0.55 | 0.97 | 0.91 | 1/1 | 1/1 | |
R6 | 0/1 | 8.3 | 0/1 | ||||||
R9 | 1/1 | 1/1 |
Crude HD Samples: TDG Attribution through Nontargeted HRMS
Synthesis routes R1 to R9 involve the intermediate TDG, which can be produced by three different methods (Figure Figure11). It is challenging to classify the method of TDG synthesis in crude HD samples because the TDG-related CAS are present at levels of <100 pg./μL, approximately 10–100 times lower than those indicative of the chlorination method. This is probably due to the high overall purity of the TDG produced (89–99.5%), and the majority of the CAS have been transformed during the chlorination step. The improved detection of CAS by nontargeted HRMS significantly increased the scope for TDG attribution. Both OPLS-DA and RF models could differentiate the TDG synthesis methods based on 714 potential CAS. The external validation results showed that classification models M3a–ccrude successfully classified TDG synthesis methods in HD samples (OPLS-DA, 56%; RF; 89%, Table 2), showing that the nontargeted HRMS method detected sufficient TDG-related CAS to enable comprehensive route resolution.
CAS Determination and Compound Identity
Chemical identification of CAS is important for understanding their formation during HD production and for verifying that relevant CAS were extracted during the analysis. CAS can be identified in several ways, for example, by searching for specific compounds expected to be present based on prior knowledge, by visual comparison of detected compounds in samples, or by looking at variables found to be important in the classification models. The first two targeted approaches have limitations that restrict the amount of CAS information that can be extracted from the sample data. In this nontargeted work, we identified key CAS that were important variables in the route classification models. While some CAS were clearly associated with the chemistry of HD production, others had no obvious origin. However, evaluations of the effects of different variables on classification model performance clearly showed that successful attribution depended on complete CAS profiles rather than individual compounds (Figure S1, Table S1, S2 and S3).
The CAS found to be related to the chlorination method in two-step routes were largely identical to those previously found by GC–MS.21 PCl3 is used for chlorination in R2, R5, and R8, and gave rise to phosphorous-containing cyclic adducts, notably those with ID numbers M2crude_11 and M2crude_14 (Table S2). Chlorination with SOCl2 (R3, 6, and 9) produced HD containing chlorinated derivatives of amylene, a stabilizer in the dichloromethane used as the reaction solvent. Chlorination with HCl (R1, 4, and 7) yielded few CAS of low intensity. CAS associated with routes 10 and 11 included polysulfides and vinyl chlorides. Dithiane (ID number M3crude_6, Table S3) is a CAS related to TDG synthesis. Most of the compounds relevant to TDG synthesis method attribution using models M3acrude, M3bcrude, and M3ccrude could not be identified due to their low abundance (Table S3).
Spiked Matrix Samples: HD Route Attribution through Nontargeted HRMS
Because chemical forensic investigations of incidents involving chemical warfare agents may require analysis of samples taken from the environment, we also investigated the extraction of HD-associated CAS from spiked matrix samples. Route determination based on analysis of CWAs in environmental matrix samples may be complicated by interactions with the sample matrix and/or degradation of the chemicals that constitute the CAS. A low spiking level was chosen to further challenge the analytical method and classification models. Thus, 0.05% w/w of the HD produced by the various routes was spiked to the matrix samples, resulting in CAS concentrations in the range of 0.05–50 ppm. As shown in Figure S2, the most abundant peaks in the chromatograms, aside from the HD peak, originated from the matrix. The use of isotope ratio filters was essential when processing data for these samples because it extracted information on compounds containing chlorine and/or sulfur from the complex HRMS data. Despite the low spiking level, CAS profiles related to specific routes were detected in spiked matrix samples. However, there were large differences between the CAS profiles of crude HD and spiked matrix samples (Figure Figure44,) and the crude classifications models could not correctly classify spiked matrix samples. HD and many of the synthesis by-products are clearly highly reactive towards several matrix components, which dramatically altered the CAS attribution profiles. Only 48 of the 714 potential CAS in crude HD were chemically stable in both spiked matrices, and new potential CAS were formed in spiked soil and textile, respectively (Figure Figure44). The 48 CAS were not equally distributed between routes and most of them were not important for route separation. Although the CAS profiles of spiked soil and textile samples differed, sufficient common CAS were found to enable the construction of matrix models using the combined soil and textile data. Highly significant classification models could be created for the separation of ethylene routes and TDG routes, and for discriminating between chlorination methods, using either RF or OPLS-DA (Table 3). The quality (R2X, R2Y, Q2, and OOB) of spiked matrix models 1 and 2 (M1matrix and M2matrix) was comparable to the corresponding crude HD models (M1crude and M2crude, Tables 2 and 3). The chosen analytical technique and peak picking method thus made it possible to extract CAS from spiked matrix data despite the low spiking level. The most important variables in the RF and OPLS-DA M2matrix models were related to PCl3 chlorination (R2, 5, and 8), with a few relating to SOCl2 chlorination (R3, 6, and 9), as shown in Table S4. Of the 12 most important variables, only one was related to HCl chlorination. The chlorination method was correctly predicted in 70% of the spiked matrix test set samples independent of classification algorithm (Table 3). Adequate classification models for predicting TDG synthesis methods could be built, but their prediction performance was slightly lower than the corresponding M3a–ccrude models. Spiked matrix test sets representing R7, R8, and R9 were easiest to predict, and the number of correct predictions in spiked soil samples was equal to that in textile samples, indicating that the classification models handled both matrices equally well.
Table 3
OPLS-DA | RF | ||||||||||
---|---|---|---|---|---|---|---|---|---|---|---|
classification model | attribution capacity | class | comp.a | R2X (cum) | R2Y (cum) | Q2(cum) | pred. soil | pred. textile | OOB error (%) | pred. soil | pred. textile |
M1matrix | ethylene or TDG routes | R(10, 11) | 1 + 2 + 0 | 0.45 | 0.99 | 0.97 | - | - | 0 | - | - |
R(1–9) | |||||||||||
M2matrix | chlorination methods | R(1, 4, 7) | 2 + 3 + 0 | 0.46 | 0.97 | 0.8 | 5/6 | 4/4b | 0 | 5/6 | 4/4b |
R(2, 5, 8) | 5/6 | 5/5b | 5/6 | 5/5b | |||||||
R(3, 6, 9) | 2/6 | 2/6 | 2/6 | 2/6 | |||||||
M3amatrix | TDG synthesis methods of R(1, 4, 7) samples | R1 | 2 + 2 + 0 | 0.75 | 0.93 | 0.66 | 2/2 | 0/1 | 2.9 | 2/2 | 1/1 |
R4 | 0/2 | 0/1 | 0/2 | 0/1 | |||||||
R7 | 2/2 | 2/2 | 2/2 | 2/2 | |||||||
M3bmatrix | TDG synthesis methods of R(2, 5, 8) samples | R2 | 2 + 3 + 0 | 0.80 | 0.97 | 0.91 | 2/2 | 1/1 | 2.8 | 0/2 | 0/1 |
R5 | 2/2 | 2/2 | 2/2 | 2/2 | |||||||
R8 | 2/2 | 2/2 | 2/2 | 2/2 | |||||||
M3cmatrix | TDG synthesis methods of R(3, 6, 9) samples | R3 | 2 + 0 + 0 | 0.73 | 0.64 | 0.26 | 0/2 | 0/2 | 0 | 0/2 | 0/2 |
R6 | 2/2 | 2/2 | 2/2 | 2/2 | |||||||
R9 | 2/2 | 0/2 | 2/2 | 2/2 |
Comparison of Classification Tools
The parallel use of OPLS-DA and RF in this study allowed us to benchmark the performance of the two classification tools. When the multimodel hierarchical decision tree (Figure Figure22) was used, the two methods achieved very similar predictive accuracy (Table 2). Many of the CAS important for separation by OPLS-DA and RF were identical, especially in M2crude (Table S2). However, since RF is a tree-based algorithm, it does not require the use of such a decision tree; the tree was only used with RF models to permit detailed performance comparison with OPLS-DA. RF could also be used to resolve all of the classes in a single model; accordingly, 11-class models were constructed for both crude samples (M4crude) and matrix samples (M4matrix). The M4crude model outperformed the OPLS-DA decision tree models for crude samples, with 10/11 correct classifications (Tables 2 and 4).
Table 4
test set
samples | ||||
---|---|---|---|---|
model | OOB error (%) | crude HD | spiked soil | spiked textile |
M4crude | 20.5 | 10/11a | - | - |
M4matrix | 0.8 | - | 8/18a | 10/15a |
Conversely, the 11-class RF model for spiked matrix samples (M4matrix) performed less well than the hierarchical decision tree models generated using both RF and OPLS-DA. In the test set validation of model M4matrix (Table 4), 18 samples out of 33 were classified correctly.
While the performance differences between the classification methods were small, they differed in their handling of misclassifications: OPLS-DA models assign samples that cannot be classified as not belonging to any class (no class-category in Table 5), whereas RF models force samples into one class. This property was shown when relevant CAS could not be detected in matrix samples spiked with low levels of HD and the samples were incorrectly assigned to the HCl chlorination routes by RF (Table 5). The CAS profile associated with HCl chlorination (R1, 4, 7) is sparse, making it sensible to incorrect classifications of samples with low CAS levels. This RF misclassification problem also affected the 11-class models.
Table 5
predicted
class | |||||||
---|---|---|---|---|---|---|---|
OPLS-DA | RF | ||||||
true class | R1, 4, 7 | R2, 5, 8 | R3, 6, 9 | no class | R1, 4, 7 | R2, 5, 8 | R3, 6, 9 |
R1, 4, 7 | 9a | 1 | 9a | 1 | |||
R2, 5, 8 | 1 | 7a | 3 | 1 | 10a | ||
R3, 6, 9 | 1 | 4a | 7 | 8 | 4a |
Future Prospects
The data presented here would preferably be used to develop a robust and efficient targeted method using the CAS database. The nontargeted approach is not readily applicable to authentic samples because it requires reference data acquired under identical instrumental conditions (e.g., analyzed in the same sample batch). Such data would be difficult to acquire because the composition of the HD reference samples is not necessarily stable over time. A CAS HRMS library would enable easy processing of new samples and could also be shared between laboratories. CAS for inclusion in such a database could be selected based on their relevance for route classification. Some (48/714) of the CAS from crude HD and spiked matrix samples were found in all three datasets, but many variables were matrix-specific (Figure Figure44). This difficulty could be overcome by using separate target libraries for crude and spiked matrix samples.
Bayesian statistics is a widely accepted forensic statistic framework33 used in order to support court decisions. It could be applied to the classification models presented above, in which estimated assignment probabilities are used, together with prior information, to calculate likelihood ratios of competing propositions. The outcome (i.e., the likelihood ratio) can then be communicated to court hearings in a transparent and scientific sound way. The methods for such calculations based on multivariate data are currently under development.14,34
Conclusions
We successfully developed a nontargeted method for attribution of crude HD and spiked matrix samples to specific synthesis routes using HRMS data. The nontargeted approach enabled efficient processing of large numbers of CAS, and the high recovery of CAS facilitated the generation of significant HD route classification models using two independent classification methods, OPLS-DA and RF. The two classification methods achieved similar classification accuracy. Classification performance was very good for crude HD samples but somewhat lower for spiked matrix samples due to matrix effects. Route determination of the spiked matrices samples was made difficult due to the low spiking level. Real-world samples involving HD, may have higher concentrations, making the classification of routes easier. Overall, our results show that nontargeted methods can be valuable tools for CAS screening in chemical forensics, and that a CAS library could be a powerful tool in future investigations into alleged uses of CWA.
Acknowledgments
The authors thank Johan Dahlén, Linköpings University, Sweden, for support and valuable discussions. This work was funded by the Swedish Ministry of Defence and the Swedish Civil Contingencies Agency.
Supporting Information Available
The Supporting Information is available free of charge at https://pubs.acs.org/doi/10.1021/acs.analchem.0c04555.
(Table S1) Most important variables in M1crude models; (Table S2) most important variables in M2crude models; (Table S3) most important variables in M3crude models; (Table S4) most important variables in M2matrix models; (Figure S1) HD synthesis descriptions and representative CAS; (Figure S2) total ion chromatogram of blank matrix samples and spiked matrix samples (PDF)
Author Contributions
The manuscript was written through contributions of all authors. All authors have given approval to the final version of the manuscript.
Notes
The authors declare no competing financial interest.
References
- Vanninen P.; Lignell H.; Heikkinen H.; Kiljunen H.; Silva O.; Aalto S.; Kauppila T.. Chemical Forensics in 21st Century Prometheus, Martellini M.; Trapp R., Eds., Springer, Cham., 2020, https://doi.org/10.1007/978-3-030-28285-1_12.
- Hoggard J. C.; Wahl J. H.; Synovec R. E.; Mong G. M.; Fraga C. G. Impurity Profiling of a Chemical Weapon Precursor for Possible Forensic Signatures by Comprehensive Two-Dimensional Gas Chromatography/Mass Spectrometry and Chemometrics. Anal. Chem. 2010, 82, 689–698. 10.1021/ac902247x. [Abstract] [CrossRef] [Google Scholar]
- Fraga C. G.; Pérez Acosta G. A.; Crenshaw M. D.; Wallace K.; Mong G. M.; Colburn H. A. Impurity Profiling to Match a Nerve Agent to Its Precursor Source for Chemical Forensics Applications. Anal. Chem. 2011, 83, 9564–9572. 10.1021/ac202340u. [Abstract] [CrossRef] [Google Scholar]
- Fraga C. G.; Bronk K.; Dockendorff B. P.; Heredia-Langner A. Organic Chemical Attribution Signatures for the Sourcing of a Mustard Agent and Its Starting Materials. Anal. Chem. 2016, 88, 5406–5413. 10.1021/acs.analchem.6b00766. [Abstract] [CrossRef] [Google Scholar]
- Fraga C. G.; Sego L. H.; Hoggard J. C.; Acosta G. A. P.; Viglino E. A.; Wahl J. H.; Synovec R. E. Preliminary effects of real-world factors on the recovery and exploitation of forensic impurity profiles of a nerve-agent simulant from office media. J Chromatogr. A 2012, 1270, 269–282. 10.1016/j.chroma.2012.10.053. [Abstract] [CrossRef] [Google Scholar]
- Strozier E. D.; Mooney D. D.; Friedenberg D. A.; Klupinski T. P.; Triplett C. A. Use of Comprehensive Two-Dimensional Gas Chromatography with Time-of-Flight Mass Spectrometric Detection and Random Forest Pattern Recognition Techniques for Classifying Chemical Threat Agents and Detecting Chemical Attribution Signatures. Anal. Chem. 2016, 88, 7068–7075. 10.1021/acs.analchem.6b00725. [Abstract] [CrossRef] [Google Scholar]
- Colburn H. A.; Wunschel D. S.; Kreuzer H. W.; Moran J. J.; Antolick K. C.; Melville A. M. Analysis of Carbohydrate and Fatty Acid Marker Abundance in Ricin Toxin Preparations for Forensic Information. Anal. Chem. 2010, 82, 6040–6047. 10.1021/ac1006206. [Abstract] [CrossRef] [Google Scholar]
- Behringer D. L.; Smith D. L.; Katona V. R.; Lewis A. T. Jr.; Hernon-Kenny L. A.; Crenshaw M. D. Demonstration of spread-on peel-off consumer products for sampling surfaces contaminated with pesticides and chemical warfare agent signatures. Forensic Sci. Int. 2014, 241, 7–14. 10.1016/j.forsciint.2014.04.030. [Abstract] [CrossRef] [Google Scholar]
- Fraga C. G.; Clowers B. H.; Moore R. J.; Zink E. M. Signature-Discovery Approach for Sample Matching of a Nerve-Agent Precursor Using Liquid Chromatography-Mass Spectrometry, XCMS, and Chemometrics. Anal. Chem. 2010, 82, 4165–4173. 10.1021/ac1003568. [Abstract] [CrossRef] [Google Scholar]
- OPCW , Decision: Addressing the Threat from Chemical Weapons Use; OPCW; 2018; pp C-SS-4/DEC.3. [Google Scholar]
- OPCW , Investigative Science and Technology Report of the scientific advisory board′s temporary working group; OPCW; 2019; Vol. SAB/REP/1/19. [Google Scholar]
- Andersson K.; Lock E.; Jalava K.; Huizer H.; Jonson S.; Kaa E.; Lopes A.; Poortman-Van Der Meer A.; Sippola E.; Dujourdy L.; Dahlén J. Development of a harmonised method for the profiling of amphetamines - VI - Evaluation of methods for comparison of amphetamine. Forensic Sci. Int. 2007, 169, 86–99. 10.1016/j.forsciint.2006.10.020. [Abstract] [CrossRef] [Google Scholar]
- Morelato M.; Beavis A.; Tahtouh M.; Ribaux O.; Kirkbride P.; Roux C. The use of forensic case data in intelligence-led policing: The example of drug profiling. Forensic Sci. Int. 2013, 226, 1–9. 10.1016/j.forsciint.2013.01.003. [Abstract] [CrossRef] [Google Scholar]
- Lopatka M.; Sigman M. E.; Sjerps M. J.; Williams M. R.; Vivó-Truyols G. Class-conditional feature modeling for ignitable liquid classification with substantial substrate contribution in fire debris analysis. Forensic Sci. Int. 2015, 252, 177–186. 10.1016/j.forsciint.2015.04.035. [Abstract] [CrossRef] [Google Scholar]
- Mazzitelli C. L.; Re M. A.; Reaves M. A.; Acevedo C. A.; Straight S. D.; Chipuk J. E. A Systematic Method for the Targeted Discovery of Chemical Attribution Signatures: Application to Isopropyl Bicyclophosphate Production. Anal. Chem. 2012, 84, 6661–6671. 10.1021/ac300859j. [Abstract] [CrossRef] [Google Scholar]
- Mörén L.; Qvarnström J.; Engqvist M.; Afshin-Sander R.; Wu X.; Dahlén J.; Löfberg C.; Larsson A.; Östin A. Attribution of fentanyl analogue synthesis routes by multivariate data analysis of orthogonal mass spectral data. Talanta 2019, 203, 122–130. 10.1016/j.talanta.2019.05.025. [Abstract] [CrossRef] [Google Scholar]
- Mirjankar N. S.; Fraga C. G.; Carman A. J.; Moran J. J. Source Attribution of Cyanides Using Anionic Impurity Profiling, Stable Isotope Ratios, Trace Elemental Analysis and Chemometrics. Anal. Chem. 2016, 88, 1827–1834. 10.1021/acs.analchem.5b04126. [Abstract] [CrossRef] [Google Scholar]
- Sacco A.; Brescia M. A.; Sgaramella A.; Sacco D. Characterization of the composition and the geographic origin of food products by means of nuclear magnetic resonance and isotope ratio mass spectroscopy. Recent Res. Dev. Agric. Food Chem. 2005, 6, 119–142. [Google Scholar]
- Ghabili K.; Agutter P. S.; Ghanei M.; Ansarin K.; Panahi Y.; Shoja M. M. Sulfur mustard toxicity: History, chemistry, pharmacokinetics, and pharmacodynamics. Crit. Rev. Toxicol. 2011, 41, 384–403. 10.3109/10408444.2010.541224. [Abstract] [CrossRef] [Google Scholar]
- Koblentz G. Chemical-weapon use in Syria: atrocities, attribution, and accountability. Nonproliferation Rev. 2019, 26, 575–598. 10.1080/10736700.2019.1718336. [CrossRef] [Google Scholar]
- Holmgren K. H.; Hok S.; Magnusson R.; Larsson A.; Åstot C.; Koester C.; Mew D.; Vu A. K.; Alcaraz A.; Williams A. M.; Norlin R.; Wiktelius D. Synthesis route attribution of sulfur mustard by multivariate data analysis of chemical signatures. Talanta 2018, 186, 615–621. 10.1016/j.talanta.2018.02.100. [Abstract] [CrossRef] [Google Scholar]
- Veenaas C.; Haglund P. Methodology for non-target screening of sewage sludge using comprehensive two-dimensional gas chromatography coupled to high-resolution mass spectrometry. Anal. Bioanal. Chem. 2017, 409, 4867–4883. 10.1007/s00216-017-0429-0. [Europe PMC free article] [Abstract] [CrossRef] [Google Scholar]
- Soulier C.; Coureau C.; Togola A. Environmental forensics in groundwater coupling passive sampling and high resolution mass spectrometry for screening. Sci. Total Environ. 2016, 563-564, 845–854. 10.1016/j.scitotenv.2016.01.056. [Abstract] [CrossRef] [Google Scholar]
- Gómez-Ramos M. M.; Ucles S.; Ferrer C.; Fernández-Alba A. R.; Hernando M. D. Exploration of environmental contaminants in honeybees using GC-TOF-MS and GC-Orbitrap-MS. Sci. Total Environ. 2019, 647, 232–244. 10.1016/j.scitotenv.2018.08.009. [Abstract] [CrossRef] [Google Scholar]
- Schymanski E. L.; Jeon J.; Gulde R.; Fenner K.; Ruff M.; Singer H. P.; Hollender J. Identifying Small Molecules via High Resolution Mass Spectrometry: Communicating Confidence. Environ. Sci. Technol. 2014, 48, 2097–2098. 10.1021/es5002105. [Abstract] [CrossRef] [Google Scholar]
- Vanninen P.RECOMMENDED OPERATING PROCEDURES FOR ANALYSIS IN THE VERIFICATION OF CHEMICAL DISARMAMENT. 2017 ed.; VERIFIN, Department of Chemistry, University of Helsinki; Finland: ISBN 978–951–51-3917-7, 2017. [Google Scholar]
- OPCW , Seventh report of the Organisation for the Prohibition of Chemical Weapons-United Nations Joint Investigative Mechanism United Nations Security Council Letters; OPCW; 2017; p S/2017/904. [Google Scholar]
- Jansson D.; Lindström S. W.; Norlin R.; Hok S.; Valdez C. A.; Williams A. M.; Alcaraz A.; Nilsson C.; Åstot C. Part 2: Forensic attribution profiling of Russian VX in food using liquid chromatography-mass spectrometry. Talanta 2018, 186, 597–606. 10.1016/j.talanta.2018.02.103. [Abstract] [CrossRef] [Google Scholar]
- Williams A. M.; Vu A. K.; Mayer B. P.; Hok S.; Valdez C. A.; Alcaraz A. Part 3: Solid phase extraction of Russian VX and its chemical attribution signatures in food matrices and their detection by GC-MS and LC-MS. Talanta 2018, 186, 607–614. 10.1016/j.talanta.2018.03.044. [Abstract] [CrossRef] [Google Scholar]
- Favela K. H.; Bohmann J. A.; Williamson W. S. Dust as a collection media for contaminant source attribution. Forensic Sci. Int. 2012, 217, 39–49. 10.1016/j.forsciint.2011.09.024. [Abstract] [CrossRef] [Google Scholar]
- D’Agostino P. A.; Hancock J. R.; Chenier C. L.; Lepage C. R. J. Liquid chromatography electrospray tandem mass spectrometric and desorption electrospray ionization tandem mass spectrometric analysis of chemical warfare agents in office media typically collected during a forensic investigation. J. Chromatogr. A 2006, 1110, 86–94. 10.1016/j.chroma.2006.01.083. [Abstract] [CrossRef] [Google Scholar]
- Wahl J. H.; Colburn H. A. Extraction of chemical impurities for forensic investigations: A case study for indoor releases of a sarin surrogate. Build. Sci. 2010, 45, 1339–1345. 10.1016/j.buildenv.2009.10.020. [CrossRef] [Google Scholar]
- Aiken CGG., Taroni T.. Statistics and the Evaluation of Evidence for Forensic Scientist (2nd edn); Wiley: Chichester, 2004. [Google Scholar]
- Ahlinder J.; Nordgaard A.; Lindström S. W. Chemometrics comes to court: evidence evaluation of chem-bio threat agent attacks. J. Chemom. 2015, 29, 267–276. 10.1002/cem.2699. [CrossRef] [Google Scholar]
- Cuyckens F.; Hurkmans R.; Castro-Perez J. M.; Leclercq L.; Mortishire-Smith R. J. Extracting metabolite ions out of a matrix background by combined mass defect, neutral loss and isotope filtration. Rapid Commun. Mass Spectrom. 2009, 23, 327–332. 10.1002/rcm.3881. [Abstract] [CrossRef] [Google Scholar]
- Bylesjö M.; Rantalainen M.; Cloarec O.; Nicholson J. K.; Holmes E.; Trygg J. OPLS discriminant analysis: combining the strengths of PLS-DA and SIMCA classification. J. Chemom. 2006, 20, 341–351. 10.1002/cem.1006. [CrossRef] [Google Scholar]
- Breiman L. Random forests. Machine Learning 2001, 45, 5–32. 10.1023/A:1010933404324. [CrossRef] [Google Scholar]
- R Core Team . R: A language and environment for statistical computing; R Foundation for Statistical. Computing: Vienna, Austria. 2020. http://www.R-project.org/. [Google Scholar]
- Liaw A.; Wiener M. Classification and Regression by random Forest. R News 2002, 2, 18–22. [Google Scholar]
- User Guide SIMCA® 15, Guide edition date: November 17, 2017. https://blog.umetrics.com/hubfs/Download%20Files/drupal/simca_15_user_guide.pdf (accessed Jan 11, 2021).
- Strobl C.; Boulesteix A.-L.; Zeileis A.; Hothorn T. Bias in random forest variable importance measures: Illustrations, sources and a solution. BMC Bioinfo. 2007, 8, 1.10.1186/1471-2105-8-25. [Europe PMC free article] [Abstract] [CrossRef] [Google Scholar]
Full text links
Read article at publisher's site: https://doi.org/10.1021/acs.analchem.0c04555
Read article for free, from open access legal sources, via Unpaywall:
http://liu.diva-portal.org/smash/get/diva2:1707979/FULLTEXT01
Citations & impact
This article has not been cited yet.
Impact metrics
Alternative metrics

Discover the attention surrounding your research
https://www.altmetric.com/details/102435221
Data
Data behind the article
This data has been text mined from the article, or deposited into data resources.
BioStudies: supplemental material and supporting data
Similar Articles
To arrive at the top five similar articles we use a word-weighted algorithm to compare words from the Title and Abstract of each citation.
Synthesis route attribution of sulfur mustard by multivariate data analysis of chemical signatures.
Talanta, 186:615-621, 01 Mar 2018
Cited by: 4 articles | PMID: 29784411
Chemometrics-assisted analysis of chemical impurity profiles of tabun nerve agent using comprehensive two-dimensional gas chromatography-time-of-flight mass spectrometry.
J Chromatogr A, 1685:463643, 13 Nov 2022
Cited by: 1 article | PMID: 36401911
Determination of the sulfur mustard hydrolysis product thiodiglycol by microcolumn liquid chromatography coupled on-line with sulfur flame photometric detection using large-volume injections and peak compression.
J Chromatogr A, 849(2):433-444, 01 Jul 1999
Cited by: 7 articles | PMID: 10457441
Funding
Funders who supported this work.